Tumor growth modeling and its applications in preclinical pharmacology studies to improve translatability of animal models
Cancer Research(2022)
摘要
Abstract Successful oncology drug development hinges on proper use of preclinical xenograft and allograft mouse models that faithfully reflect histopathology and genomic features of patient tumors and exhibit clinically similar drug response. For in vivo pharmacology studies, tumor-bearing mice are treated by drugs and efficacy is monitored by continuingly measuring tumor volumes over weeks or even months, resulting in mouse-specific tumor growth data. In our previous study, we used linear mixed model (LMM) to fit tumor growth data and demonstrated its superiority to simple readouts, such as tumor growth inhibition (TGI), in discovering biomarkers and exploring mechanism of action for drugs. Here we extended the study to more parametric statistical models on an expanded dataset with over 100 thousand tumor growth curves. Six parametric models (exponential, exponential square, Gompertz, Logistic, Monomolecular, and von Bertalanffy) were fit to tumor growth curves within a single-treatment group, by the least square method after variance stabilization transformation. Best models were selected by Akaike information criterion (AIC)-based confidence set. In the Irinotecan case study, logarithmic transformation of tumor volume converts exponential or exponential square models to mixed-effects linear models that integrate random effect of mouse difference within a treatment group, and are used to identify predictive biomarkers for drug efficacy. We compared three variance stabilization transformations and found that log transformation of tumor volume is appropriate for majority of tumor growth curves. In log transformed data, exponential or exponential square model can satisfactorily fit nearly all tumor growth curves, as 96.1% of best model confidence sets contain exponential or exponential square model, followed by von Bertalanffy (92.5%), Gompertz (92.1%), Logistic (90.5%), and Monomolecular (58.9%). The percentage of studies, in which all treatment groups can be best fit by exponential or exponential square model, is 91.3% (82.4% for von Bertalanffy, 81.4% for Gompertz, 79.1% for Logistic, 37.3% for Monomolecular). Thus, exponential or exponential square model can be applied to most in vivo mouse studies, and should be preferred for their mathematical simplicity and interpretability. We further demonstrated the utility of the modeling method on a 16-PDX-model study for Irinotecan to identify biological processes and pathways related to its mechanism of action. Citation Format: Huajun Zhou, Binchen Mao, Sheng Guo. Tumor growth modeling and its applications in preclinical pharmacology studies to improve translatability of animal models [abstract]. In: Proceedings of the American Association for Cancer Research Annual Meeting 2022; 2022 Apr 8-13. Philadelphia (PA): AACR; Cancer Res 2022;82(12_Suppl):Abstract nr 2743.
更多查看译文
关键词
tumor growth modeling,tumor growth,animal models,preclinical pharmacology studies
AI 理解论文
溯源树
样例
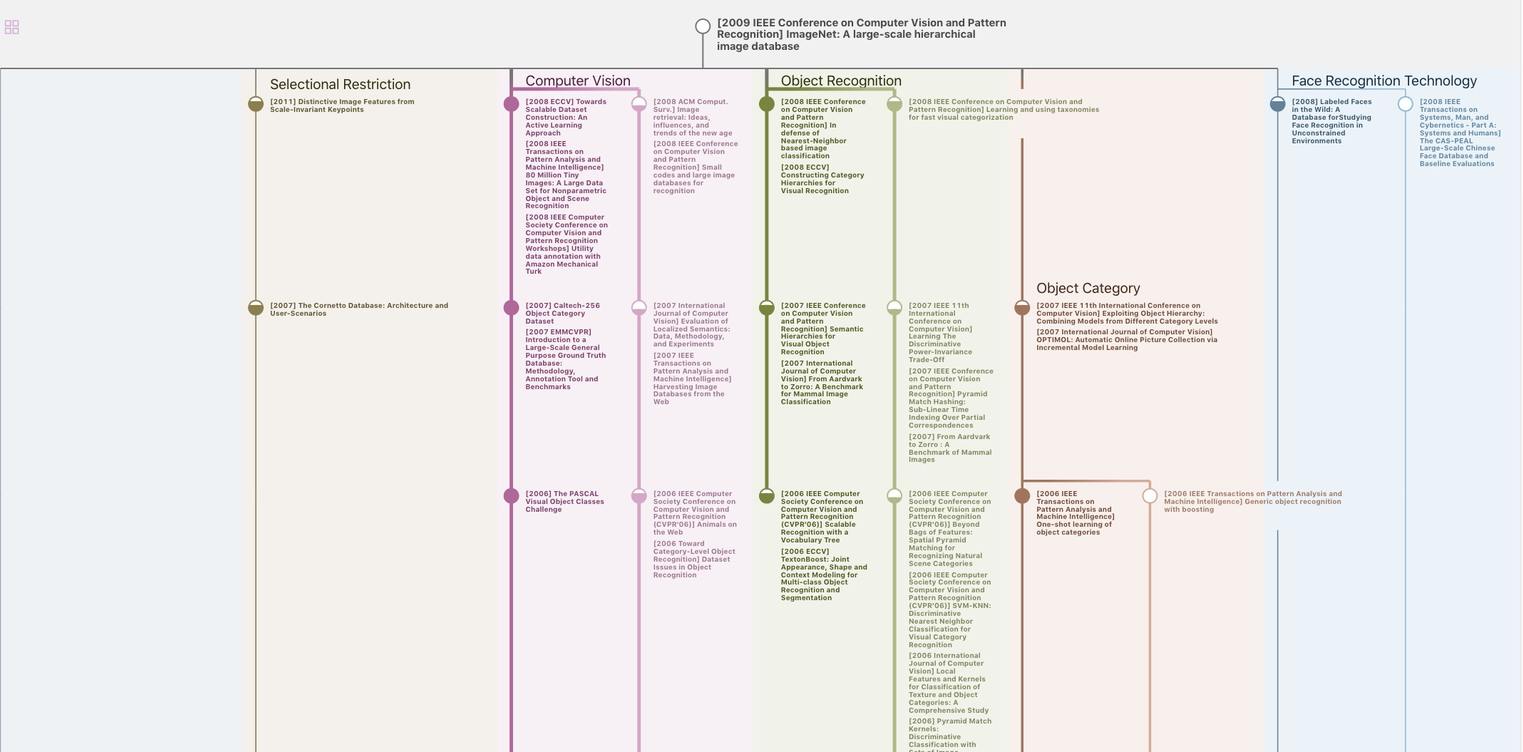
生成溯源树,研究论文发展脉络
Chat Paper
正在生成论文摘要