Abstract 2733: A computational model of cellular metabolism with mutation data predicts metabolites associated with somatic mutations in cancers
Cancer Research(2022)
摘要
Abstract Metabolic reprogramming is considered a hallmark of cancers, which plays an important role in cancer progression and development, partly as a consequence of somatic mutations. A representative product of metabolic reprogramming in cancers is oncometabolites that show abnormal accumulation in a cancer cell, induce malignancy, and are generated upon mutations in a metabolic gene, often IDH1, SDH or FH. Identification of novel mutation-associated metabolites will facilitate developing biomarkers and treatment strategies for cancers, as in the case of ivosidenib, an FDA approved drug for treating acute myeloid leukemia (AML) having the IDH1 mutant. To this end, we develop a computational workflow that predicts so-called metabolite-gene-pathway sets (MGPs) that present metabolites and metabolic pathways significantly associated with gene mutations in cancers. The computational workflow uses cancer patients’ mutation data and a computational model of cellular metabolism called a genome-scale metabolic models (GEM). In this study, the computational workflow was demonstrated using 943 cancer patient-specific GEMs representing 24 different cancer types based on the Pan-Cancer Analysis of Whole Genomes (PCAWG) data [1], which, as a result, predicted 4,135 MGPs for these multiple cancer types. The computational workflow was shown to generate biologically meaningful MGPs on the basis of multi-omics data from 17 AML samples collected in this study as well as previous published studies. For the AML samples, metabolites of the MGPs predicted using the computational workflow showed significantly different intracellular concentrations, depending on mutation of genes involved in the MGP. Moreover, for the 115 MGPs predicted for CNS-GBM/Oligo that are associated with mutation of CIC, EGFR, IDH1 or TP53, 69% of the MGPs were supported by previous studies. This two-level validation indeed showed that it is possible to characterize metabolic pathways and their metabolites that are affected in response to somatic mutations in a cancer cell. Our computational workflow and its prediction outcomes will help better understand the mutation-associated metabolic reprogramming in cancers, and serve as a valuable resource for further extended studies on cancer metabolism. [1] The ICGC/TCGA Pan-Cancer Analysis of Whole Genomes Consortium. Pan-cancer analysis of whole genomes. Nature 578, 82-93 Citation Format: Sang Mi Lee, GaRyoung Lee, Sungyoung Lee, Hyojin Song, Sung Soo Yoon, Hongseok Yun, Youngil Koh, Hyun Uk Kim. A computational model of cellular metabolism with mutation data predicts metabolites associated with somatic mutations in cancers [abstract]. In: Proceedings of the American Association for Cancer Research Annual Meeting 2022; 2022 Apr 8-13. Philadelphia (PA): AACR; Cancer Res 2022;82(12_Suppl):Abstract nr 2733.
更多查看译文
关键词
cellular metabolism,somatic mutations,mutation data
AI 理解论文
溯源树
样例
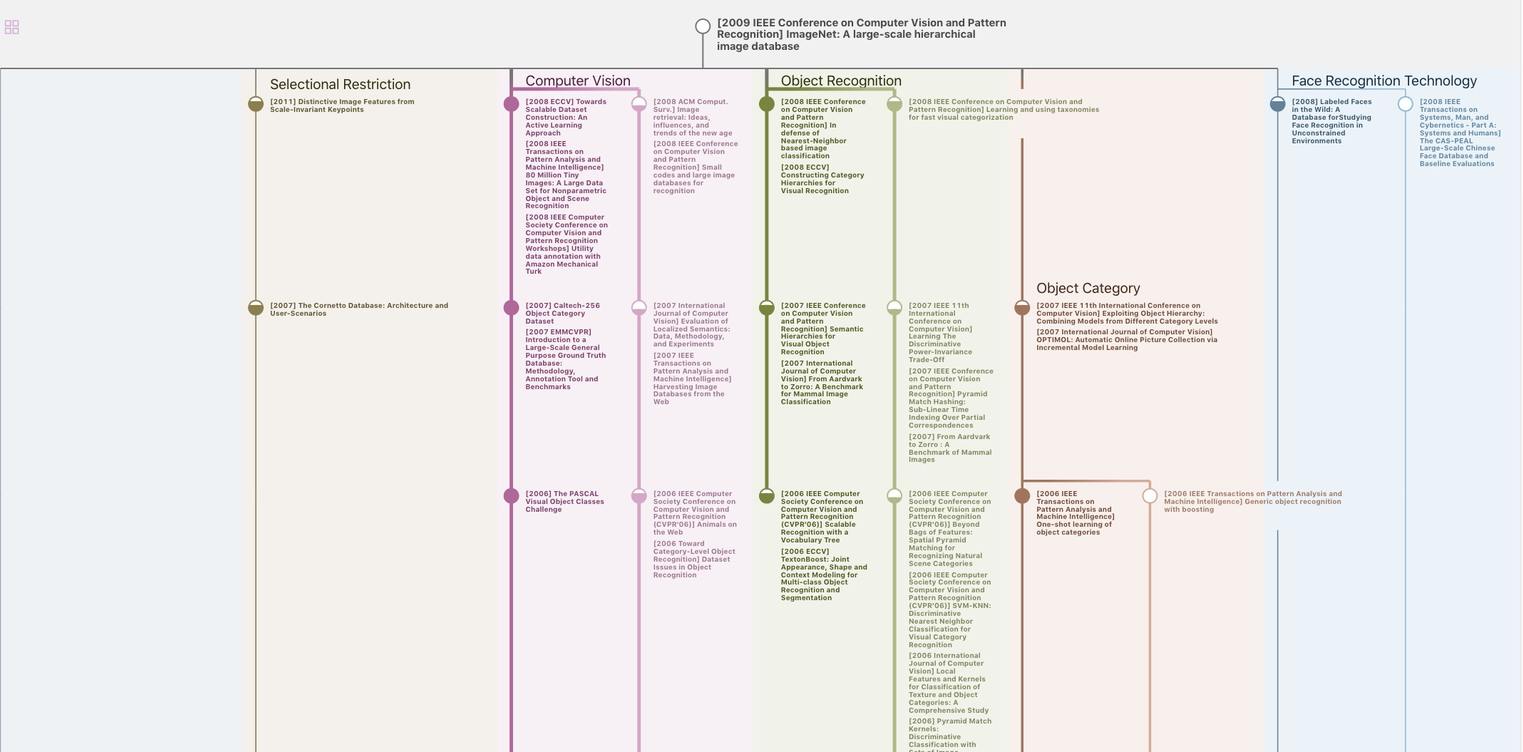
生成溯源树,研究论文发展脉络
Chat Paper
正在生成论文摘要