A resampling-based empirical Bayes method for precise false discovery rate estimation under dependence
crossref(2022)
摘要
Abstract Many methods exist for controlling or estimating the false discovery rate (FDR), which is the mean of the false discovery proportion (FDP). However, in the presence of correlation between the test statistics, the FDP may show considerable variation between repeated experiments. Here we first provide an intuitive explanation for this phenomenon by demonstrating an increased sampling variability of the test statistics when tests are dependent. Next, we propose an empirical Bayes solution based on resampling techniques that is applicable to many types of test statistics, and allows to estimate the null distribution more precisely. This reduces the variability of the FDP and even yields an increase in sensitivity. Furthermore the implicit assumptions made by empirical Bayes approaches to false discovery rate estimation are explored. We demonstrate our approach on the differential expression problem in RNA-sequencing, and on a data integration problem of microbiome sequence count and flow cytometry data, where the test statistics are correlated by design. Our algorithm is available as the R-package reconsi.
更多查看译文
AI 理解论文
溯源树
样例
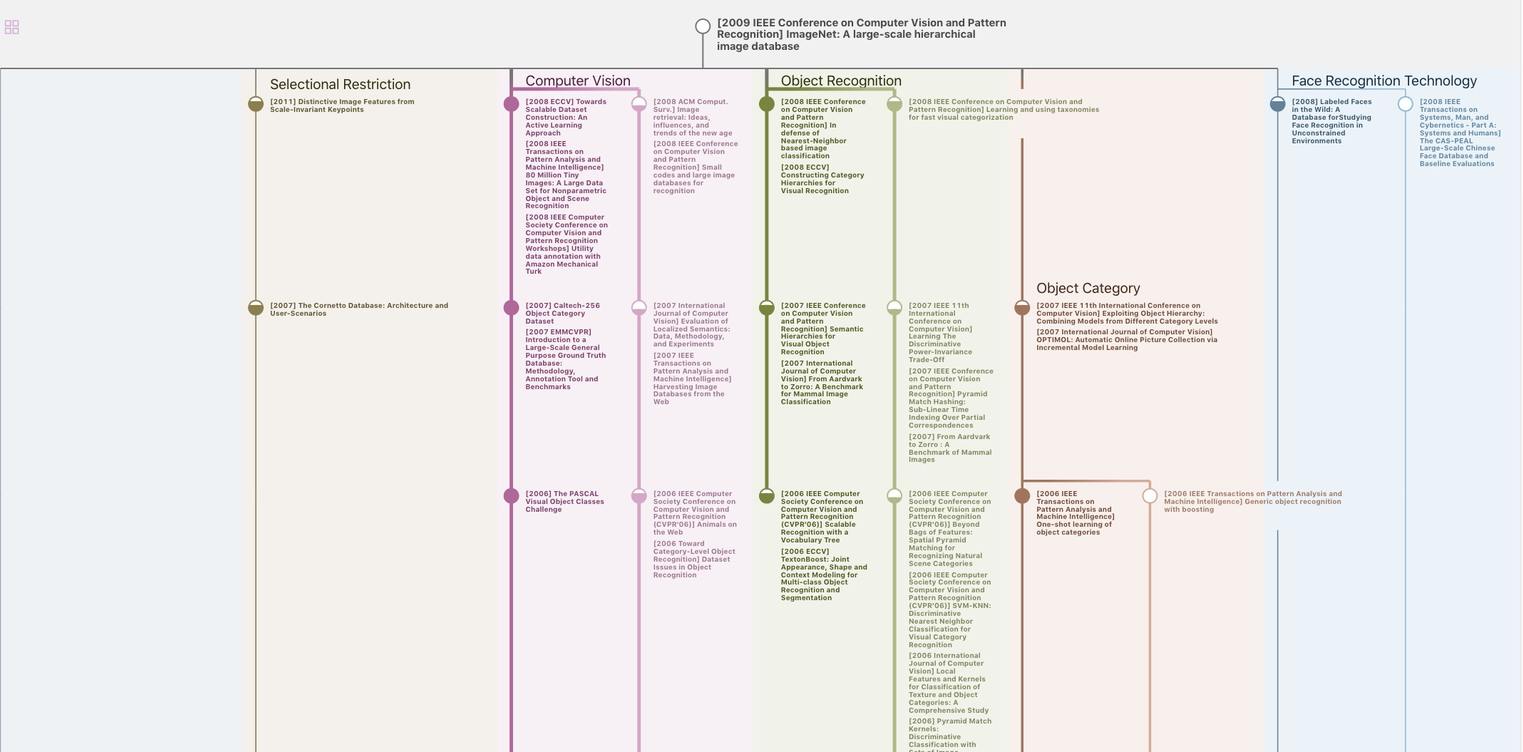
生成溯源树,研究论文发展脉络
Chat Paper
正在生成论文摘要