Data-Driven Global Subseasonal Forecast for Intraseasonal Oscillation Components
ATMOSPHERE(2023)
摘要
As a challenge in the construction of a "seamless forecast" system, improving the prediction skills of subseasonal forecasts is a key issue for meteorologists. In view of the evolution characteristics of numerical models and deep-learning models for subseasonal forecasts, as forecast times increase, the prediction skill for high-frequency components will decrease, as the lead time is already far beyond the predictability. Meanwhile, intraseasonal low-frequency components are essential to the change in general circulation on subseasonal timescales. In this paper, the Global Subseasonal Forecast Model (GSFM v1.0) first extracted the intraseasonal oscillation (ISO) components of atmospheric signals and used an improved deep-learning model (SE-ResNet) to train and predict the ISO components of geopotential height at 500 hPa (Z500) and temperature at 850 hPa (T850). The results show that the 10-30 day prediction performance of the SE-ResNet model is better than that of the model trained directly with original data. Compared with other models/methods, this model has a good ability to depict the subseasonal evolution of the ISO components of Z500 and T850. In particular, although the prediction results from the Climate Forecast System Version 2 have better performance through 10 days, the SE-ResNet model is substantially superior to CFSv2 through 10-30 days, especially in the middle and high latitudes. The SE-ResNet model also has a better effect in predicting planetary waves with wavenumbers of 3-8. Thus, the application of data-driven subseasonal forecasts of atmospheric ISO components may shed light on improving the skill of seasonal forecasts.
更多查看译文
关键词
neural network,machine learning,extended-range forecast,intraseasonal component
AI 理解论文
溯源树
样例
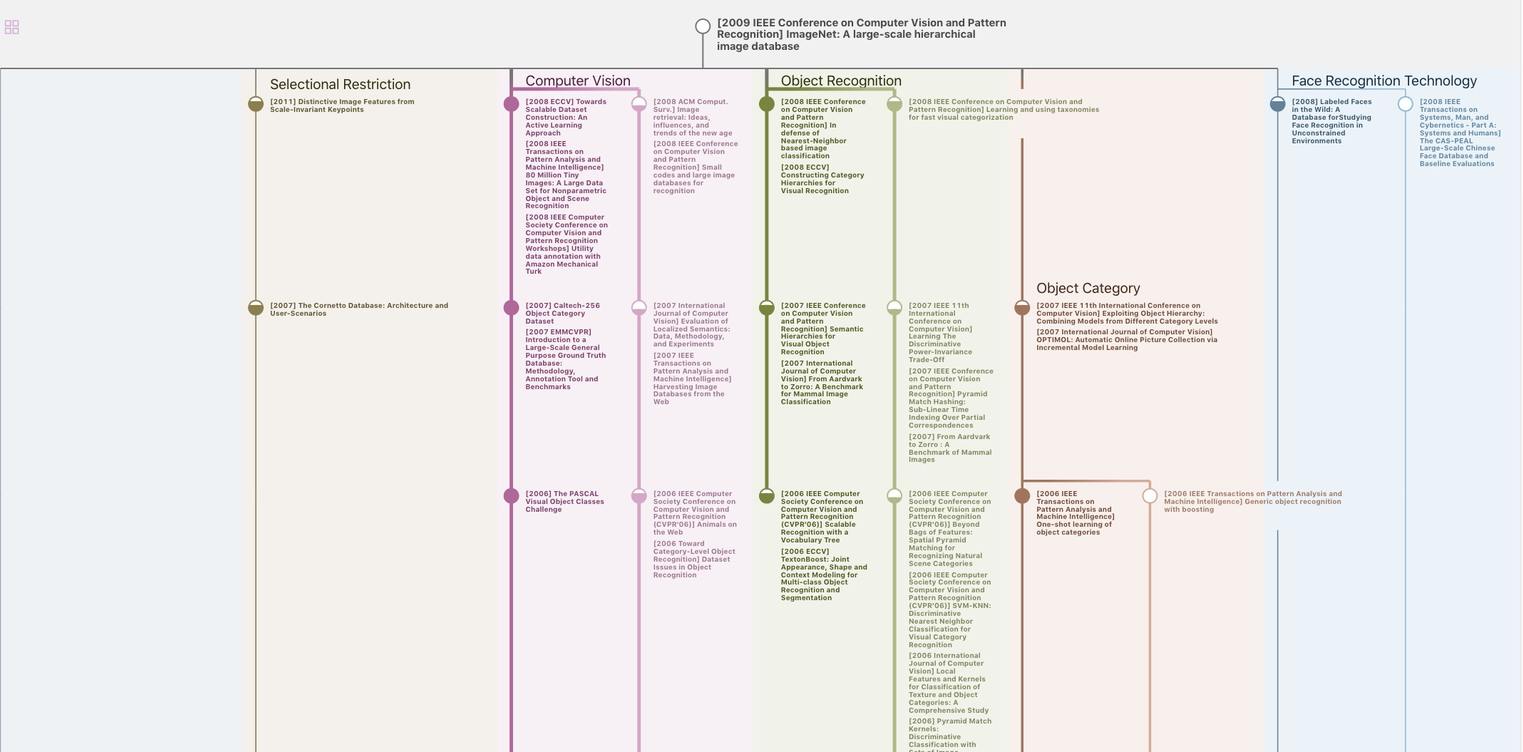
生成溯源树,研究论文发展脉络
Chat Paper
正在生成论文摘要