Reflective Learning With Label Noise
IEEE Transactions on Circuits and Systems for Video Technology(2023)
摘要
Learning with noisy labels is one of the most challenging tasks in semi-supervised learning, and it poses significant problems in various practical applications. In the network learning process, the noisy labels concealed in the training dataset are easy to remember, resulting in poor generalization performance. To overcome this problem, inspired by the correction ability of humans - "think and learn from the past," an end-to-end dynamic correction framework against label noise called Reflective Learning (RL) is proposed. This solution incorporates valuable knowledge from the past network training process to assist in correcting noisy labels. Specifically, during network training, a dynamic iterative function is implemented to adaptively correct noisy labels by employing the network's predictive distribution information of all training epochs. This dynamic iterative function takes the form of a Standard Normal Distribution function to effectively match the changes of noisy label correction information contained in the network's predictive probabilities. The proposed method is general and applicable to any backbone network and different types of noise without auxiliary information. Experiments are conducted on datasets with synthetic and real-world label noise datasets, including CIFAR-10, CIFAR-100, Tiny-ImageNet, and Clothing1M. They demonstrate that the proposed method is superior to the state-of-the-art results.
更多查看译文
关键词
Reflective learning,noisy labels,dynamic iterative function
AI 理解论文
溯源树
样例
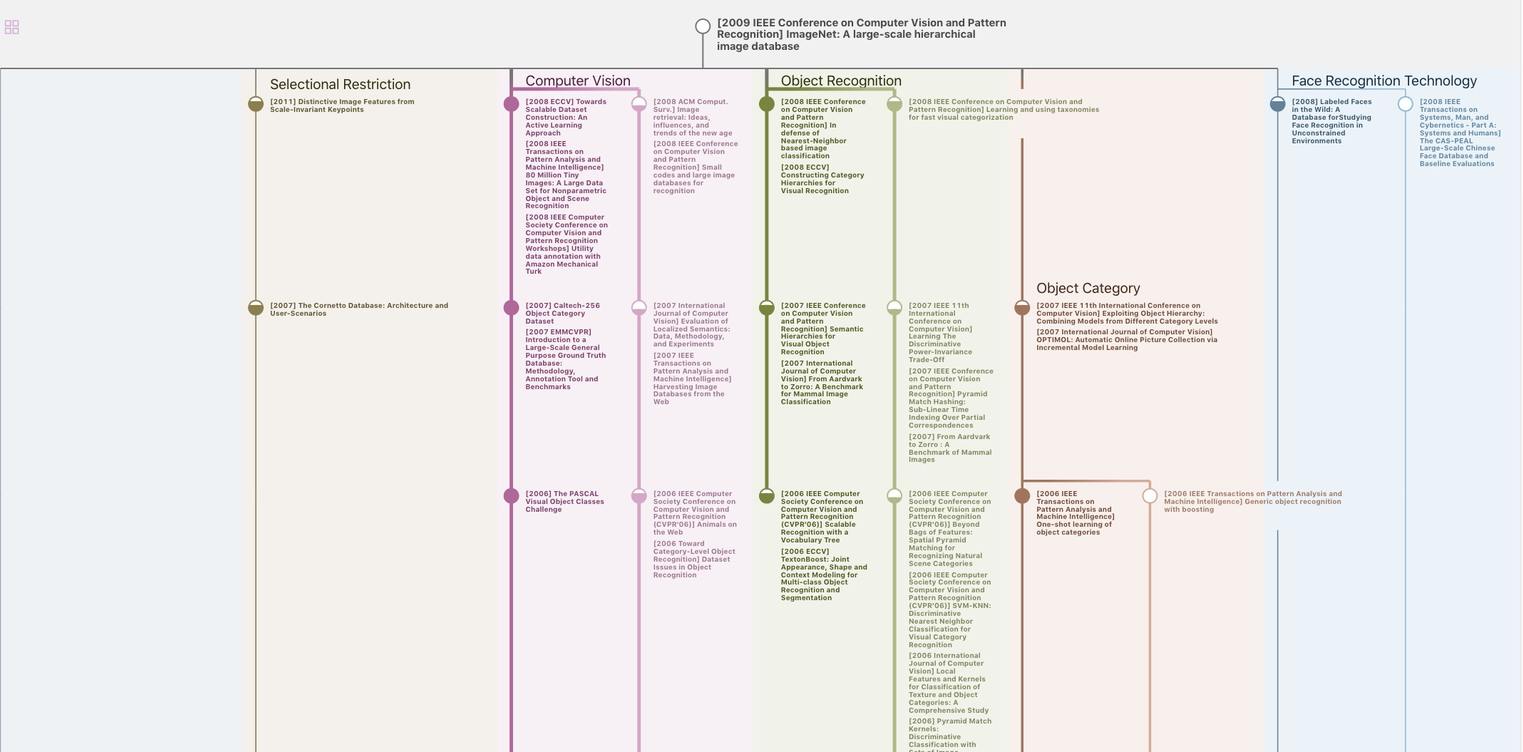
生成溯源树,研究论文发展脉络
Chat Paper
正在生成论文摘要