Aperiodic processes explaining rhythms in behavior: A matter of false detection or definition?
crossref(2022)
摘要
Behavioral studies suggest attention-related performance fluctuates at a 3-8Hz rhythm (Landau and Fries, 2012; Fiebelkorn et al., 2013). Brookshire (2022) argues that these previous studies failed to distinguish periodic from aperiodic processes, which led to the spurious detection of rhythms. The argument is based on simulations of accuracy time courses (ATCs) by autoregressive models of order 1 (AR(1)), which is a known aperiodic process. Brookshire shows that for this aperiodic process, conventional methods often detect spurious peaks in the 3-8Hz range. Here, we argue that stationary stochastic AR(1) processes are invalid models of ATCs in general as ATCs are deterministic, non-stationary signals. When AR(1) realizations are taken as models for deterministic signals, they do not constitute per definition aperiodic processes. Brookshire’s analyses therefore do not conclusively establish that previous methods indeed detected rhythms spuriously. Furthermore, we show that because Brookshire’s method relies on AR(1) model fits, it can have very low sensitivity when non-rhythmic signals are superimposed onto rhythmic signals. Given these limitations, Brookshire’s conclusion that 0/23 reanalyzed data sets provide evidence for rhythmicity needs to be revisited with alternative methods. Here, we argue that an appropriate model of an ATCs is a deterministic signal (akin to an impulse response or event-related potential) with additive white noise due to random sampling of hits and misses. Such a deterministic ATC can be either periodic or aperiodic. We show that when the deterministic ATC is truly aperiodic, detrending and random sampling lead to spurious rhythm detection. This supports the general point that previous studies did not adequately distinguish periodic from aperiodic processes. In fact, we show that previous methods like Landau and Fries (2012) have much higher false positive ratios than is apparent from Brookshire (2022). Our analysis naturally suggest two effective alternative methods, avoiding the use of AR(1) models.
更多查看译文
AI 理解论文
溯源树
样例
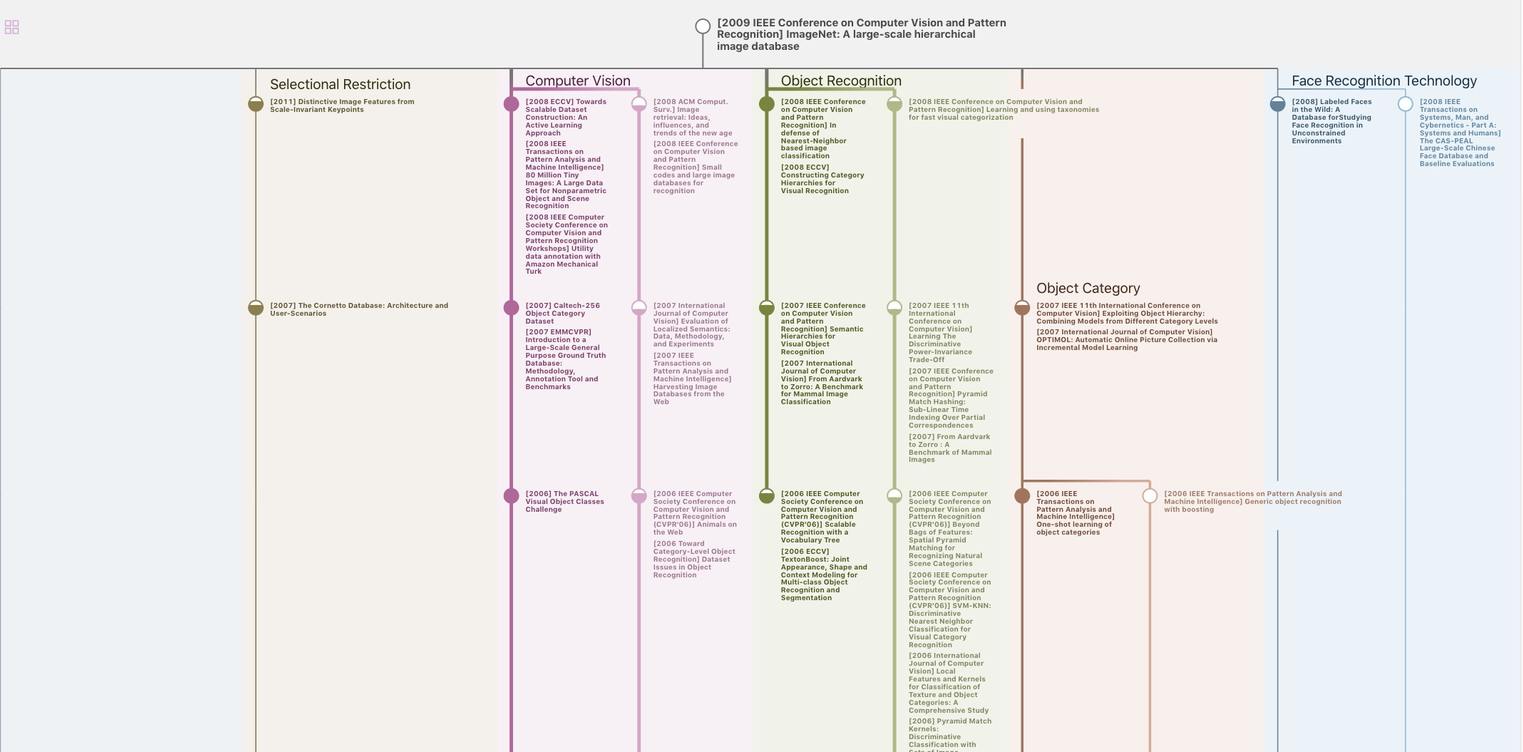
生成溯源树,研究论文发展脉络
Chat Paper
正在生成论文摘要