Classical-Assisted Quantum Ground State Preparation with Tensor Network States and Monte Carlo Sampling
arXiv (Cornell University)(2023)
摘要
Quantum computing offers potential solutions for finding ground states in condensed-matter physics and chemistry. However, achieving effective ground state preparation is also computationally hard for arbitrary Hamiltonians. It is necessary to propose certain assumptions to make this problem efficiently solvable, including preparing a trial state of a non-trivial overlap with the genuine ground state. Here, we propose a classical-assisted quantum ground state preparation method for quantum many-body systems, combining Tensor Network States (TNS) and Monte Carlo (MC) sampling as a heuristic method to prepare a trial state with a non-trivial overlap with the genuine ground state. We extract a sparse trial state by sampling from TNS, which can be efficiently prepared by a quantum algorithm on early fault-tolerant quantum computers. Our method demonstrates a polynomial improvement in scaling of overlap between the trial state and genuine ground state compared to random trial states, as evidenced by numerical tests on the spin-$1/2$ $J_1$-$J_2$ Heisenberg model. Furthermore, our method is a novel approach to hybridize a classical numerical method and a quantum algorithm and brings inspiration to ground state preparation in other fields.
更多查看译文
关键词
tensor network
AI 理解论文
溯源树
样例
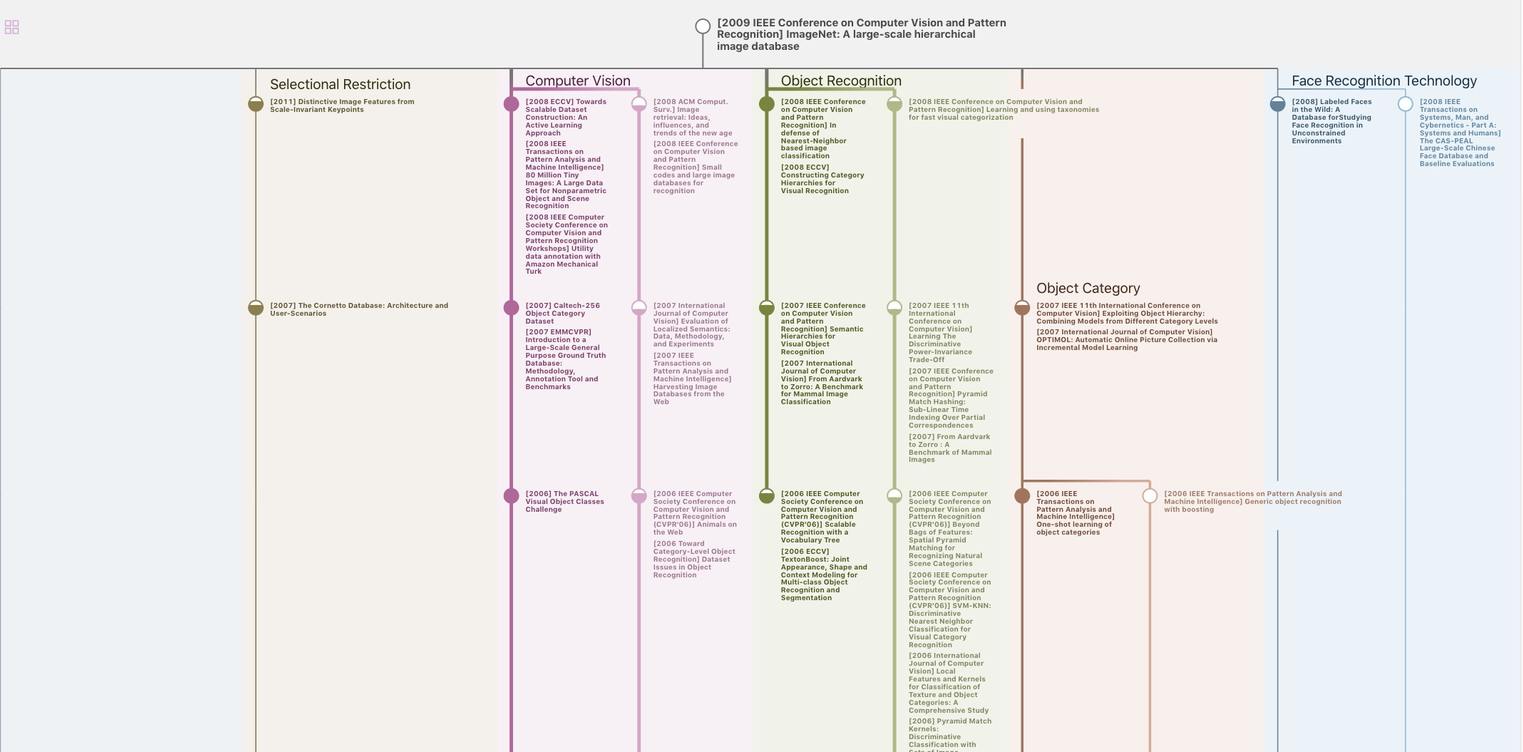
生成溯源树,研究论文发展脉络
Chat Paper
正在生成论文摘要