Exploring chemical compound space with a graph-based recommender system
arXiv (Cornell University)(2023)
摘要
With the availability of extensive databases of inorganic materials, data-driven approaches leveraging machine learning have gained prominence in materials science research. In this study, we propose an innovative adaptation of data-driven concepts to the mapping and exploration of chemical compound space. Recommender systems, widely utilized for suggesting items to users, employ techniques such as collaborative filtering, which rely on bipartite graphs composed of users, items, and their interactions. Building upon the Open Quantum Materials Database (OQMD), we constructed a bipartite graph where elements from the periodic table and sites within crystal structures are treated as separate entities. The relationships between them, defined by the presence of ions at specific sites and weighted according to the thermodynamic stability of the respective compounds, allowed us to generate an embedding space that contains vector representations for each ion and each site. Through the correlation of ion-site occupancy with their respective distances within the embedding space, we explored new ion-site occupancies, facilitating the discovery of novel stable compounds. Moreover, the graph's embedding space enabled a comprehensive examination of chemical similarities among elements, and a detailed analysis of local geometries of sites. To demonstrate the effectiveness and robustness of our method, we conducted a historical evaluation using different versions of the OQMD and recommended new compounds with Kagome lattices, showcasing the applicability of our approach to practical materials design.
更多查看译文
关键词
chemical compound space,graph-based
AI 理解论文
溯源树
样例
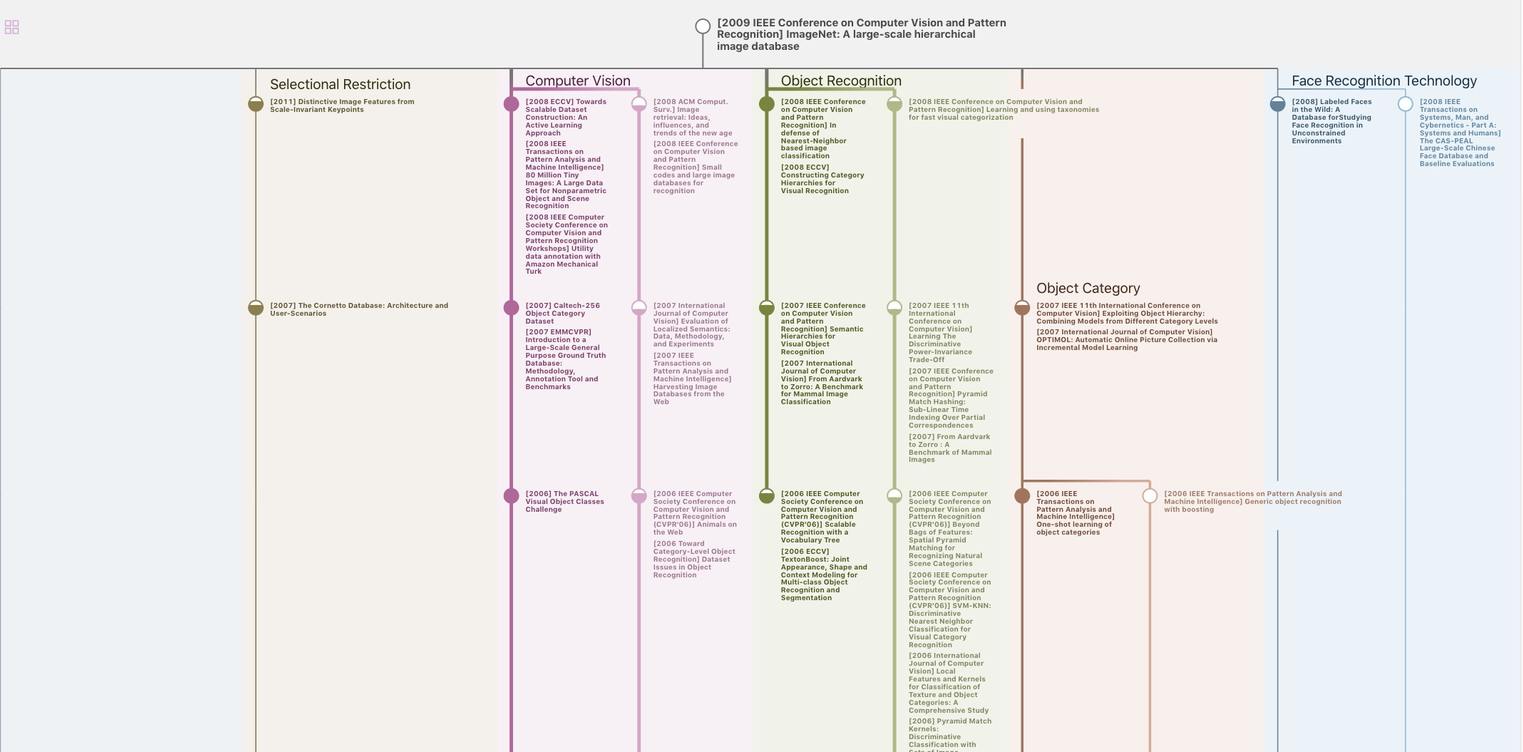
生成溯源树,研究论文发展脉络
Chat Paper
正在生成论文摘要