Systematic Analysis of the Impact of Label Noise Correction on ML Fairness
ADVANCES IN ARTIFICIAL INTELLIGENCE, AI 2023, PT II(2024)
摘要
Arbitrary, inconsistent, or faulty decision-making raises serious concerns, and preventing unfair models is an increasingly important challenge in Machine Learning. Data often reflect past discriminatory behavior, and models trained on such data may reflect bias on sensitive attributes, such as gender, race, or age. One approach to developing fair models is to preprocess the training data to remove the underlying biases while preserving the relevant information, for example, by correcting biased labels. While multiple label noise correction methods are available, the information about their behavior in identifying discrimination is very limited. In this work, we develop an empirical methodology to systematically evaluate the effectiveness of label noise correction techniques in ensuring the fairness of models trained on biased datasets. Our methodology involves manipulating the amount of label noise and can be used with fairness benchmarks but also with standard ML datasets. We apply the methodology to analyze six label noise correction methods according to several fairness metrics on standard OpenML datasets. Our results suggest that the Hybrid Label Noise Correction [20] method achieves the best trade-off between predictive performance and fairness. Clustering-Based Correction [14] can reduce discrimination the most, however, at the cost of lower predictive performance.
更多查看译文
关键词
Label noise correction,ML fairness,Bias mitigation,Semi-synthetic data
AI 理解论文
溯源树
样例
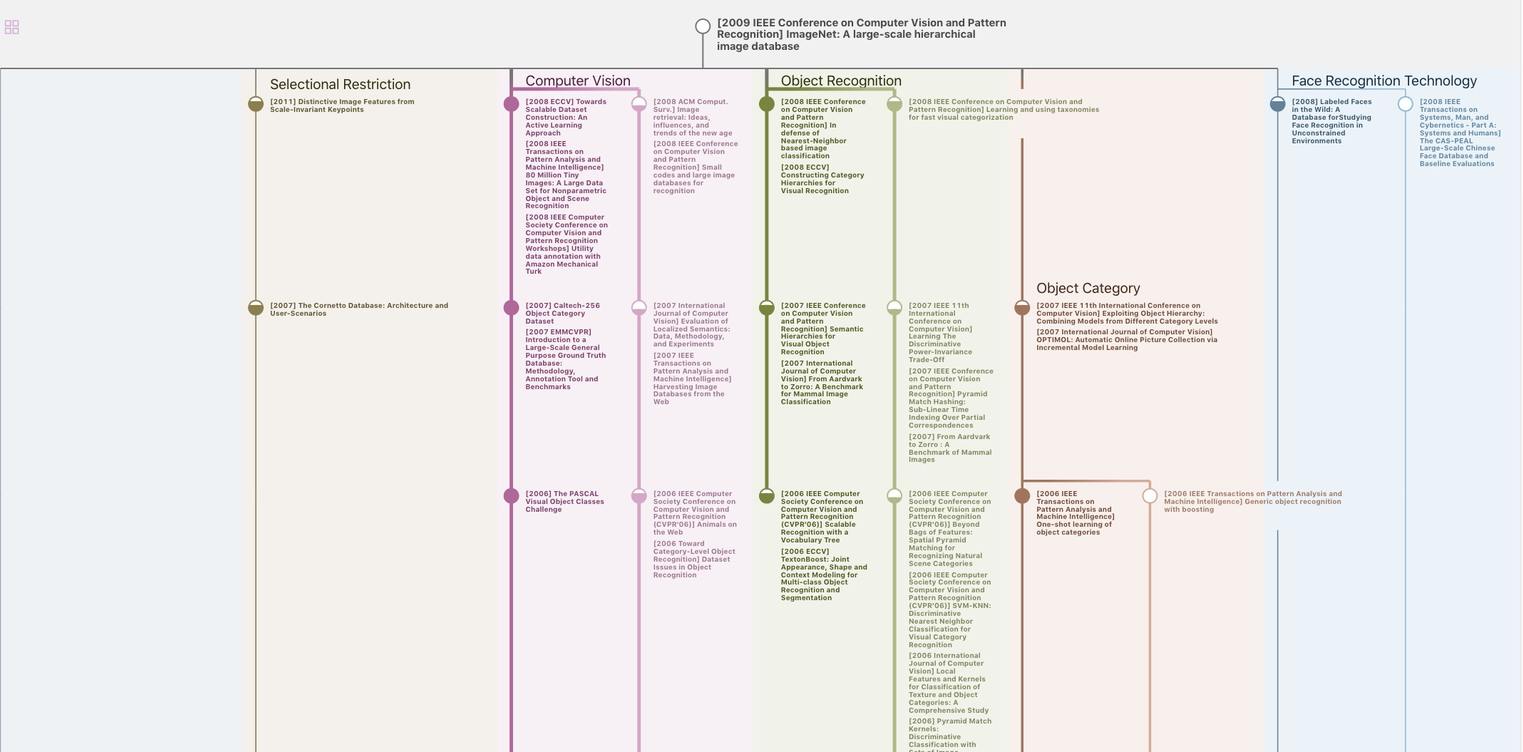
生成溯源树,研究论文发展脉络
Chat Paper
正在生成论文摘要