Federated Deep Reinforcement Learning-based Bitrate Adaptation for Dynamic Adaptive Streaming over HTTP
CoRR(2023)
摘要
In video streaming over HTTP, the bitrate adaptation selects the quality of video chunks depending on the current network condition. Some previous works have applied deep reinforcement learning (DRL) algorithms to determine the chunk's bitrate from the observed states to maximize the quality-of-experience (QoE). However, to build an intelligent model that can predict in various environments, such as 3G, 4G, Wifi, \textit{etc.}, the states observed from these environments must be sent to a server for training centrally. In this work, we integrate federated learning (FL) to DRL-based rate adaptation to train a model appropriate for different environments. The clients in the proposed framework train their model locally and only update the weights to the server. The simulations show that our federated DRL-based rate adaptations, called FDRLABR with different DRL algorithms, such as deep Q-learning, advantage actor-critic, and proximal policy optimization, yield better performance than the traditional bitrate adaptation methods in various environments.
更多查看译文
关键词
dynamic adaptive streaming,bitrate adaptation,http,learning-based
AI 理解论文
溯源树
样例
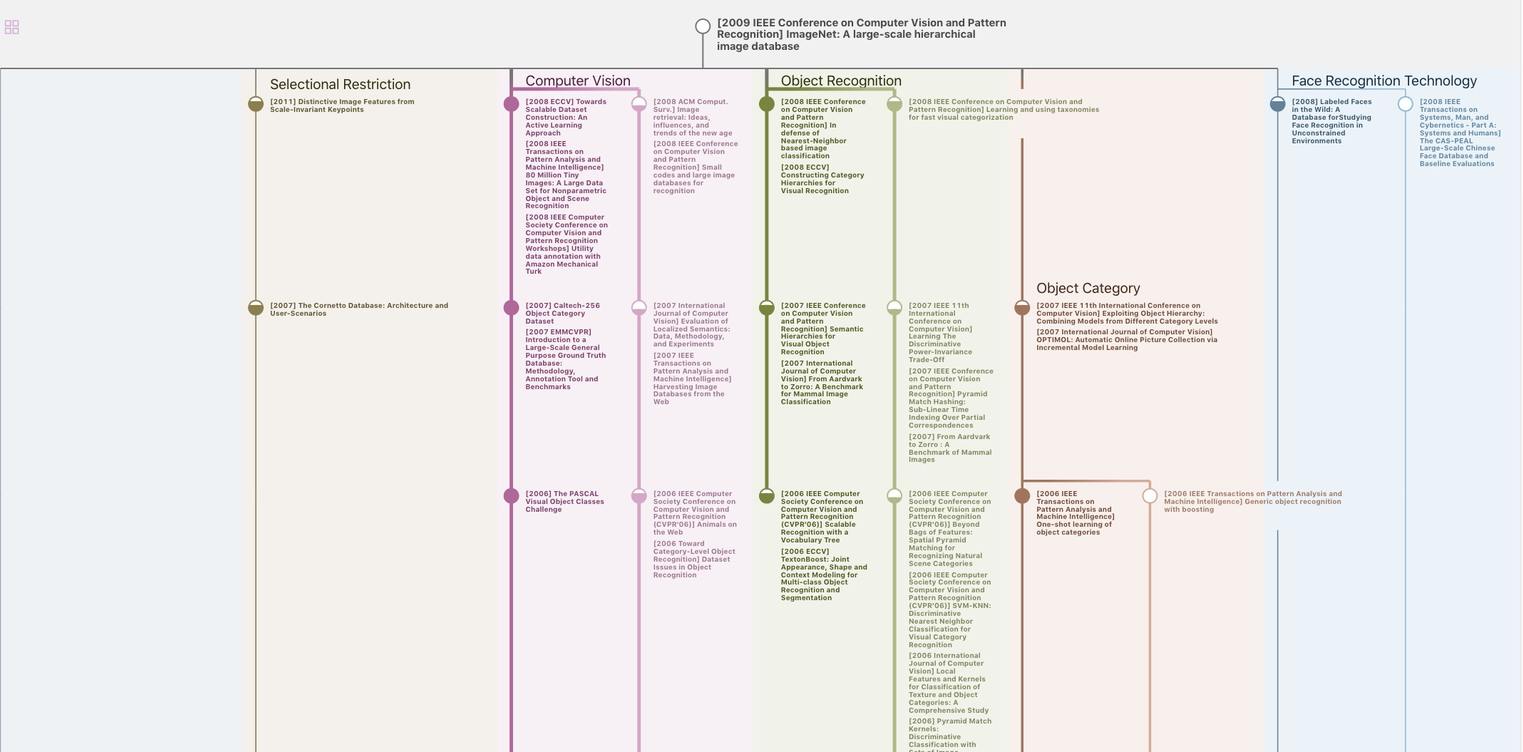
生成溯源树,研究论文发展脉络
Chat Paper
正在生成论文摘要