Coupling parameter and particle dynamics for adaptive sampling in Neural Galerkin schemes
Physica D: Nonlinear Phenomena(2024)
摘要
Training nonlinear parametrizations such as deep neural networks to numerically approximate solutions of partial differential equations is often based on minimizing a loss that includes the residual, which is analytically available in limited settings only. At the same time, empirically estimating the training loss is challenging because residuals and related quantities can have high variance, especially for transport-dominated and high-dimensional problems that exhibit local features such as waves and coherent structures. Thus, estimators based on data samples from un-informed, uniform distributions are inefficient. This work introduces Neural Galerkin schemes that estimate the training loss with data from adaptive distributions, which are empirically represented via ensembles of particles. The ensembles are actively adapted by evolving the particles with dynamics coupled to the nonlinear parametrizations of the solution fields so that the ensembles remain informative for estimating the training loss. Numerical experiments indicate that few dynamic particles are sufficient for obtaining accurate empirical estimates of the training loss, even for problems with local features and with high-dimensional spatial domains.
更多查看译文
关键词
Numerical analysis,Model reduction,Scientific machine learning,Deep neural networks,Active learning
AI 理解论文
溯源树
样例
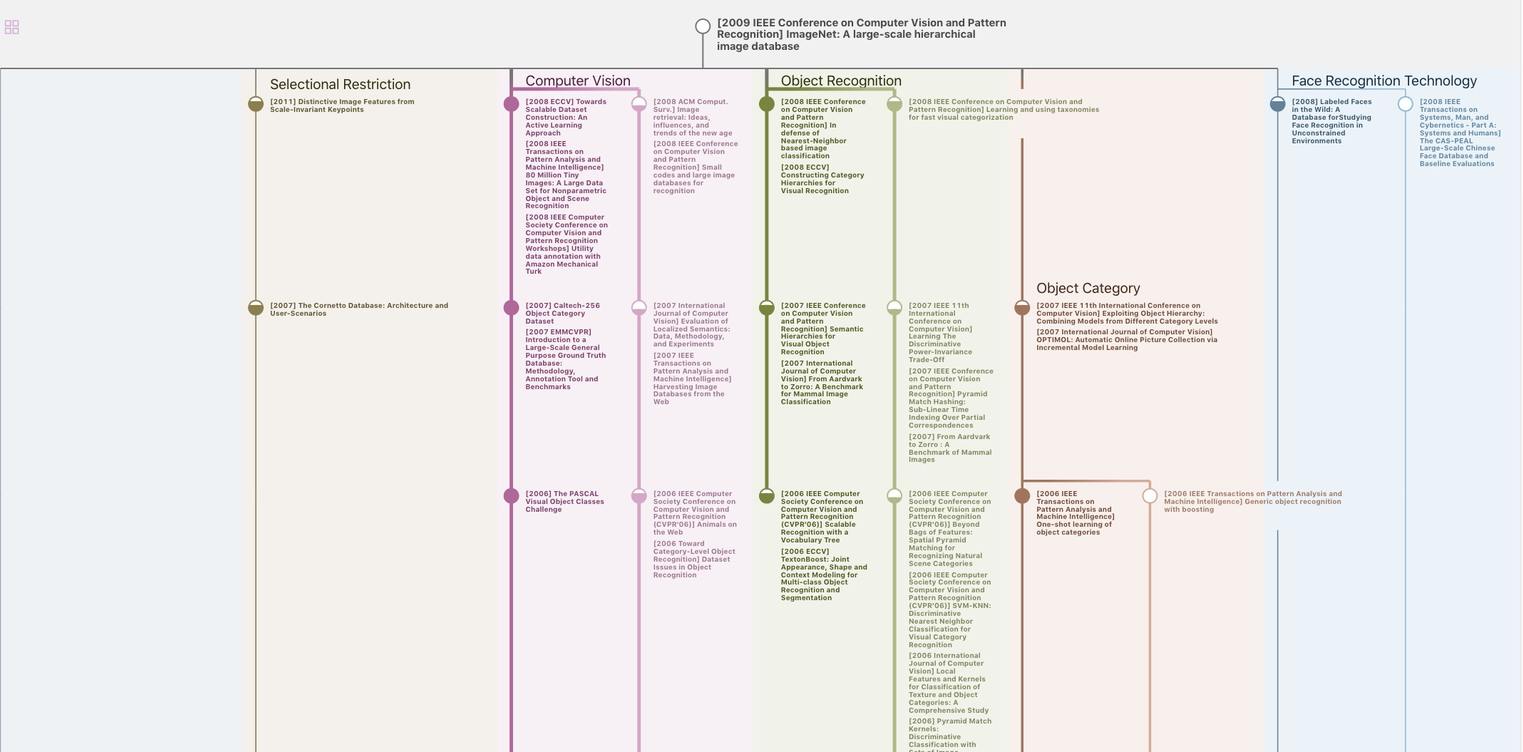
生成溯源树,研究论文发展脉络
Chat Paper
正在生成论文摘要