Predictive maintenance decision-making for variable faults with non-equivalent costs of fault severities
Advanced Engineering Informatics(2023)
摘要
Predictive Maintenance is crucial for production systems as it helps maintaining the reliability and availability of components/equipment as well as preventing unexpected shutdowns during production. Traditional maintenance strategies mostly focus on the predictive diagnosis of fault types and identical maintenance decisions would be delivered for the equipment with the same fault type. It often results in “over-maintenance” as the variable fault severities may require non-equivalent costs of maintenance resources. To tackle this problem, this paper aims at developing a fault prediction model firstly predicting fault severity and fault type simultaneously and subsequently providing distinguished maintenance strategy for variable faults accordingly, through which the abnormal faults of equipment can be effectively prevented, and machines can be efficiently and economically maintained based on the model suggested decisions. The main works in this study are 1) The fault features based on monitored vibration signals are extracted from multi-domains, and most significant features are selected by L1-Support Vector Machine (L1-SVM) together with variance filtering method; 2) A parallel fault prediction model based on Back propagation Neural Network and Long Short Term Memory Neural Network (BP-LSTM) is proposed, which is used to predict the fault type and fault degree simultaneously; 3) A Deep Q-Network (DQN)-based maintenance decision-making model is established for different fault types with various fault severities.
更多查看译文
关键词
Feature Selection,BP-LSTM,DQN,Fault prediction,Fault severity,Maintenance decision-making
AI 理解论文
溯源树
样例
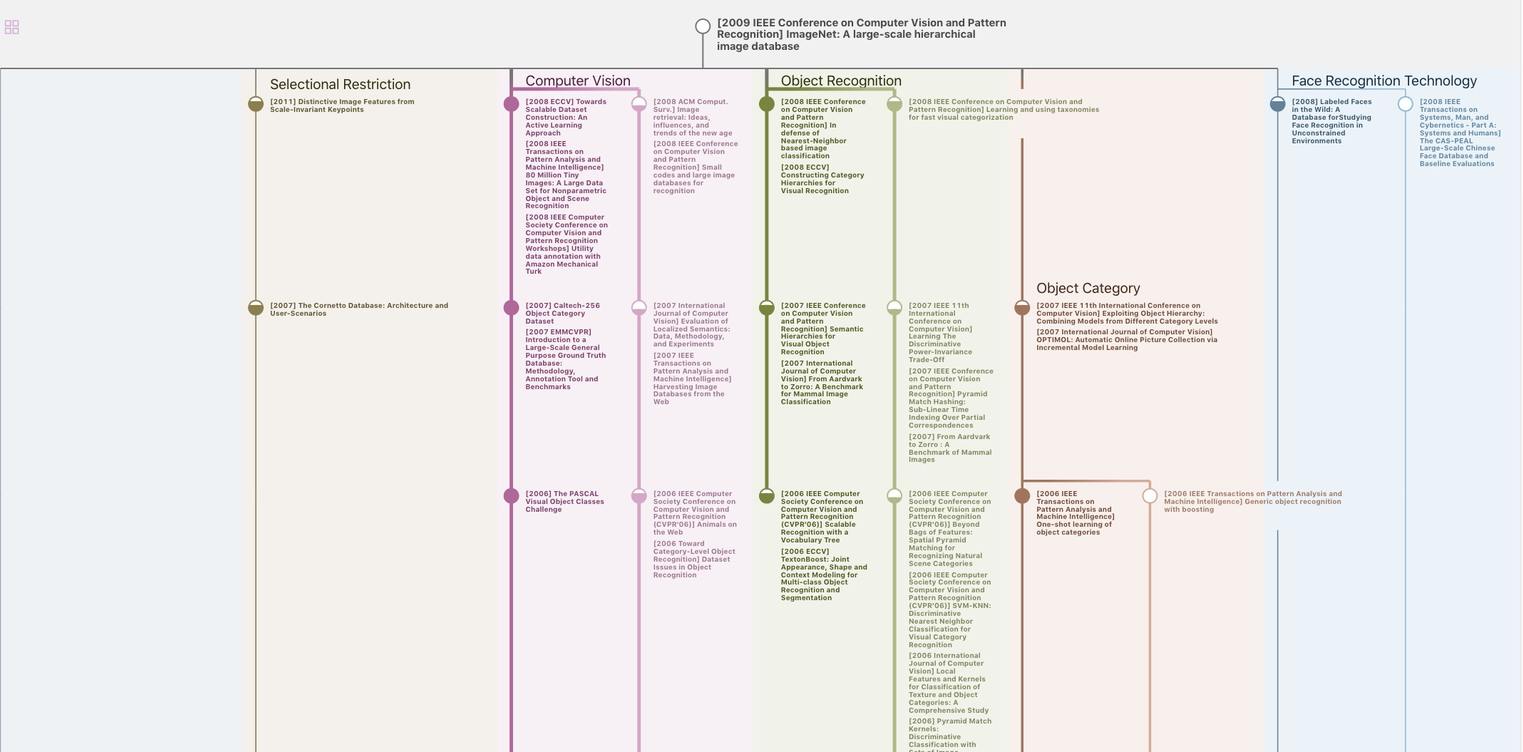
生成溯源树,研究论文发展脉络
Chat Paper
正在生成论文摘要