ParameterNet: Parameters Are All You Need
arXiv (Cornell University)(2023)
摘要
The large-scale visual pretraining has significantly improve the performance
of large vision models. However, we observe the low FLOPs pitfall that
the existing low-FLOPs models cannot benefit from large-scale pretraining. In
this paper, we introduce a novel design principle, termed ParameterNet, aimed
at augmenting the number of parameters in large-scale visual pretraining models
while minimizing the increase in FLOPs. We leverage dynamic convolutions to
incorporate additional parameters into the networks with only a marginal rise
in FLOPs. The ParameterNet approach allows low-FLOPs networks to take advantage
of large-scale visual pretraining. Furthermore, we extend the ParameterNet
concept to the language domain to enhance inference results while preserving
inference speed. Experiments on the large-scale ImageNet-22K have shown the
superiority of our ParameterNet scheme. For example, ParameterNet-600M can
achieve higher accuracy on ImageNet than the widely-used Swin Transformer
(81.6% vs. 80.9%) and has much lower FLOPs (0.6G vs. 4.5G). In
the language domain, LLaMA-1B enhanced with ParameterNet achieves 2% higher
accuracy over vanilla LLaMA. The code will be released at
.
更多查看译文
关键词
mobile networks,parameters,pretraining,large-scale
AI 理解论文
溯源树
样例
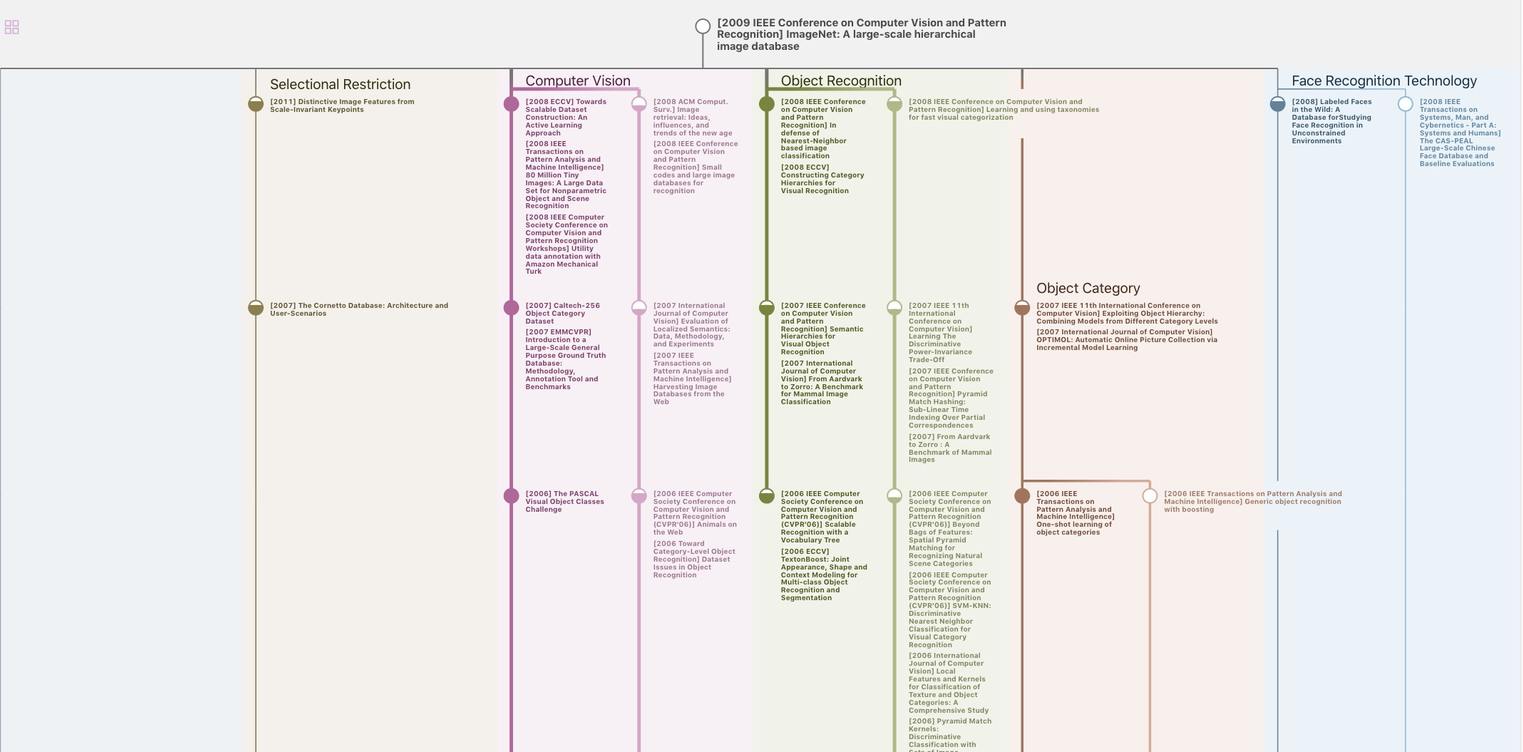
生成溯源树,研究论文发展脉络
Chat Paper
正在生成论文摘要