A Multilingual Translator to SQL with Database Schema Pruning to Improve Self-Attention
CoRR(2023)
摘要
Long sequences of text are challenging in the context of transformers, due to quadratic memory increase in the self-attention mechanism. As this issue directly affects the translation from natural language to SQL queries (as techniques usually take as input a concatenated text with the question and the database schema), we present techniques that allow long text sequences to be handled by transformers with up to 512 input tokens. We propose a training process with database schema pruning (removal of tables and columns names that are useless for the query of interest). In addition, we used a multilingual approach with the mT5-large model fine-tuned with a data-augmented Spider dataset in four languages simultaneously: English, Portuguese, Spanish, and French. Our proposed technique used the Spider dataset and increased the exact set match accuracy results from 0.718 to 0.736 in a validation dataset (Dev). Source code, evaluations, and checkpoints are available at: \underline{https://github.com/C4AI/gap-text2sql}.
更多查看译文
关键词
Semantic parsing, SQL generation, Deep learning, Neural network, Natural language process, Text-to-SQL, Databases, Transformers self-attention, Transformers, Spider dataset
AI 理解论文
溯源树
样例
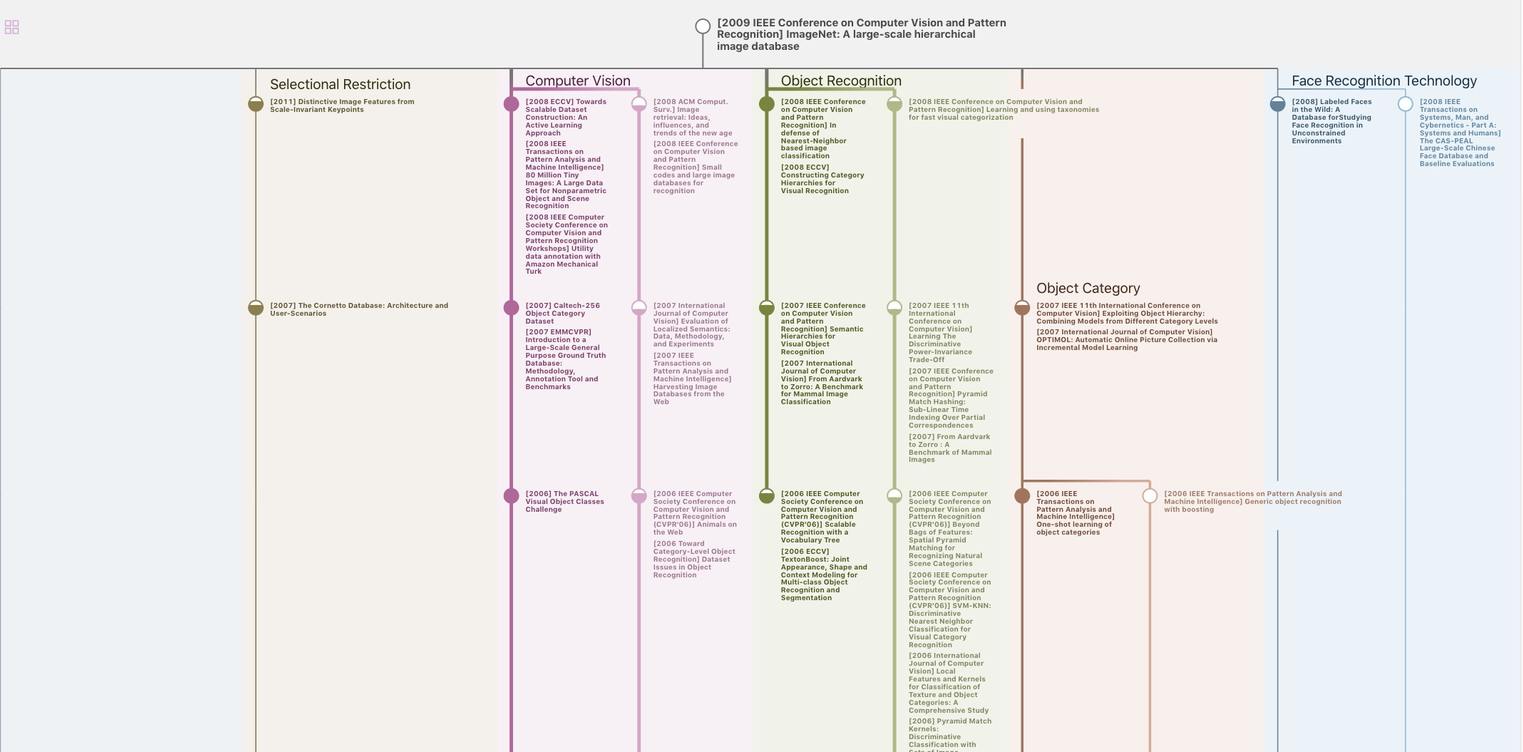
生成溯源树,研究论文发展脉络
Chat Paper
正在生成论文摘要