Federated Zero-Shot Industrial Fault Diagnosis With Cloud-Shared Semantic Knowledge Base
IEEE Internet of Things Journal(2023)
摘要
Recently, a considerable literature has grown up around the few-sample fault diagnosis task, in which few samples of fault data are available for model training. The lack of fault samples usually causes significant degradation of diagnosis performance, which is referred as few-sample problem. Many federated learning-based fault diagnosis methods are proposed as a popular strategy to solve this problem. However, the vast majority of these algorithms assume that few-sample categories of the local client are well-sampled in some clients. In this sense, they are unable to cope with faults that have rare or even no sample in all clients, namely, global unseen faults. To diagnosis global unseen fault categories, a federated zero-shot fault diagnosis framework is proposed in this article. First, we propose a general approach for building a semantic knowledge base, which provides auxiliary discriminant descriptions of different faults. Second, a bidirectional alignment network is designed using two coupled variational autoencoders, enabling the fusion of data and attributes and allowing attribute descriptions to assist in fault diagnosis tasks. Third, a cloud–edge collaboration model aggregation strategy is developed, which utilizes a generative replay mechanism and integrates the knowledge of each client, thus, enhancing the generalization and generation ability of the global model. Experiments conducted on the thermal power plant group illustrate the feasibility and effectiveness of the proposed framework to categorize local and global unseen classes.
更多查看译文
关键词
fault,knowledge,zero-shot,cloud-shared
AI 理解论文
溯源树
样例
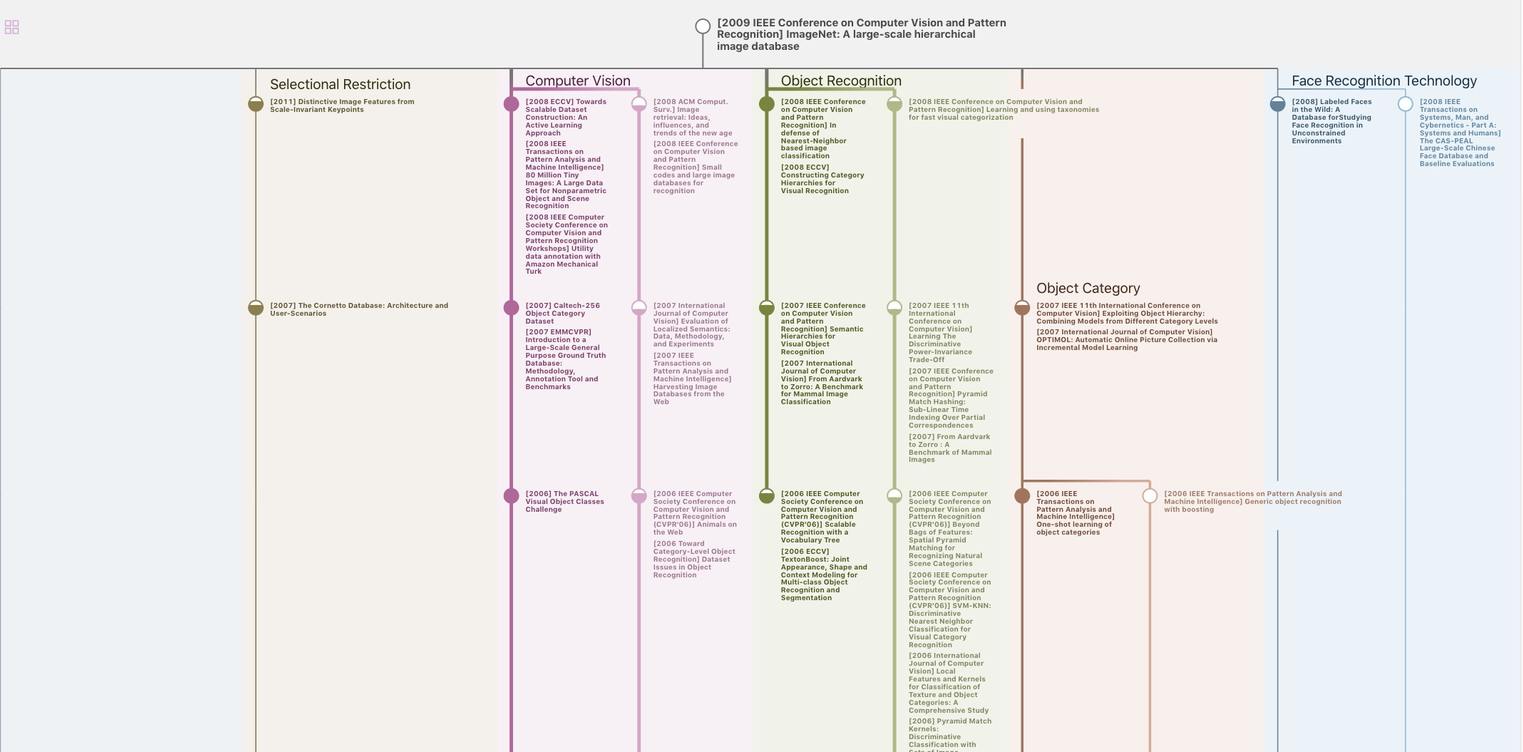
生成溯源树,研究论文发展脉络
Chat Paper
正在生成论文摘要