Adaptive Bernstein change detector for high-dimensional data streams
Data Mining and Knowledge Discovery(2024)
摘要
Change detection is of fundamental importance when analyzing data streams. Detecting changes both quickly and accurately enables monitoring and prediction systems to react, e.g., by issuing an alarm or by updating a learning algorithm. However, detecting changes is challenging when observations are high-dimensional. In high-dimensional data, change detectors should not only be able to identify when changes happen, but also in which subspace they occur. Ideally, one should also quantify how severe they are. Our approach, ABCD, has these properties. ABCD learns an encoder-decoder model and monitors its accuracy over a window of adaptive size. ABCD derives a change score based on Bernstein’s inequality to detect deviations in terms of accuracy, which indicate changes. Our experiments demonstrate that ABCD outperforms its best competitor by up to 20% in F1-score on average. It can also accurately estimate changes’ subspace, together with a severity measure that correlates with the ground truth.
更多查看译文
关键词
Change detection,Concept drift,Data streams,High-dimensionality
AI 理解论文
溯源树
样例
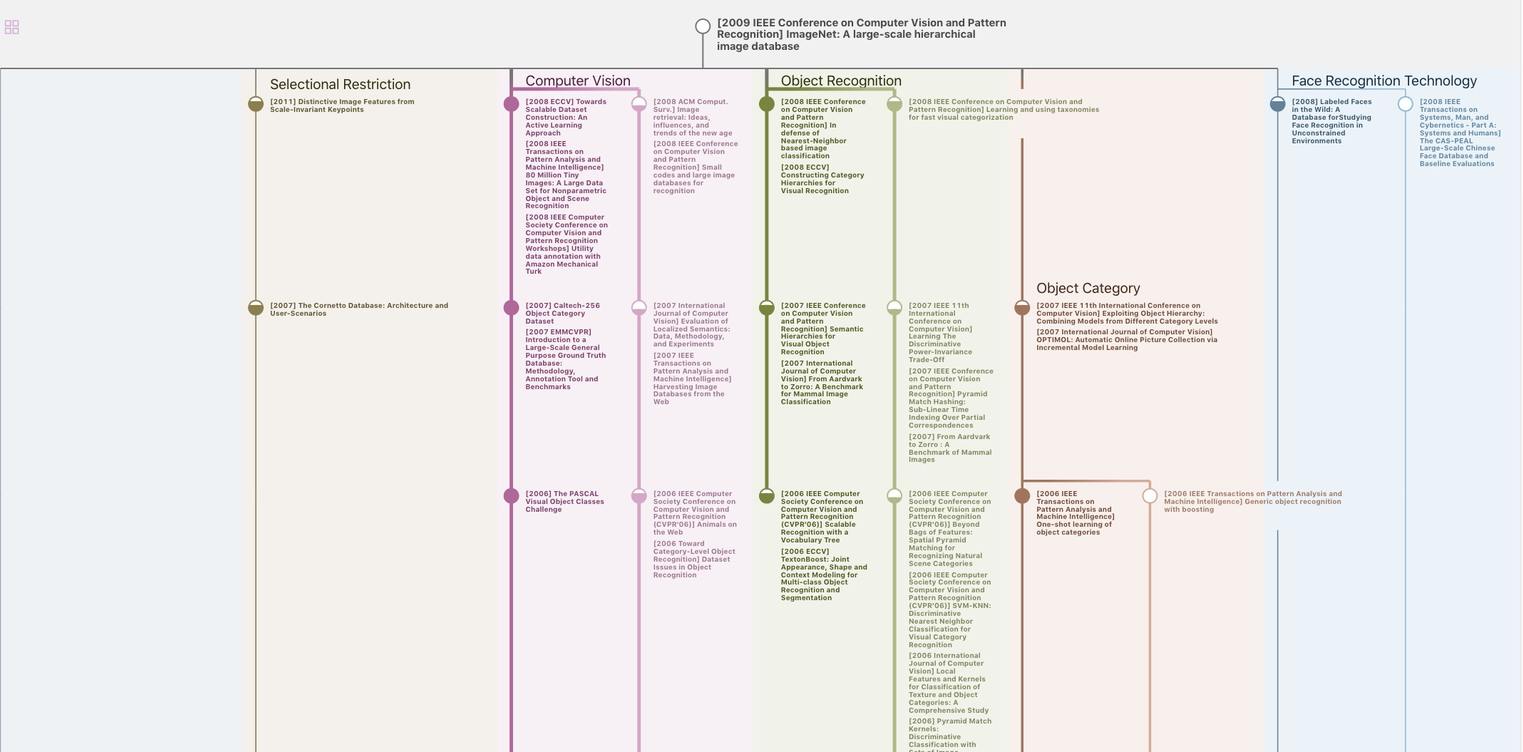
生成溯源树,研究论文发展脉络
Chat Paper
正在生成论文摘要