A Gradient Descent-Ascent Method for Continuous-Time Risk-Averse Optimal Control
arXiv (Cornell University)(2023)
摘要
In this paper, we consider continuous-time stochastic optimal control problems where the cost is evaluated through a coherent risk measure. We provide an explicit gradient descent-ascent algorithm which applies to problems subject to non-linear stochastic differential equations. More specifically, we leverage duality properties of coherent risk measures to relax the problem via a smooth min-max reformulation which induces artificial strong concavity in the max subproblem. We then formulate necessary conditions of optimality for this relaxed problem which we leverage to prove convergence of the gradient descent-ascent algorithm to candidate solutions of the original problem. Finally, we showcase the efficiency of our algorithm through numerical simulations involving trajectory tracking problems and highlight the benefit of favoring risk measures over classical expectation.
更多查看译文
关键词
optimal
AI 理解论文
溯源树
样例
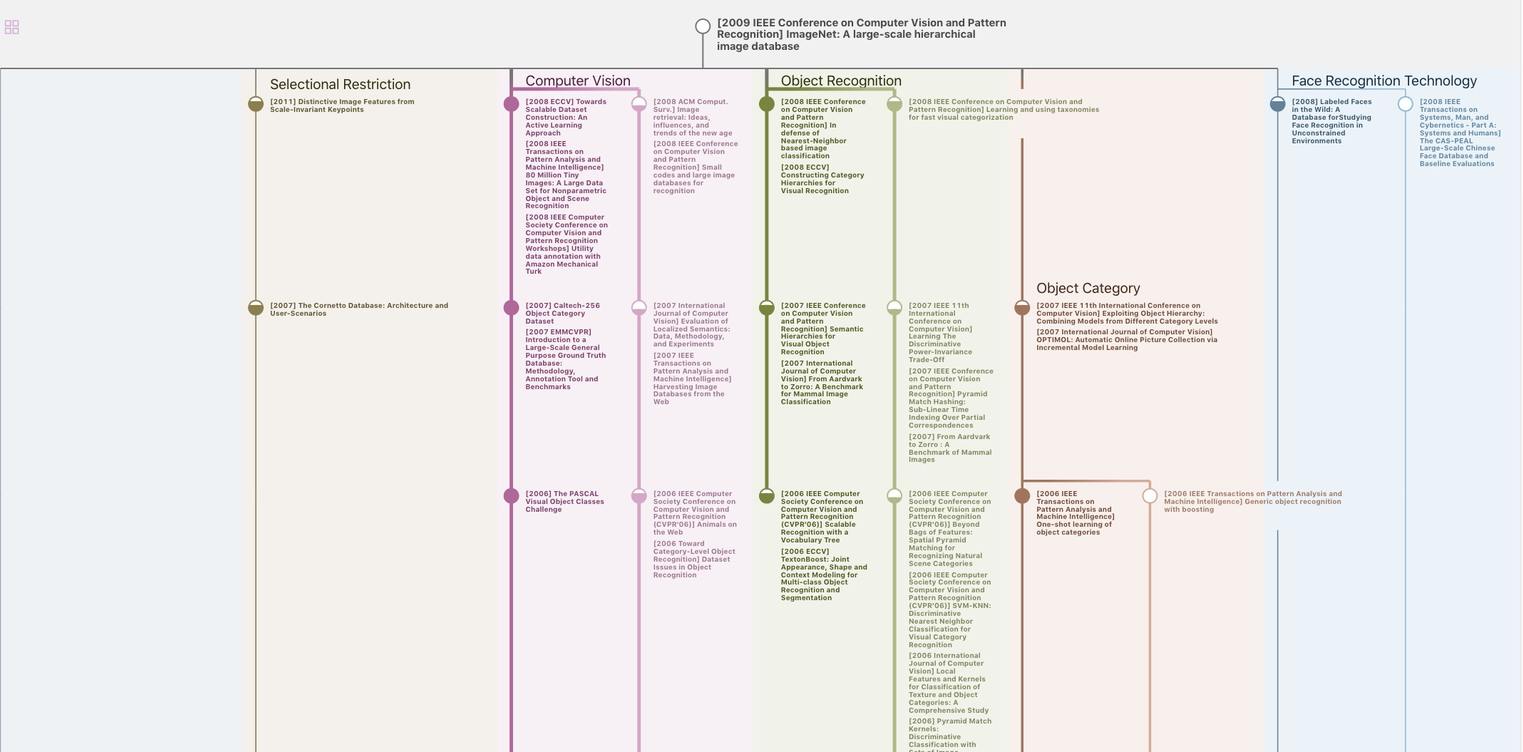
生成溯源树,研究论文发展脉络
Chat Paper
正在生成论文摘要