Generative Multimodal Entity Linking
International Conference on Language Resources and Evaluation(2023)
摘要
Multimodal Entity Linking (MEL) is the task of mapping mentions with multimodal contexts to the referent entities from a knowledge base (e.g., Wikipedia). Prior MEL methods mainly focus on designing complex multimodal interaction mechanisms and require fine-tuning all model parameters, which can be prohibitively costly and difficult to scale in the era of Large Language Models (LLMs). In this work, we propose GEMEL, a simple yet effective Generative Multimodal Entity Linking method, which leverages the capabilities of LLMs from large-scale pre-training to directly generate target entity names. We keep the vision and language model frozen and only train a linear layer to enable cross-modality interactions. To adapt LLMs to the MEL task, we take advantage of the emerging in-context learning (ICL) capability of LLMs by retrieving multimodal instances as demonstrations. Extensive experiments show that with only ~0.3% of the model parameters fine-tuned, GEMEL achieves state-of-the-art results on two well-established MEL datasets (4.1% accuracy gains on WikiDiverse and 15.4% accuracy gains on WikiMEL). Our approach is compatible with any off-the-shelf language model, paving the way towards an efficient and general solution for utilizing LLMs in the MEL task.
更多查看译文
AI 理解论文
溯源树
样例
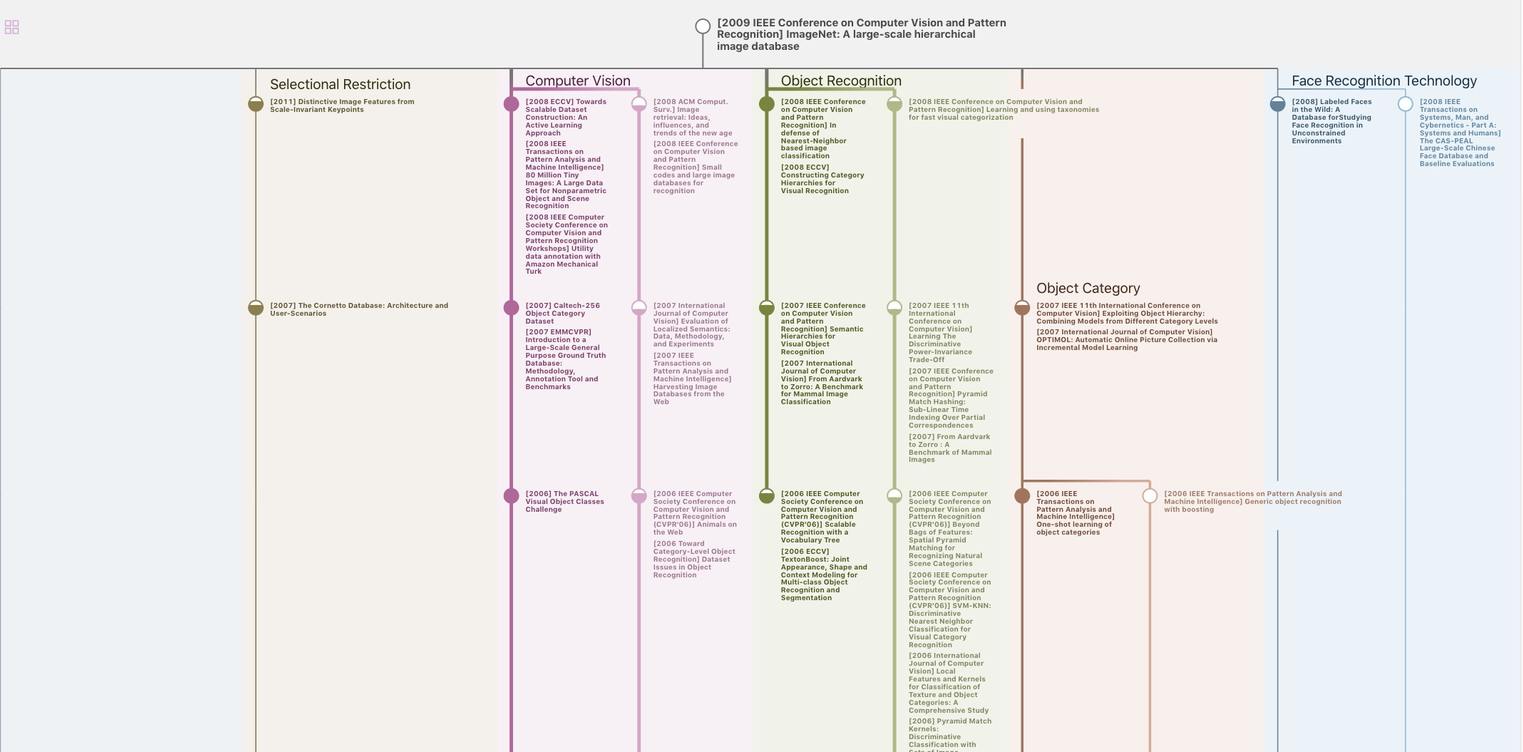
生成溯源树,研究论文发展脉络
Chat Paper
正在生成论文摘要