Learnability and Algorithm for Continual Learning
CoRR(2023)
摘要
This paper studies the challenging continual learning (CL) setting of Class Incremental Learning (CIL). CIL learns a sequence of tasks consisting of disjoint sets of concepts or classes. At any time, a single model is built that can be applied to predict/classify test instances of any classes learned thus far without providing any task related information for each test instance. Although many techniques have been proposed for CIL, they are mostly empirical. It has been shown recently that a strong CIL system needs a strong within-task prediction (WP) and a strong out-of-distribution (OOD) detection for each task. However, it is still not known whether CIL is actually learnable. This paper shows that CIL is learnable. Based on the theory, a new CIL algorithm is also proposed. Experimental results demonstrate its effectiveness.
更多查看译文
关键词
learning,algorithm
AI 理解论文
溯源树
样例
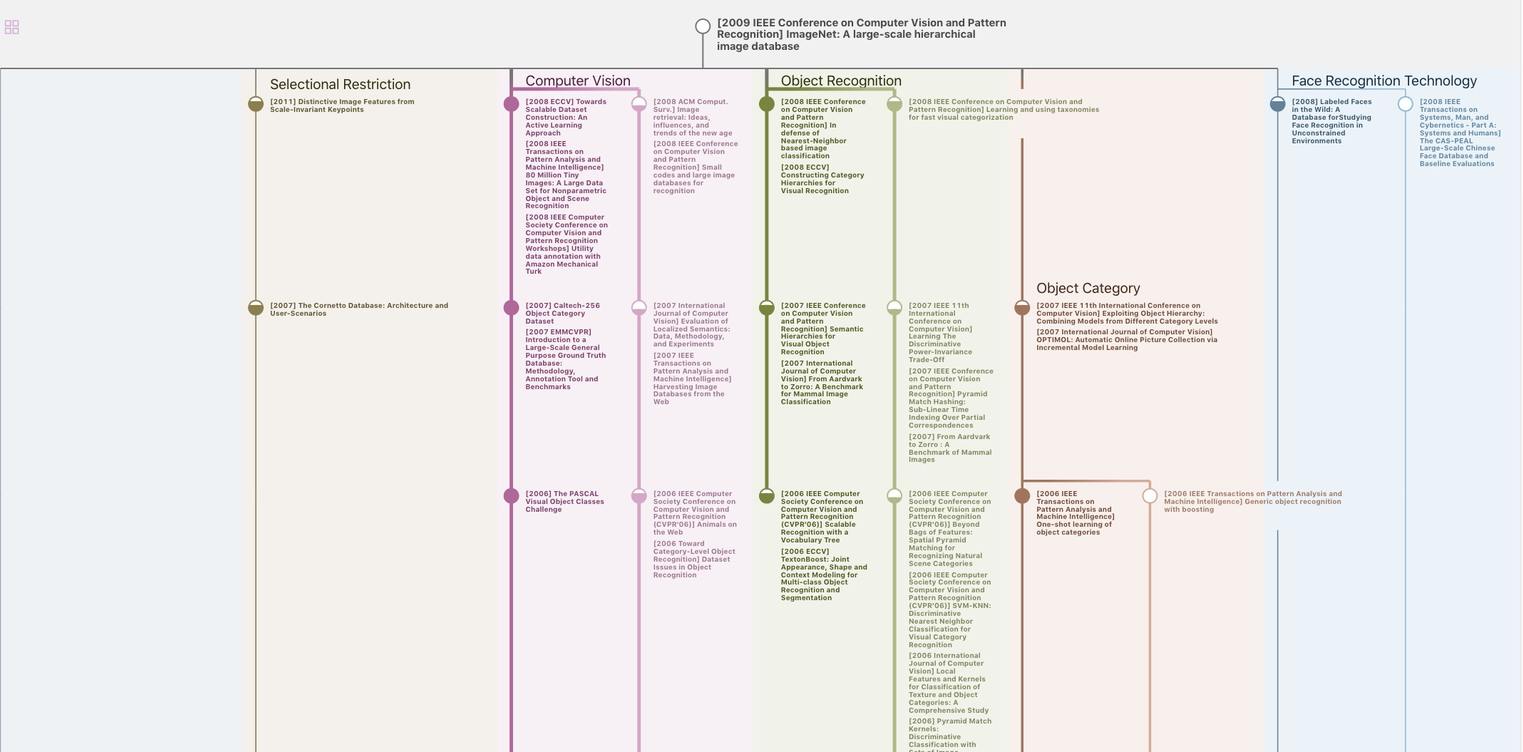
生成溯源树,研究论文发展脉络
Chat Paper
正在生成论文摘要