Self-Tuning Nonlinear Iterative Learning for a Precision Testing Stage: A Set-Membership Approach
IEEE Transactions on Industrial Informatics(2023)
摘要
Iterative learning control (ILC) is an appealing method in motion control applications that can achieve the performance limit of feedforward compensation in repeating tasks. Compensating for the repetitive errors rapidly (G1) and at the same time reducing the accumulation of the nonrepetitive errors effectively (G2) are two major goals of ILC. However, in the simultaneous presence of model uncertainties and noises, most ILC methods that have been used in the application cannot fully achieve these two goals. To tackle this problem, this article develops a self-tuning nonlinear ILC method (STNILC). First, a new nonlinear ILC method (NILC) is designed in the set-membership framework based on the boundedness noise-uncertainty assumption. Then, a self-tuning algorithm is developed to determine the unknown noise-uncertainty bound that is required by NILC. Theoretical results confirm that desirable transient and steady-state convergence performances can be achieved by the proposed STNILC method. Comparative experimental results on a short-stroke stage for testing the lithographic projection lens verify the superiorities of STNILC in sufficiently achieving G1 and G2.
更多查看译文
关键词
precision testing stage,learning,self-tuning,set-membership
AI 理解论文
溯源树
样例
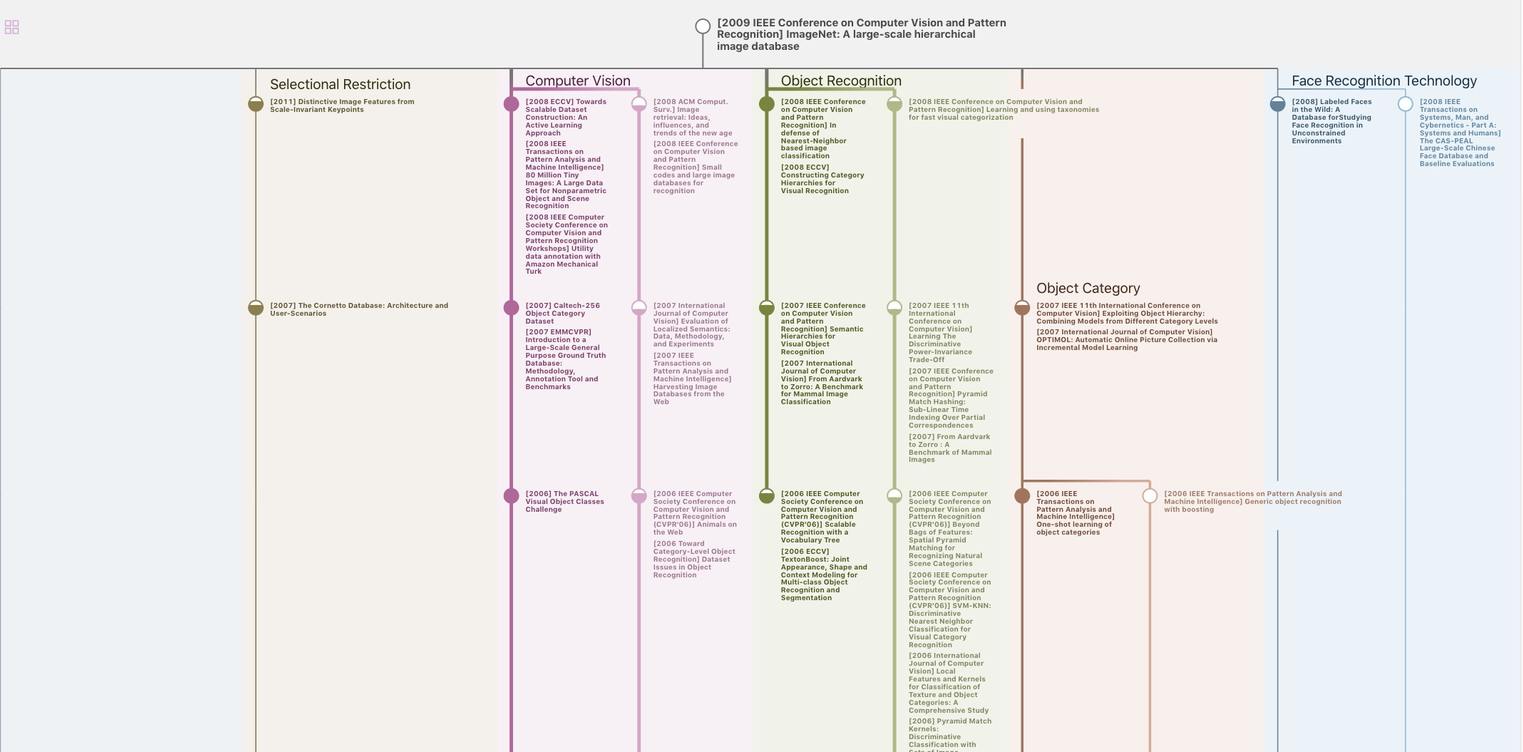
生成溯源树,研究论文发展脉络
Chat Paper
正在生成论文摘要