Wildfire detection via transfer learning: a survey
Signal, Image and Video Processing(2024)
摘要
This paper presents a comprehensive survey of publicly available neural network models specifically designed for detecting wildfires using regular visible-range cameras positioned on hilltops or forest lookout towers. The surveyed models are first pre-trained on the ImageNet-1K dataset and then fine-tuned on a custom wildfire dataset to enhance their performance. Evaluations are conducted on diverse wildfire images, enabling a thorough assessment of their capabilities. The survey findings provide valuable insights for individuals interested in leveraging transfer learning techniques for wildfire detection. Among the examined models, Swin Transformer-tiny achieves the highest area under the curve value, indicating strong overall performance in distinguishing wildfire events. However, ConvNext-tiny stands out for its exceptional ability to detect all instances of wildfires while maintaining the lowest false alarm rate within our dataset. These results highlight the varying strengths of different neural network models and offer valuable guidance for selecting an appropriate model based on specific detection requirements and priorities.
更多查看译文
关键词
Wildfire detection,Transfer learning,Convolutional neural network,Vision transformer
AI 理解论文
溯源树
样例
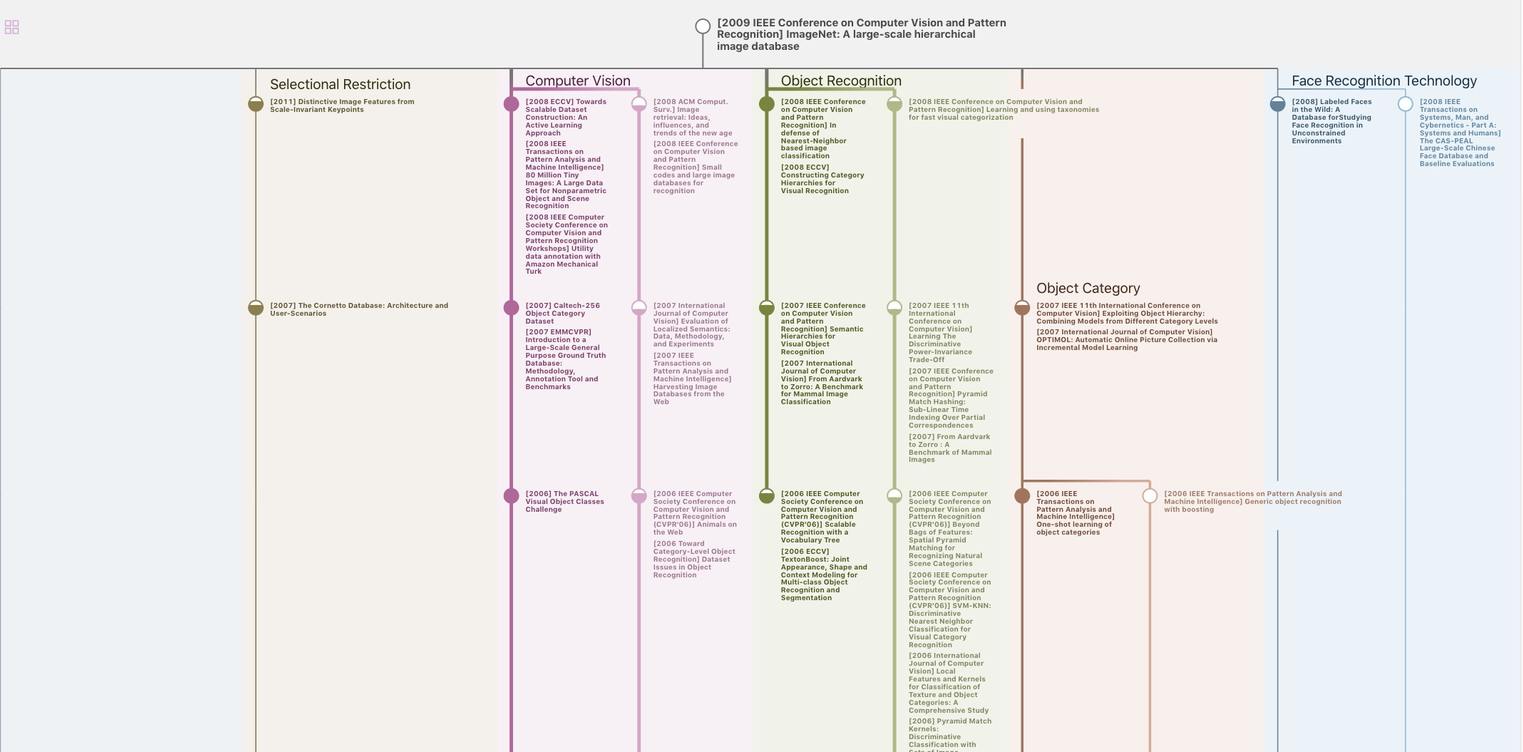
生成溯源树,研究论文发展脉络
Chat Paper
正在生成论文摘要