Efficient Machine Translation Corpus Generation
CoRR(2023)
摘要
This paper proposes an efficient and semi-automated method for human-in-the-loop post-editing for machine translation (MT) corpus generation. The method is based on online training of a custom MT quality estimation metric on-the-fly as linguists perform post-edits. The online estimator is used to prioritize worse hypotheses for post-editing, and auto-close best hypotheses without post-editing. This way, significant improvements can be achieved in the resulting quality of post-edits at a lower cost due to reduced human involvement. The trained estimator can also provide an online sanity check mechanism for post-edits and remove the need for additional linguists to review them or work on the same hypotheses. In this paper, the effect of prioritizing with the proposed method on the resulting MT corpus quality is presented versus scheduling hypotheses randomly. As demonstrated by experiments, the proposed method improves the lifecycle of MT models by focusing the linguist effort on production samples and hypotheses, which matter most for expanding MT corpora to be used for re-training them.
更多查看译文
关键词
corpus,translation,generation,efficient machine
AI 理解论文
溯源树
样例
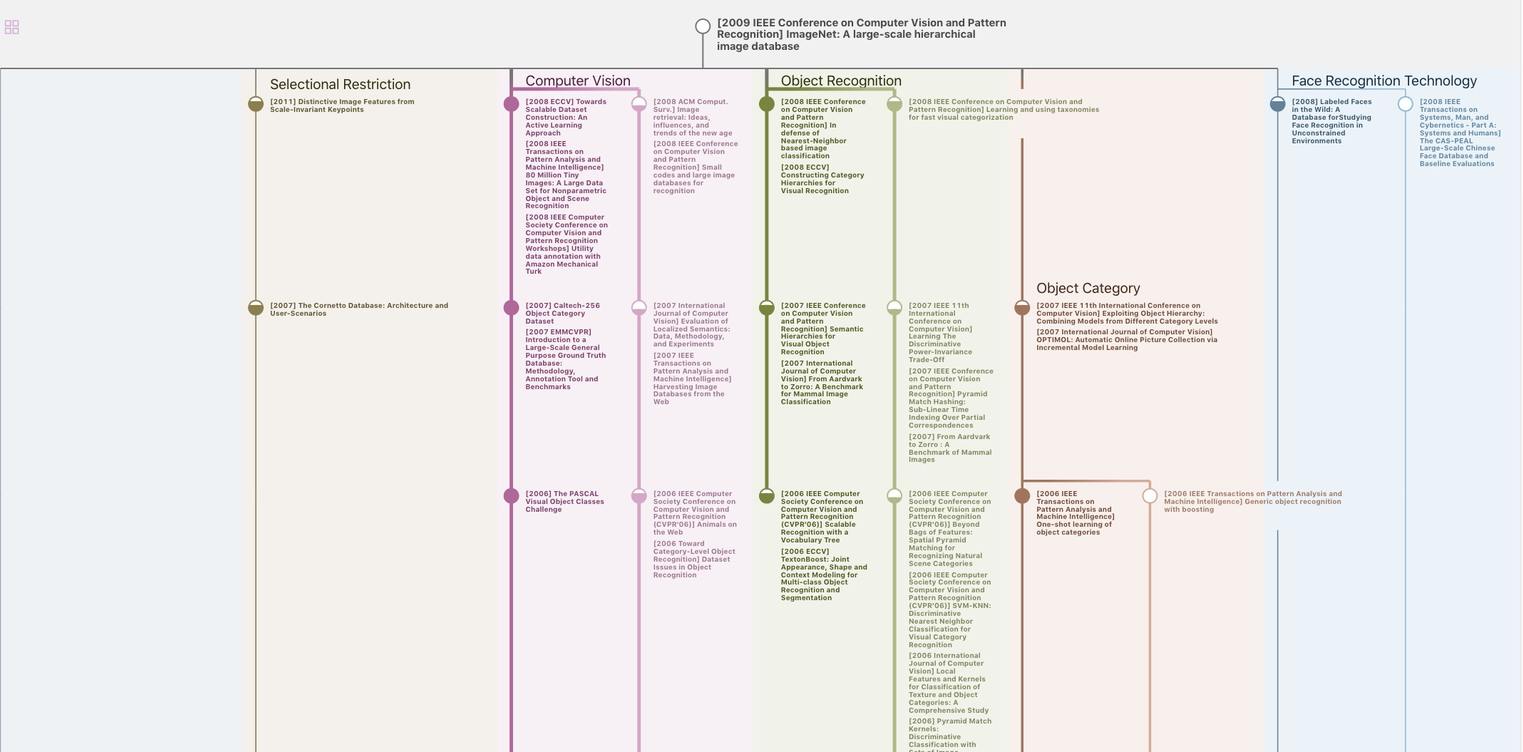
生成溯源树,研究论文发展脉络
Chat Paper
正在生成论文摘要