Machine Learning-Based Coarse Frequency Bands Classification For Cognitive Radio Applications
2023 20th International Conference on Electrical Engineering/Electronics, Computer, Telecommunications and Information Technology (ECTI-CON)(2023)
摘要
This paper is focused on multiple supervised machine learning algorithms’ performance evaluation in terms of classification accuracy and speed for the blind frequency bands classification into three occupancy classes: white, gray, and black spaces for potential implementation in cognitive radio application. Training and validation data sets consisting of 180 000 samples, including 60 000 samples per class, have been collected in the controlled experiment. Data samples have been generated using a hardware signal generator and recorded on the receiver’s front end as the time-domain complex signals. Gray space data samples contain one, two, or three signals modulated into 2FSK, BPSK, or QPSK with symbol rates 10, 100, or 1000 kSymbol/s. White space data samples contain no own generated signals. Black space data samples contain two signals with the symbol rate of 22.5 MSymbol/s and offset +14 MHz and −14 MHz from the central frequency occupying the entire observation band. Training and validation of twenty supervised machine learning algorithms have been performed offline in the Matlab Classification Learner application using the collected data set. Fine decision trees have demonstrated the highest classification accuracy of 87.8 %, the observed classification speed of 630000 Objects/s is also higher than the required 2000 Objects/s. Medium decision trees and ensemble boosted trees have demonstrated 87.5 % and 87.7 % accuracy and classification speeds of 950000 and 230000 Objects/s respectively. Therefore, ensemble boosted trees, and fine and medium decision trees have been selected for the deployment on the target radio application in the scope of future work.
更多查看译文
关键词
machine learning,decision trees,vacant frequency channels,cognitive radio
AI 理解论文
溯源树
样例
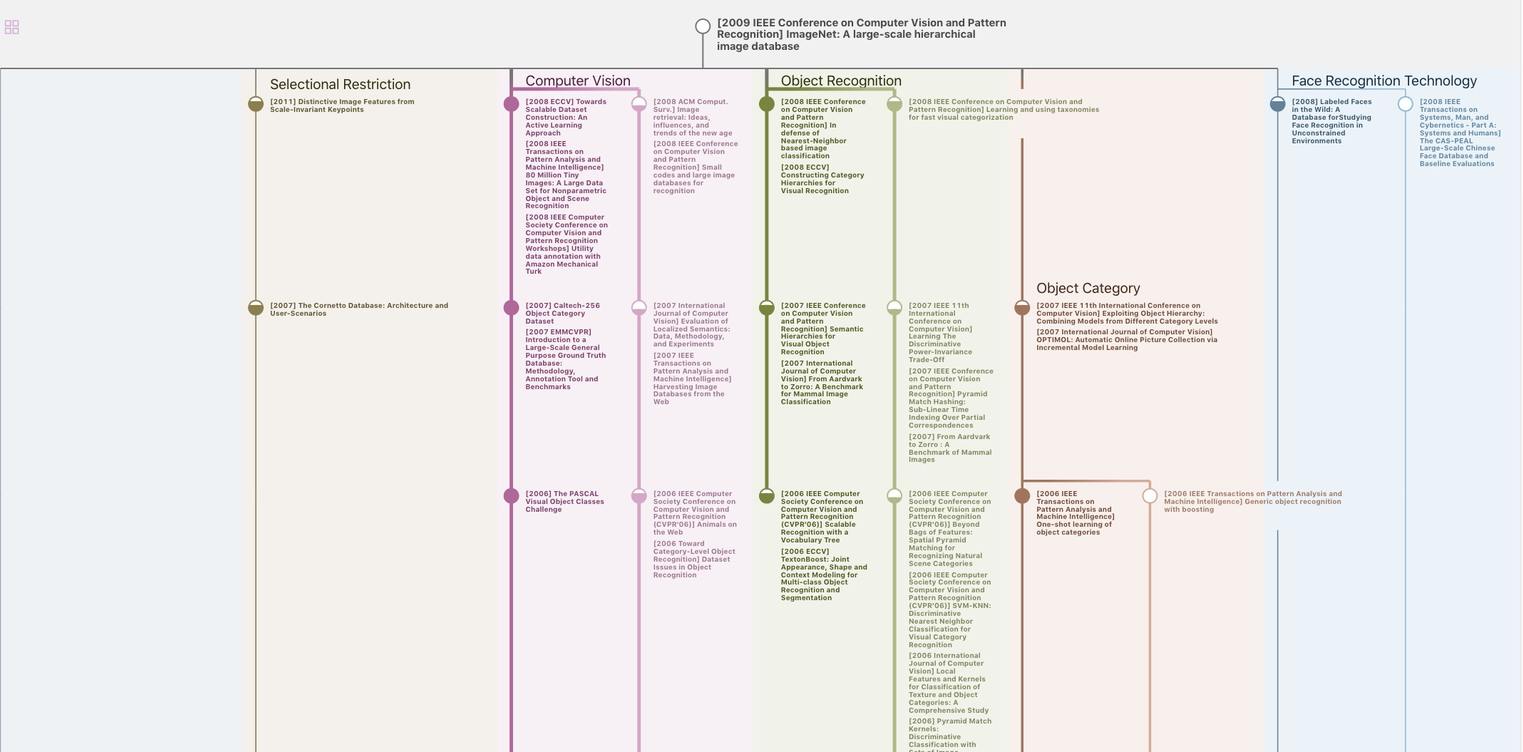
生成溯源树,研究论文发展脉络
Chat Paper
正在生成论文摘要