PAC-Chernoff Bounds: Understanding Generalization in the Interpolation Regime
CoRR(2023)
摘要
In this paper, we present a distribution-dependent PAC-Chernoff bound that is
perfectly tight for interpolators even under overparametrized model classes.
This bound relies on basic principles of Large Deviation Theory and naturally
provides a characterization of the smoothness of a model described as a simple
real-valued function. Based on this distribution-dependent bound and the novel
definition of smoothness, we propose an unifying theoretical explanation of why
some interpolators generalize remarkably well while others not. And why a wide
range of modern learning techniques (i.e., ℓ_2-norm,
distance-from-initialization, input-gradient and variance regularization
together with data augmentation, invariant architectures, and
overparameterization) are able to find them. The emergent conclusion is that
all these methods provide complimentary procedures that bias the optimizer to
smoother interpolators, which, according to this theoretical analysis, are the
ones with better generalization error. One of the main insights of this study
is that distribution-dependent bounds serve as a powerful tool better
understand the complex dynamics behind the generalization capabilities of
highly-overparameterized interpolators.
更多查看译文
关键词
interpolation regime,rate
AI 理解论文
溯源树
样例
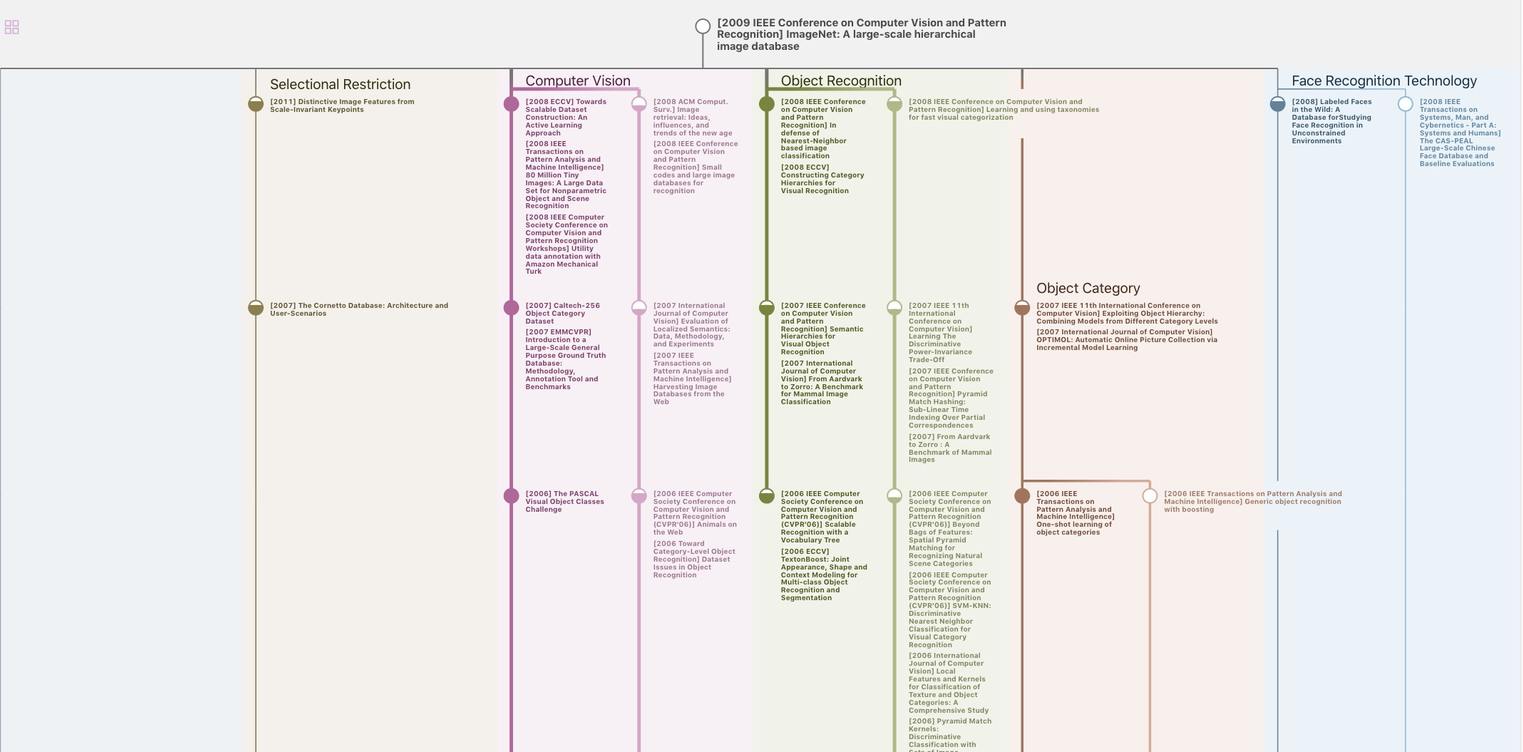
生成溯源树,研究论文发展脉络
Chat Paper
正在生成论文摘要