Robust feature selection using label enhancement and
user-61447a76e55422cecdaf7d19(2023)
摘要
High-dimensionality is the most noticeable characteristic of multilabel data. In practice, multilabel data typically contain complex noises. Ignoring these noises in the feature selection process tends to cause an inaccurate prediction. Besides, many existing multilabel feature selection methods assume that the relation between samples and relevant labels is crisp, and the difference information hiding in the label space is lost. Given all these, in this paper, soft labels are investigated by label enhancement in multilabel feature selection. A robust feature selection algorithm is proposed using label enhancement and multilabel β-precision fuzzy rough sets. With the perspective of data distribution from samples in the same and different class, the margin-based robust fuzzy neighborhood is first defined to construct the robust fuzzy granular space. Second, label enhancement strategy is given in the robust fuzzy granular space considering multilabel data distribution. To investigate the noise-tolerant model, the underlying structure of label space after label enhancement is employed to encode the score vector of samples, which is used to search the pseudo-different class's samples of target sample. Then, the multilabel β-precision fuzzy rough set model is built to deal with multilabel data. Moreover, the fuzzy approximation degree of knowledge and the fuzzy dependency of decision classes with respect to conditional features are fused to measure the significance of features. Finally, a robust heuristic multilabel feature selection algorithm is proposed. Extensive experiments on classification performance and anti-noise ability are conducted, which verify that the proposed algorithm is effective and robust.
更多查看译文
关键词
Fuzzy rough sets,Multilabel feature selection,Label enhancement,Robustness
AI 理解论文
溯源树
样例
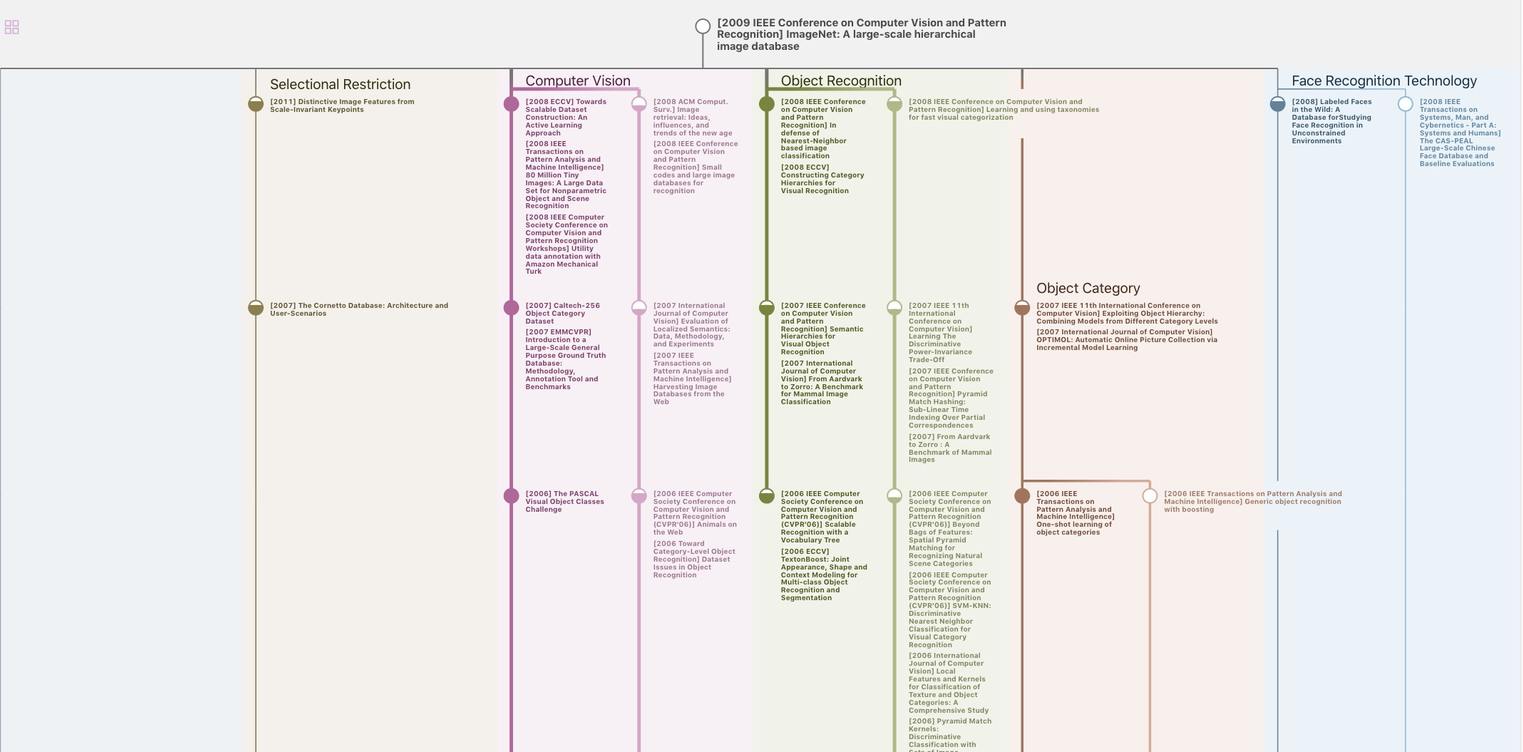
生成溯源树,研究论文发展脉络
Chat Paper
正在生成论文摘要