Large-Scale Quantum Separability Through a Reproducible Machine Learning Lens
CoRR(2023)
摘要
The quantum separability problem consists in deciding whether a bipartite density matrix is entangled or separable. In this work, we propose a machine learning pipeline for finding approximate solutions for this NP-hard problem in large-scale scenarios. We provide an efficient Frank-Wolfe-based algorithm to approximately seek the nearest separable density matrix and derive a systematic way for labeling density matrices as separable or entangled, allowing us to treat quantum separability as a classification problem. Our method is applicable to any two-qudit mixed states. Numerical experiments with quantum states of 3- and 7-dimensional qudits validate the efficiency of the proposed procedure, and demonstrate that it scales up to thousands of density matrices with a high quantum entanglement detection accuracy. This takes a step towards benchmarking quantum separability to support the development of more powerful entanglement detection techniques.
更多查看译文
关键词
quantum,reproducible machine learning
AI 理解论文
溯源树
样例
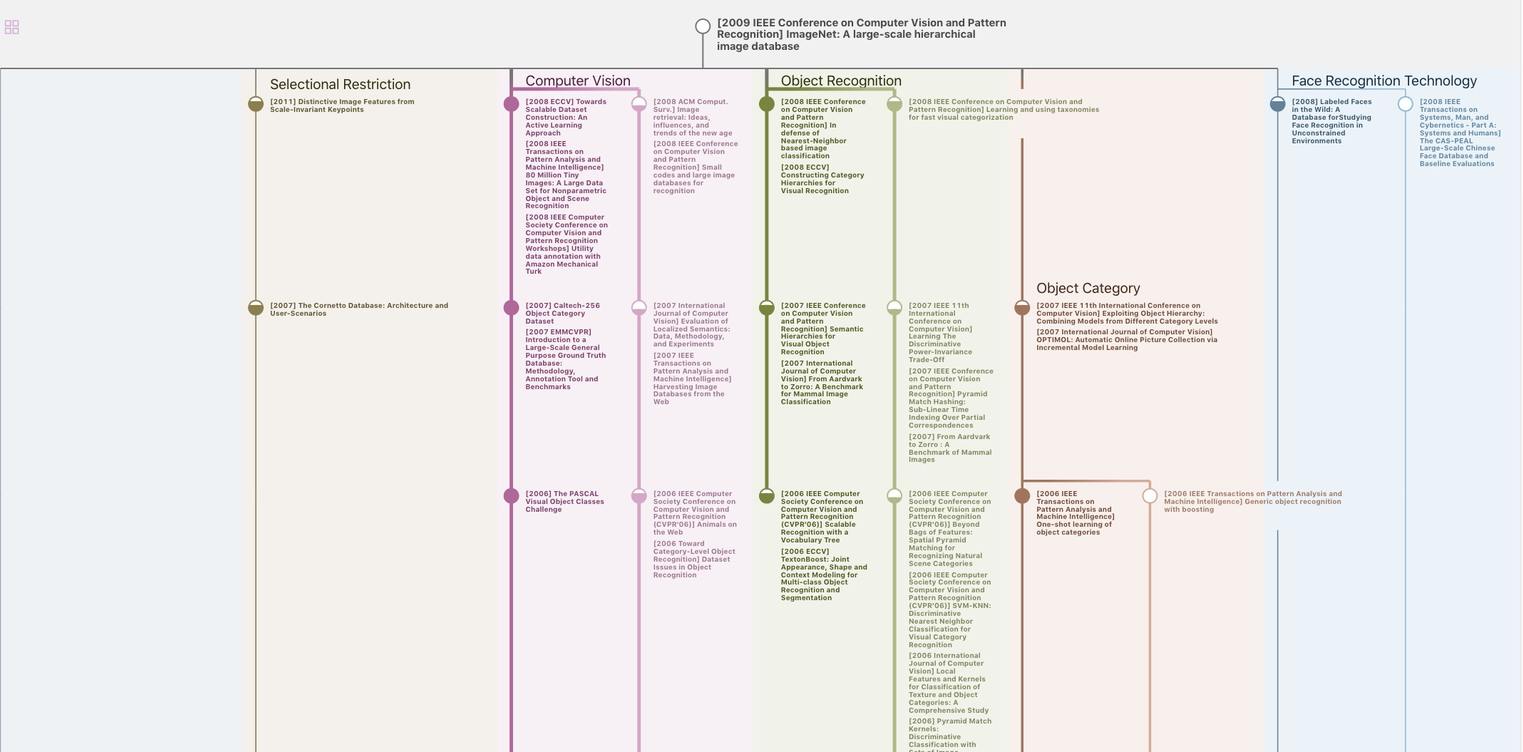
生成溯源树,研究论文发展脉络
Chat Paper
正在生成论文摘要