Clinical validation of a fully automated framework for ventricular segmentation and wall motion classification from cine CMR
Research Square (Research Square)(2022)
摘要
Abstract Background We present the clinical validation of an AI-based framework for automatic ventricular segmentation and quantification, and automatic wall motion classification from cine CMR. Methods The framework was evaluated using two datasets, the SCMR Consensus dataset and a cohort private dataset composed by 146 patients. The automatic segmentation of left ventricle (LV) of SCMR Consensus dataset was evaluated through Dice Similarity Coefficient (DSC). The ventricular function quantification was assessed by processing both datasets, measuring LV and right ventricle (RV) end-diastolic and end-systolic volumes, ejection fraction (EF), and left ventricle mass (LVM). The validation was based on agreement indices, such as the intra-class correlation coefficients (ICC). Automatic wall motion classification was performed using the cohort dataset, and assessed using confusion matrix metrics. Results The comparison between the proposed framework and experts' manual performances on ventricular segmentation and function quantification processes showed high levels of contouring overlap (DSC=0.72), and agreement (ICC > 0.96 and ICC > 0.81 for volumes and EFs of LV and RV, respectively, and ICC > 0.78 for LVM). The automatic wall motion analysis obtained a classification accuracy of 0.77, with a sensitivity value of 1.00, meaning no false negative.. Conclusions The validation results confirmed the proposed framework robustness and generalization capabilities. Moreover, our proposal demonstrated its potential for automatic wall motion classification in clinical practice.
更多查看译文
关键词
ventricular segmentation,wall motion classification,clinical validation
AI 理解论文
溯源树
样例
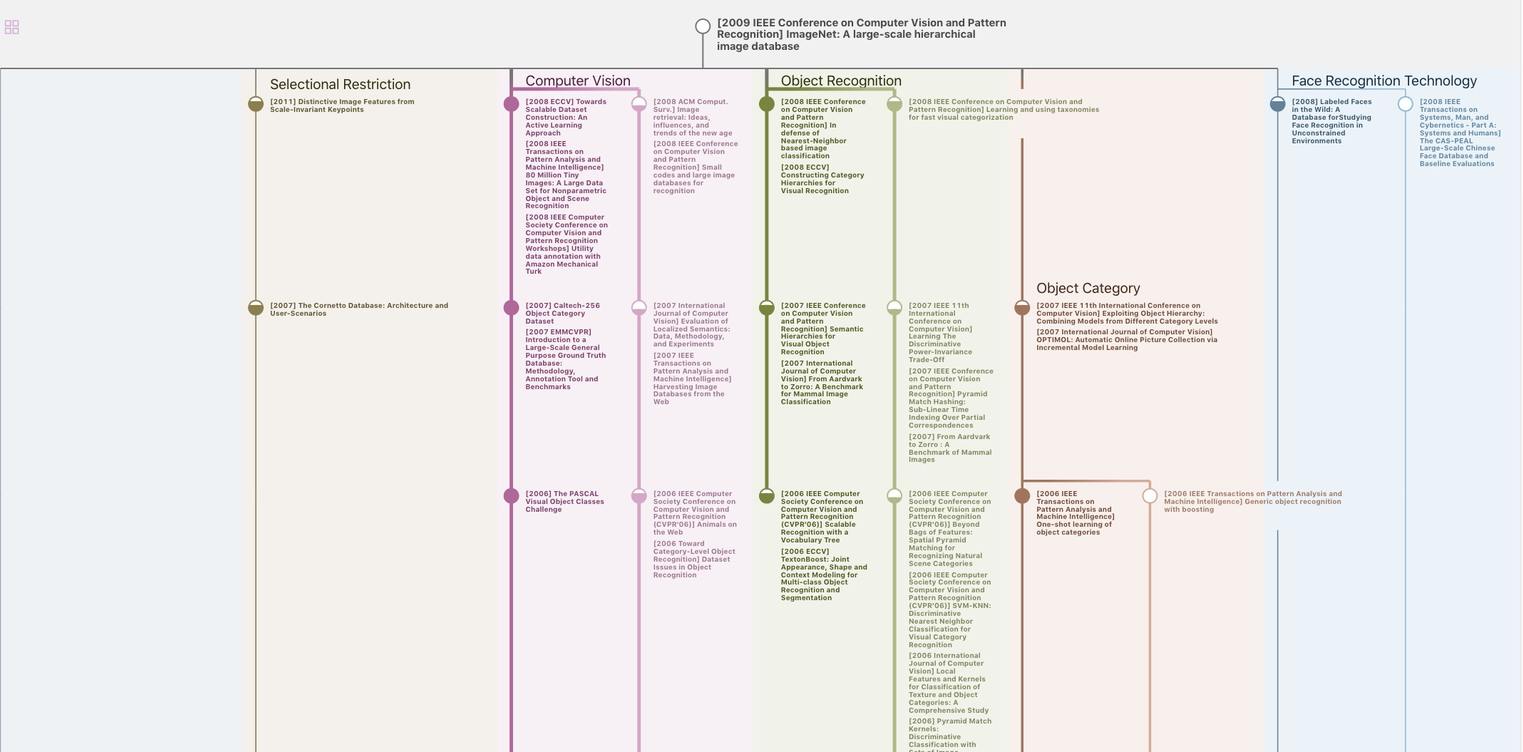
生成溯源树,研究论文发展脉络
Chat Paper
正在生成论文摘要