Online broad learning system for dynamic data flow
Third International Conference on Artificial Intelligence and Electromechanical Automation (AIEA 2022)(2022)
摘要
Dynamic data flow is a data model with the characteristics of high speed, high dimensionality, change, and potential infinity, and its data distribution evolves over time. Broad Learning System (BLS) is a learning model with fast training speed, simple structure and high accuracy. It has the characteristics of online learning and rapid adjustment of the model structure. The traditional online BLS algorithm cannot capture the changing trend of the dynamic data flow with time in time, so it is not suitable for dealing with the dynamic data flow problem. This paper proposes a novel online broad learning algorithm (MDO-BLS) that combines sliding window strategy and forgetting factor method. This BLS model has a forgetting mechanism, that is, the model has the ability to learn new samples online while forgetting the knowledge of old samples, so that it can quickly capture the trend of data changes. To verify the performance of the proposed BLS model with forgetting mechanism, experiments are performed using a dynamic regression dataset. The experimental results show that the BLS model with forgetting mechanism is superior to the traditional BLS and some other online algorithm in both prediction accuracy and time overhead, so it is more suitable for application in dynamic data flow environment.
更多查看译文
关键词
online broad learning system,flow,data
AI 理解论文
溯源树
样例
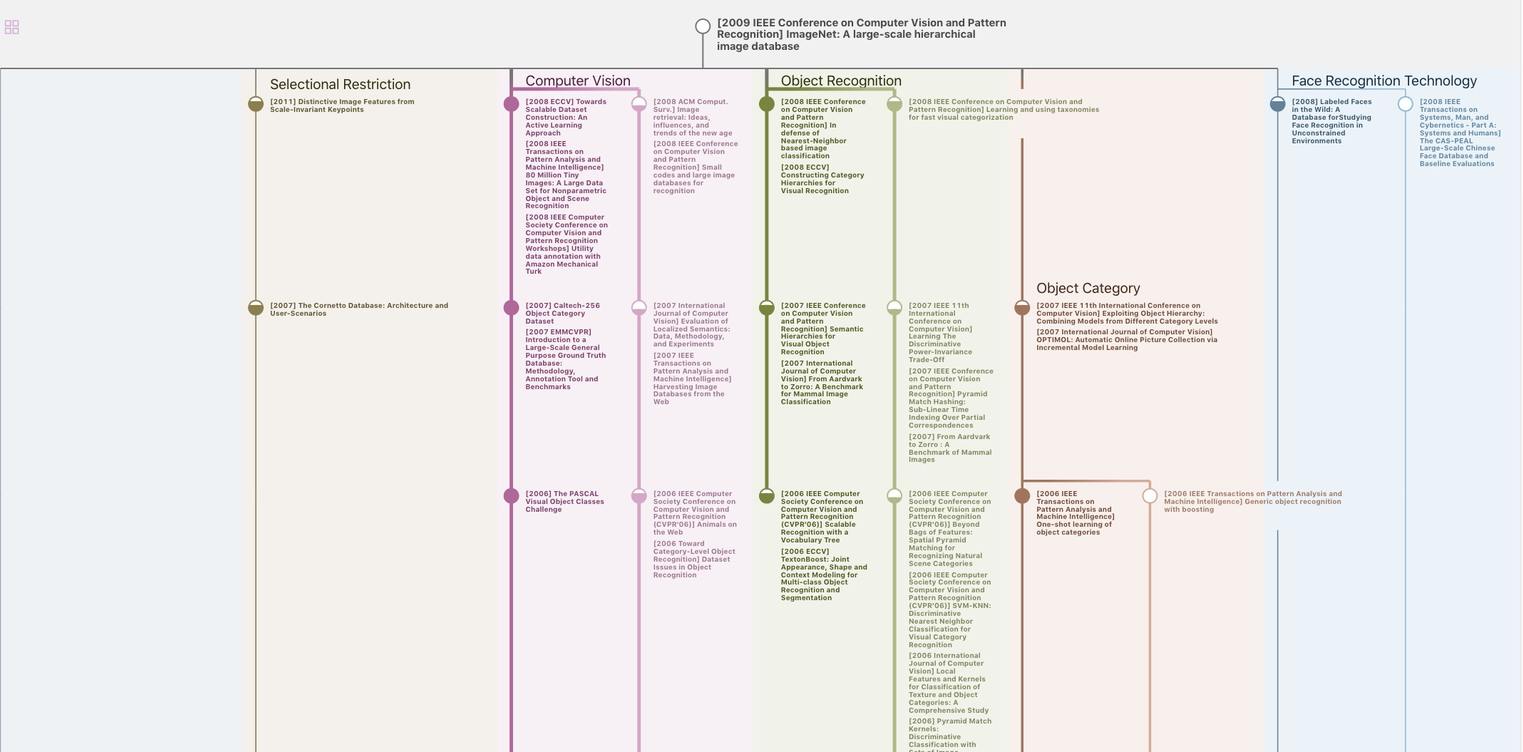
生成溯源树,研究论文发展脉络
Chat Paper
正在生成论文摘要