The Effect of Text Data Augmentation Methods and Strategies in Classification Tasks of Unstructured Medical Notes
Research Square (Research Square)(2022)
摘要
Abstract Background Text classification tasks of unstructured medical notes are often challenged with the issues of highly imbalanced classes and/or small sample sizes. Data augmentation is a common approach to mitigate the impact of these issues and enhance model performance. However, not all augmentation methods improve model performance, and an uninformed and arbitrary choice of augmentation methods may hurt model performance instead. In addition, the widely used strategy of augmenting until balanced may not always work the best. Methods In this paper, we investigated the effect of 20 different augmentation methods and several different augmentation strategies in 16 classification tasks. The 16 classification tasks were divided into 4 groups based on their disease prevalence, and different augmentation strategies and the 20 augmentation methods were applied to different groups. The Transformer Encoder model was run in all tasks for each of the 20 augmentation methods and the strategies, and then their model performance was compared against each other and against that without augmentation. Results Our results show that in addition to being a fast augmenter, the Splitting Augmenter consistently improved the model performance in terms of AUC-ROC and F1 Score in all strategies for most tasks. For highly imbalanced tasks, the strategy that augments the minority class until balanced, improved model performance by the largest margin. For other tasks, the best performing strategy was the one that augments the minority class until balanced and then augments both classes by an additional 10%. The largest improvement was 0.13 in F1 score and an impressive 0.34 in AUC-ROC, and both were produced by the Splitting Augmenter in the strategy that augments the minority class until balanced. Conclusions Different text data augmentation methods have different effects on the model performance. Some enhance model performance, and others yield no improvement or even have an adverse impact. With the right choice of augmentation methods, the model performance can be substantially improved. For the highly imbalanced tasks, the strategy that augments the minority class until balanced yielded the largest improvement. For other tasks, the strategy that keeps augmenting both classes by an additional 10% after reaching balance enhanced model performance further.
更多查看译文
关键词
text data augmentation methods,notes,classification tasks
AI 理解论文
溯源树
样例
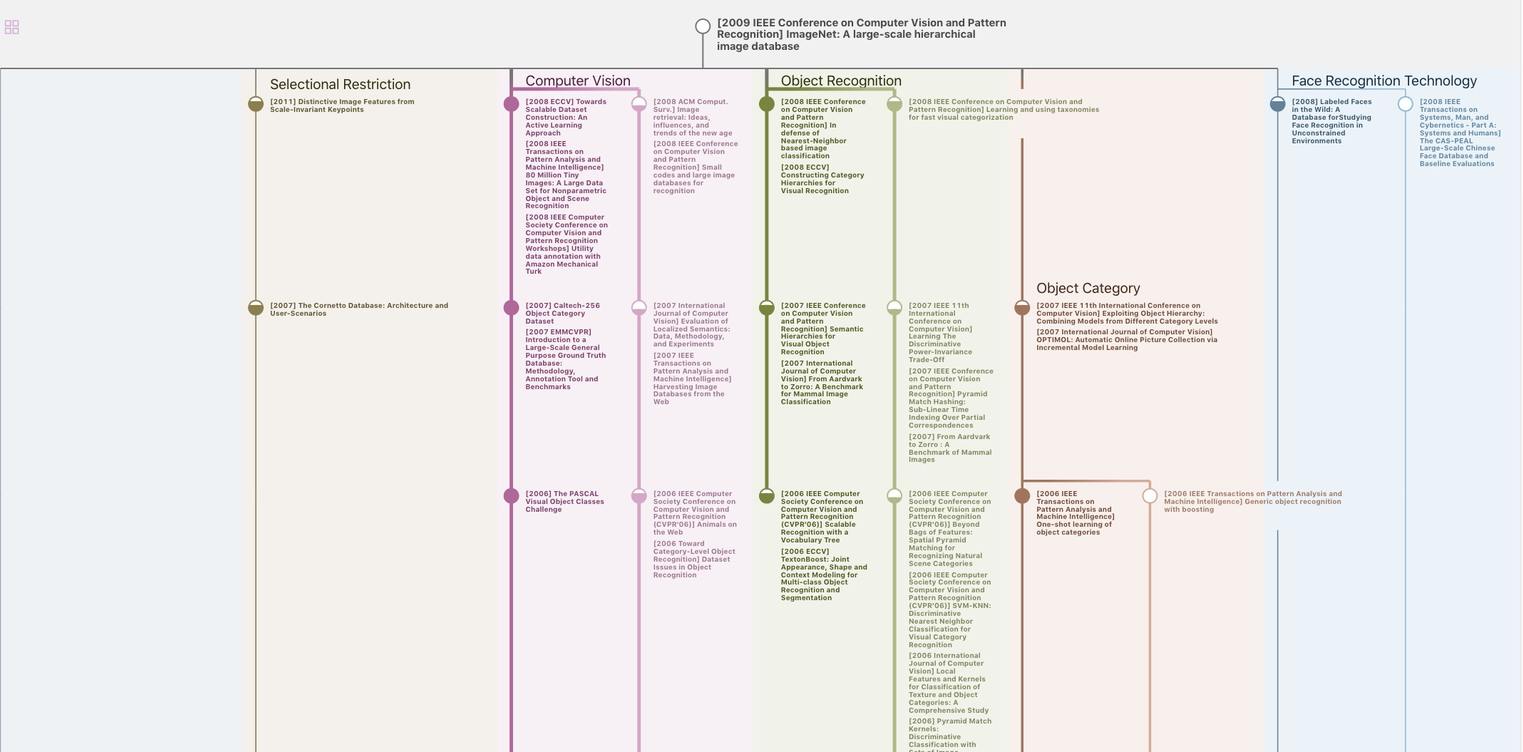
生成溯源树,研究论文发展脉络
Chat Paper
正在生成论文摘要