Prototype-Based Intent Perception
IEEE TRANSACTIONS ON MULTIMEDIA(2023)
摘要
Intent perception is a novel task that aims to understand the intention of images, regular classification methods usually perform unsatisfactorily on intent perception due to the semantic ambiguity problem, i.e. the intra-class variety problem in which images of the same intent class may contain objects of different semantic categories and the inter-class confusion problem in which images of different intent classes may contain objects of similar semantic categories. To address this problem, this paper introduces prototype learning into the intent perception and proposes a unified framework named PIP-Net to reduce the influence of semantic ambiguity. Specifically, for each intent class, we first filter semantic ambiguity samples which are far away from the cluster center. Then we use features of the filtered samples to generate prototypes via clustering algorithm. Besides, we enhance the diversity between prototypes of different classes to better handle the inter-class confusion problem. To update the prototypes in the training process, we introduce a global matching algorithm to holistically match each feature with class prototypes, and use the momentum update strategy to stably update prototypes. Experimental results on the Intentonomy dataset demonstrate that our method can consistently outperform the traditional classification paradigm in multiple baseline models, and verify the effectiveness of our proposed prototype learning paradigm in addressing the intent perception problem. Our proposed PIP-Net achieves a new state-of-the-art performance on Intentonomy, including Macro F1 score of 31.57% and averaging F1 score of 41.85%.
更多查看译文
关键词
Prototypes,Semantics,Task analysis,Feature extraction,Training,Clustering algorithms,Animals,Intent perception,semantic ambiguity,prototype learning,cluster
AI 理解论文
溯源树
样例
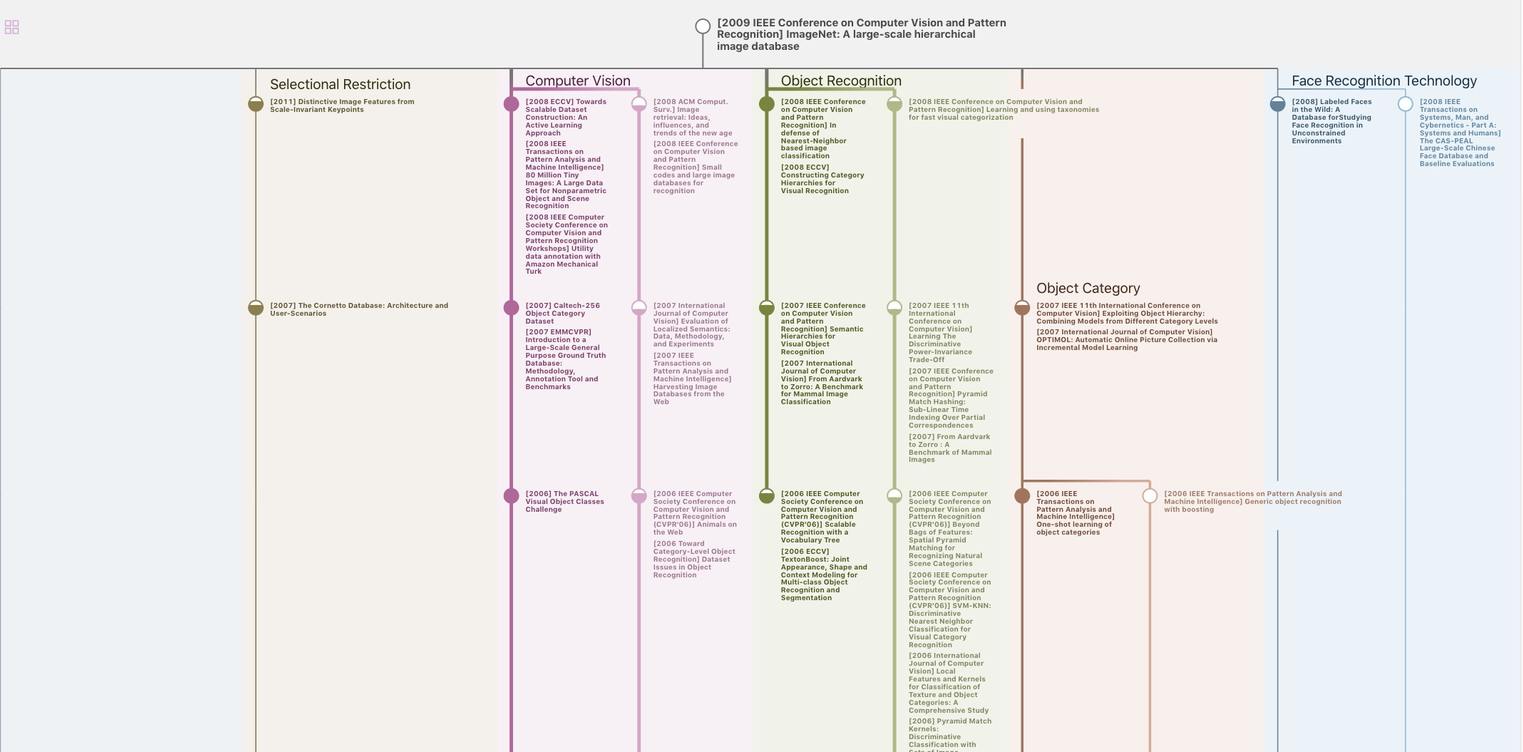
生成溯源树,研究论文发展脉络
Chat Paper
正在生成论文摘要