A Compound Data Poisoning Technique with Significant Adversarial Effects on Transformer-based Text Classification Tasks
Research Square (Research Square)(2023)
摘要
Abstract Transformer-based models have demonstrated much success in various natural language processing (NLP) tasks. However, they are often vulnerable to adversarial attacks, such as data poisoning, that can intentionally fool the model into generating incorrect results. In this paper, we present a novel, compound variant of a data poisoning attack on a transformer-based model that maximizes the poisoning effect while minimizing the scope of poisoning. We do so by combining the established data poisoning technique (label flipping) with a novel adversarial artifact selection and insertion technique aimed at minimizing detectability and the scope of the poisoning footprint. We find that using a combination of these two techniques, we achieve a state-of-the-art attack success rate (ASR) of ~90% while poisoning only 0.5% of the original training set, thus minimizing the scope and detectability of the poisoning action.
更多查看译文
关键词
compound data poisoning technique,significant adversarial effects,text classification tasks,transformer-based
AI 理解论文
溯源树
样例
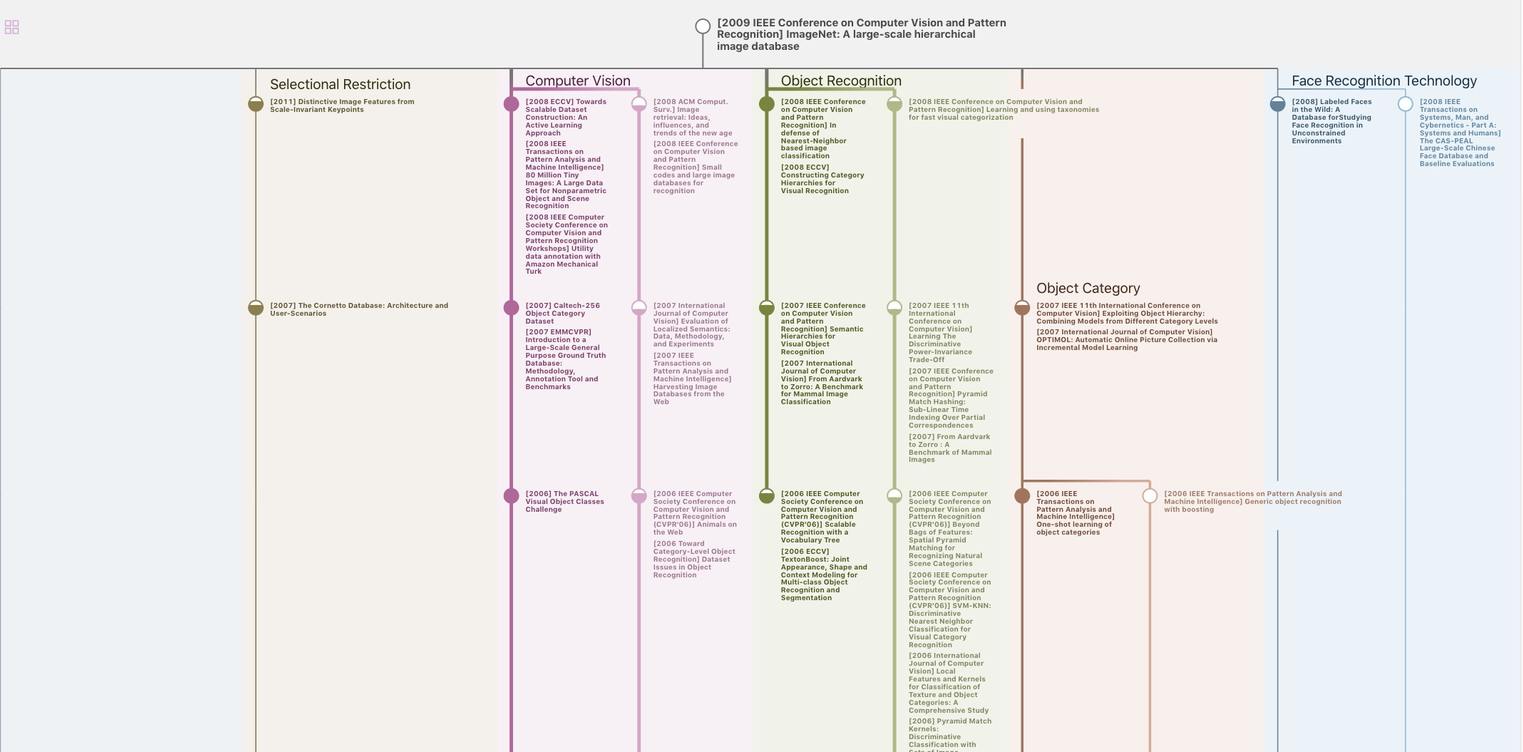
生成溯源树,研究论文发展脉络
Chat Paper
正在生成论文摘要