Model-Averaged Bayesian t-Tests
crossref(2022)
摘要
One of the most common statistical analyses in experimental psychology concerns the comparison of two means using the frequentist t-test. However, frequentist t-tests do not quantify evidence and require various assumption tests. Recently popularized Bayesian t-tests do quantify evidence, but these were developed for scenarios where the two populations are assumed to have the same variance. As an alternative to both methods we outline a comprehensive t-test framework based on Bayesian model-averaging. This new t-test framework simultaneously takes into account models that assume equal and unequal variances, and models that use t-likelihoods to improve robustness to outliers. The resulting inference is based on a weighted average across the entire model ensemble, with higher weights assigned to models that predicted the observed data well. This new t-test framework provides an integrated approach to assumption checks and inference by applying a series of pertinent models to the data simultaneously rather than sequentially. The integrated Bayesian model-averaged t-tests achieve robustness without having to commit to a single model following a series of assumption checks. To facilitate practical application we provide user-friendly implementations via the RoBTT package in R.
更多查看译文
AI 理解论文
溯源树
样例
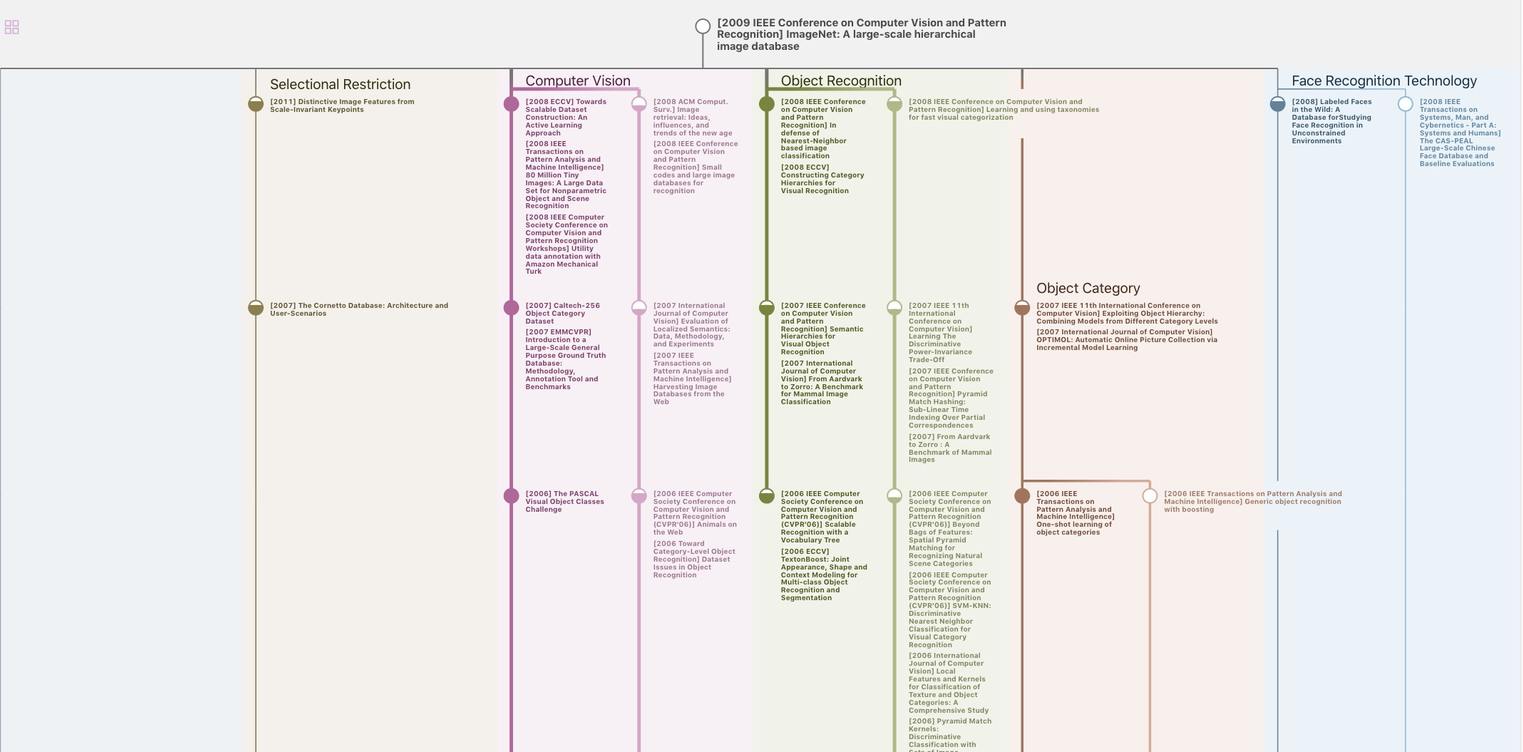
生成溯源树,研究论文发展脉络
Chat Paper
正在生成论文摘要