InFocus: Amplifying Critical Feature Influence on Non-Intrusive Load Monitoring Through Self-Attention Mechanisms.
IEEE Transactions on Smart Grid(2023)
摘要
Non-intrusive load monitoring (NILM) enables extracting individual appliances power consumption data from an aggregated power signal in a cost-effective way. The extracted appliance-level power data can greatly facilitate tasks such as malfunction diagnosis and load forecasting, which are of significant importance for efficient energy use. Various neural networks including convolutional neural network (CNN), recurrent neural network (RNN), and transformer (self-attention-based neural network) are employed in the design of NILM solutions since 2015. In particular, CNN is revealed to extract certain critical features such as power level and typical usage duration, thus, achieving superior performance. However, the global features especially the dependency correlations between different positions in a sequence cannot be properly acquired. Accordingly, we devise a novel model incorporating an added attention layer to overcome this limitation. The added self-attention mechanism can automatically assign attention scores/weights to different features outputted by convolutional layers, which amplifies the positive influence of critical knowledge while realizing global reference. Moreover, this model can explicitly extract the appliance s multi-state information, which endows the model with more interpretability. We further improve our model by substituting the added self-attention mechanism with a lightweight one, which decreases the number of model parameters while maintaining the decomposing accuracy. Experimental results over two real-world datasets, REDD and UK-DALE, demonstrate that our models outperform the state-of-the-art, achieving 6.5 %-52 % improvement on average for three standard evaluation metrics. Moreover, we offer an extracted NILM solution system architecture incorporating neural networks, aiming to establish a framework to support future research.
更多查看译文
关键词
Non-intrusive load monitoring,energy disaggregation,dual-convolutional neural network,self-attention
AI 理解论文
溯源树
样例
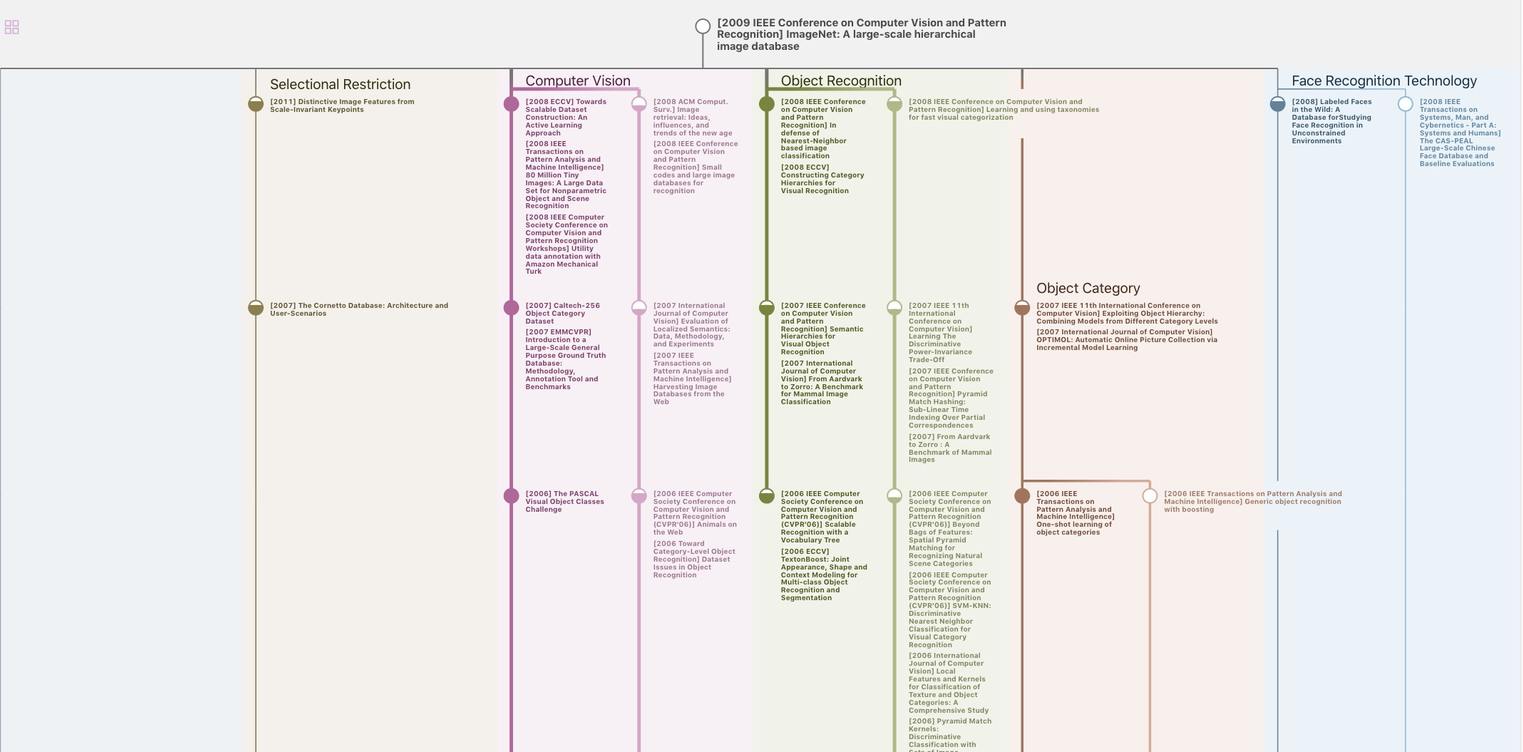
生成溯源树,研究论文发展脉络
Chat Paper
正在生成论文摘要