Assessing daily PM2.5 at every square kilometer of India over 2008-2020 using a machine learning framework
ISEE Conference Abstracts(2022)
摘要
BACKGROUND AND AIM: High levels of ambient particulate matter (PM) combined with sparse ground monitoring networks, specifically concentrated in urban areas in India, pose massive challenges to studying health effects of PM in India. Thus it is important to develop robust predictive models that provide exposure estimates at high spatiotemporal resolution across India. METHODS: We used a machine-learning-based approach by ensemble averaging across four different learners to develop a model calibrated against ground-monitoring-based data from 1060 stations spanning 2008-2020. To counter the sparsity of available PM2.5, we also implemented a calibration model for the ratio of PM2.5 and PM10. Predictors encompassing all major areas such as, meteorology, land-use patterns, satellite observations, emissions inventories and chemical transport models were utilized. Overall cross-validation(CV) was conducted by leaving out monitors and performance was assessed at the daily level using R2, RMSE, bias and slope. RESULTS: In the left-out 20% validation dataset, median estimated PM2.5 using ensemble averaging was 44.5 µg/m3 compared to the observed concentration of 45 µg/m3. The overall daily CV-R2 was 0.84 ranging between 0.73-0.92 across years, while daily RMSE ranged between 3.7-4.2 µg/m3. Among the learners, tree-based algorithms (gradient boosting, extreme gradient boosting and random forests) performed better than deeplearning, and ensemble averaging further improved the predictive performance. Among predictors, meteorology, reanalysis based PM2.5 and elevation were more predictive by the tree based learners while urban-rural classification and road density were high-rank predictors by the deeplearning algorithm. CONCLUSIONS: We have developed a valuable resource for air pollution epidemiology in a LMIC scenario with both high levels of pollution and burden of disease. The resulting predictions can leverage existing, ongoing and future health studies all across India to accurately assess the burden of ambient PM2.5 on a multitude of health outcomes. KEYWORDS Particulate Matter, Machine learning, Ensemble averaging, India, Spatiotemporal
更多查看译文
关键词
machine learning,india,square kilometer
AI 理解论文
溯源树
样例
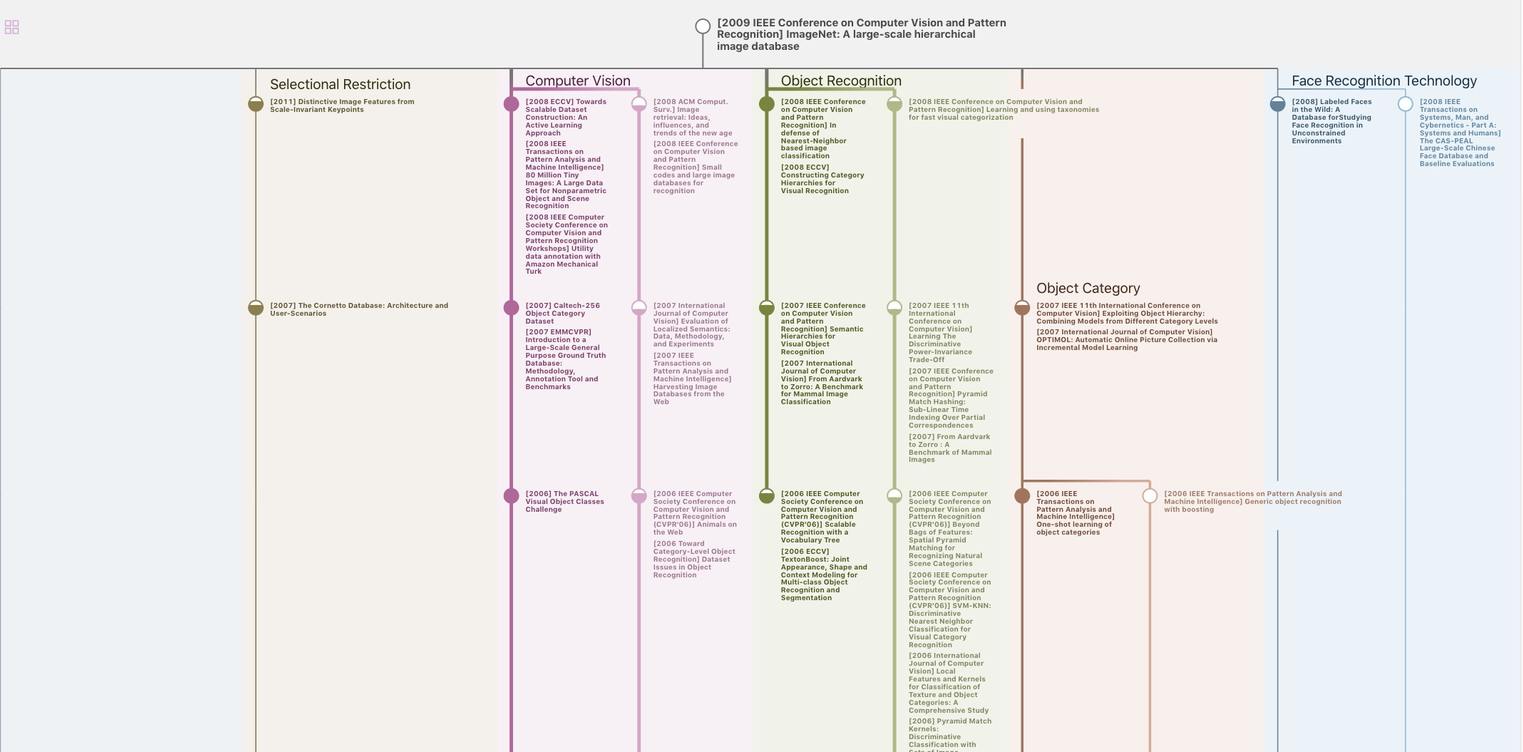
生成溯源树,研究论文发展脉络
Chat Paper
正在生成论文摘要