Deep learning predicts patients’ outcome and mutations from H&E slides in Gastrointestinal stromal tumor (GIST)
crossref(2022)
摘要
Abstract Background. Most gastrointestinal stromal tumors (GIST) have activating mutations of KITor PDGFRA genes and imatinib has proven effective in palliative and adjuvant situations. Risk assessment according to the AFIP/Miettinen classification and mutational profiling are major tools for management of patients with GIST. However, the AFIP/Miettinen classification depends heavily on mitotic counts, which is laborious and sometimes inconsistent between pathologists. It has also been shown to be imperfect in stratifying patients. Molecular testing is costly and time consuming, therefore not systematically performed in all countries. New methods to improve risk and molecular predictions are hence crucial to improve the tailoring of adjuvant therapy. Methods. We have built deep learning (DL) models on digitized H&E-stained whole slide images (WSI) to predict patients’ outcome and mutations. Models were trained with a cohort of 1233 GIST and validated on an independent cohort of 286 GIST. All WSIs from center 1 are available under controlled access at the European Genome-phenome Archive via accession number X(process is ongoing, will be completed at publication). Results. DL models outperformed the Miettinen classification for relapse-free-survival prediction in localized GIST without adjuvant Imatinib (C-index=0.83 in testing and 0.72 for independent validation). DL splitted Miettinen intermediate risk GIST into high/low risk groups(p-value=2.1e-03). DL models achieved an area under the receiver operating characteristic curve (AUC) of 0.81, 0.91 and 0.71 for predicting mutations in KIT, PDGFRA and wild type respectively in testing and 0.84, 0.93 and 0.56 in independent validation. Notably, PDGFRA exon18 D842V mutation which is resistant to Imatinib, was predicted with an AUC of 0.87 and 0.90 in testing and independent validation, respectively. Additionally, novel histological criteria predictive of patients’ outcome and mutations were identified by reviewing the tiles selected by the models. Conclusions. Our results strongly suggest that implementing DL with digitized WSI may represent a reproducible way to improve tailoring therapy and precision medicine for patients with GIST.
更多查看译文
AI 理解论文
溯源树
样例
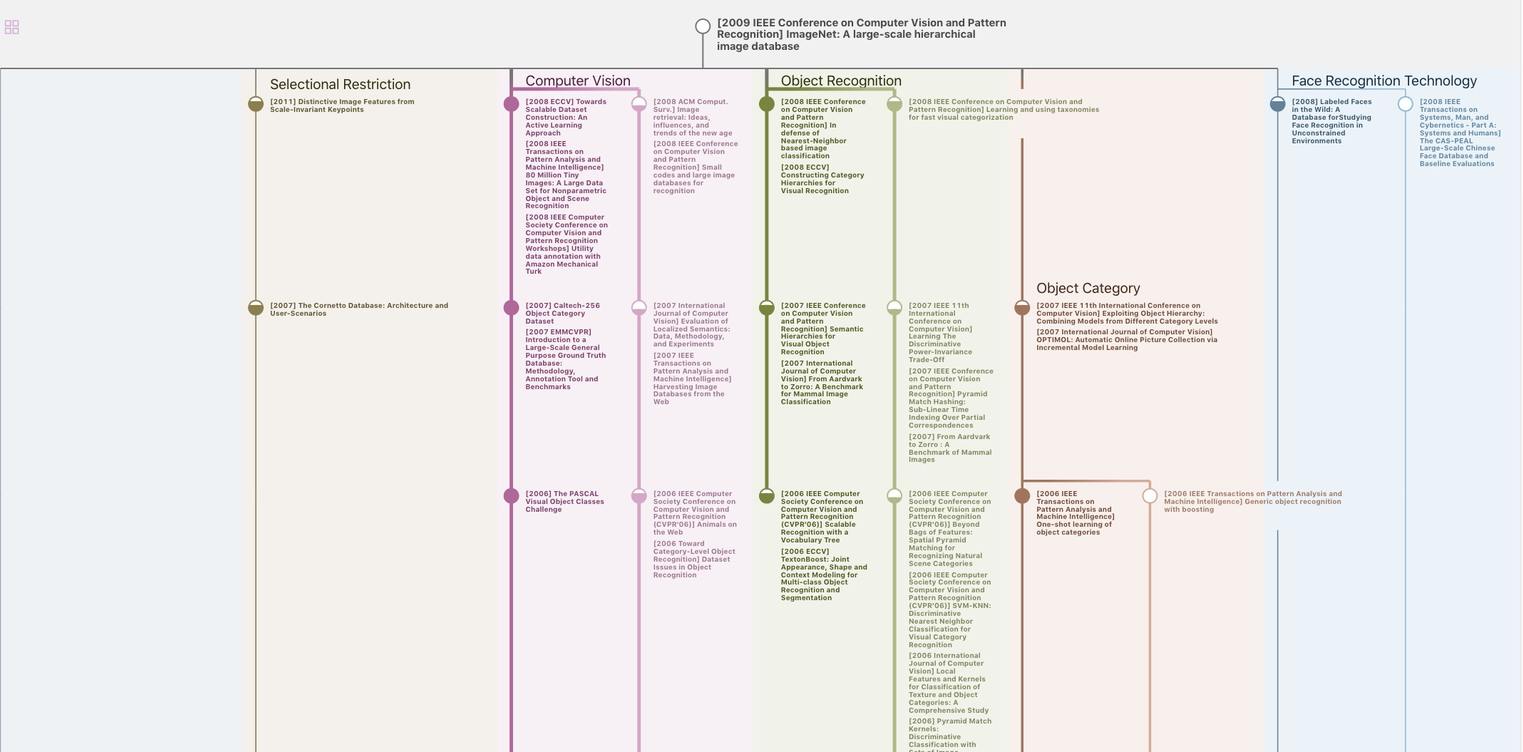
生成溯源树,研究论文发展脉络
Chat Paper
正在生成论文摘要