Learning-Based Cloud Server Configuration for Energy Minimization under Reliability Constraint.
IEEE transactions on reliability(2024)
摘要
Cloud computing has attracted wide attention from both academia and industry, since it can provide flexible and on-demand hardware and software resources as services. Energy consumption of cloud servers is the main concern of cloud service providers since reducing energy consumption can bring them a lower operation cost (and hence a higher profit) and alleviate carbon footprints to the environment. Typically, the common power management techniques for enhancing energy efficiency would make cloud servers more vulnerable to soft errors and hence adversely impact the quality of services. Thus, reliability cannot be ignored in the design of methodologies for improving the energy efficiency of cloud servers. In this article, we aim to minimize the energy consumption of cloud servers under the soft-error reliability constraint by configuring the size and speed of servers. Specifically, we first derive the expected reliability based energy consumption of cloud servers to formulate the reliability-constrained energy minimization problem. We then leverage the reinforcement learning technique to obtain an optimal server configuration solution that maximizes system energy efficiency while maintaining the system reliability constraint. Finally, we perform extensive simulation experiments to analyze the relationship between system energy consumption and server configuration under varying arrival rates and execution requirements of service requests. Comparative experiments are also performed to validate the efficacy of the proposed learning-based server configuration scheme. Results show that compared to a benchmark method, the energy saved by the proposed scheme can reach up to 31.5%.
更多查看译文
关键词
Servers,Cloud computing,Reliability,Energy consumption,Quality of service,Transient analysis,Task analysis,Cloud service,energy efficiency,multiserver,reinforcement learning,reliability
AI 理解论文
溯源树
样例
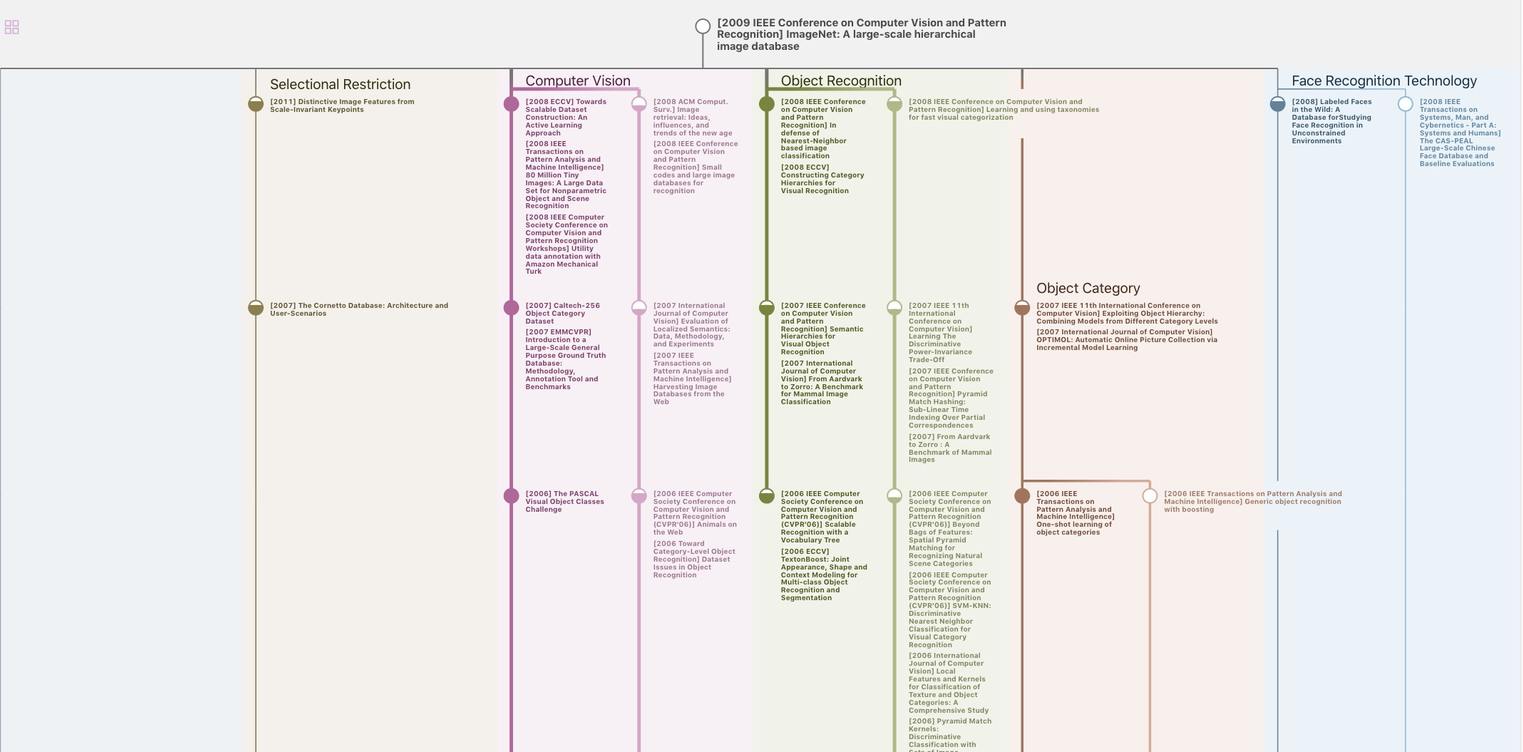
生成溯源树,研究论文发展脉络
Chat Paper
正在生成论文摘要