Looking back to the future: Unpacking the first nine months of the novel COVID-19 infodemic on Twitter with a content analysis (Preprint)
crossref(2023)
摘要
UNSTRUCTURED This study analyzed 25,018,086 tweets posted during the initial nine months of the COVID-19 pandemic between March to November 2020. Using a mixed method of automated (i.e., LDA topic model) and manual content analyses, results from this study point to debatable information as the most prevalent and diffused information category followed by credible information, general disinformation, trolling, biased or one-sided and conspiratorial disinformation. Contrary to mainstream understanding, the prevalence of credible information was double that of disinformation. Overall, General Users were the most prolific category of users (53.0%) involved in the spread of COVID-19 related disinformation. News media organizations (16.50%); Writers, Podcasters, Journalists or Media Personalities (9.93%), and Organizations such as Non-Profits, Pharmaceutical Manufacturers, Medical groups, and Businesses (6.64%); Researchers, Scholars, Scientists or Healthcare Professionals (6.09%) were among the top five categories of users tweeting COVID-19 disinformation on Twitter. Politicization of public health was an inherent trait within topics and targets associated with disinformation narratives. Debatable information also warrants further attention, resources, and remediation as the most diffused form of information during the initial nine months of the COVID-19 pandemic on Twitter. Considering the shaping and diffusion of disinformation narratives involved a participatory process, so too must the strategies that respond to this issue. Relevant stakeholders must adopt a whole-of-society lens when devising measures to ensure a healthy information ecosystem on Twitter and other similar social media platforms. They must also address politicization of public health, acknowledge, and clearly communicate scientific and situational uncertainty associated with novel or recurring infectious disease crises and emergencies such as the the COVID-19 pandemic.
更多查看译文
AI 理解论文
溯源树
样例
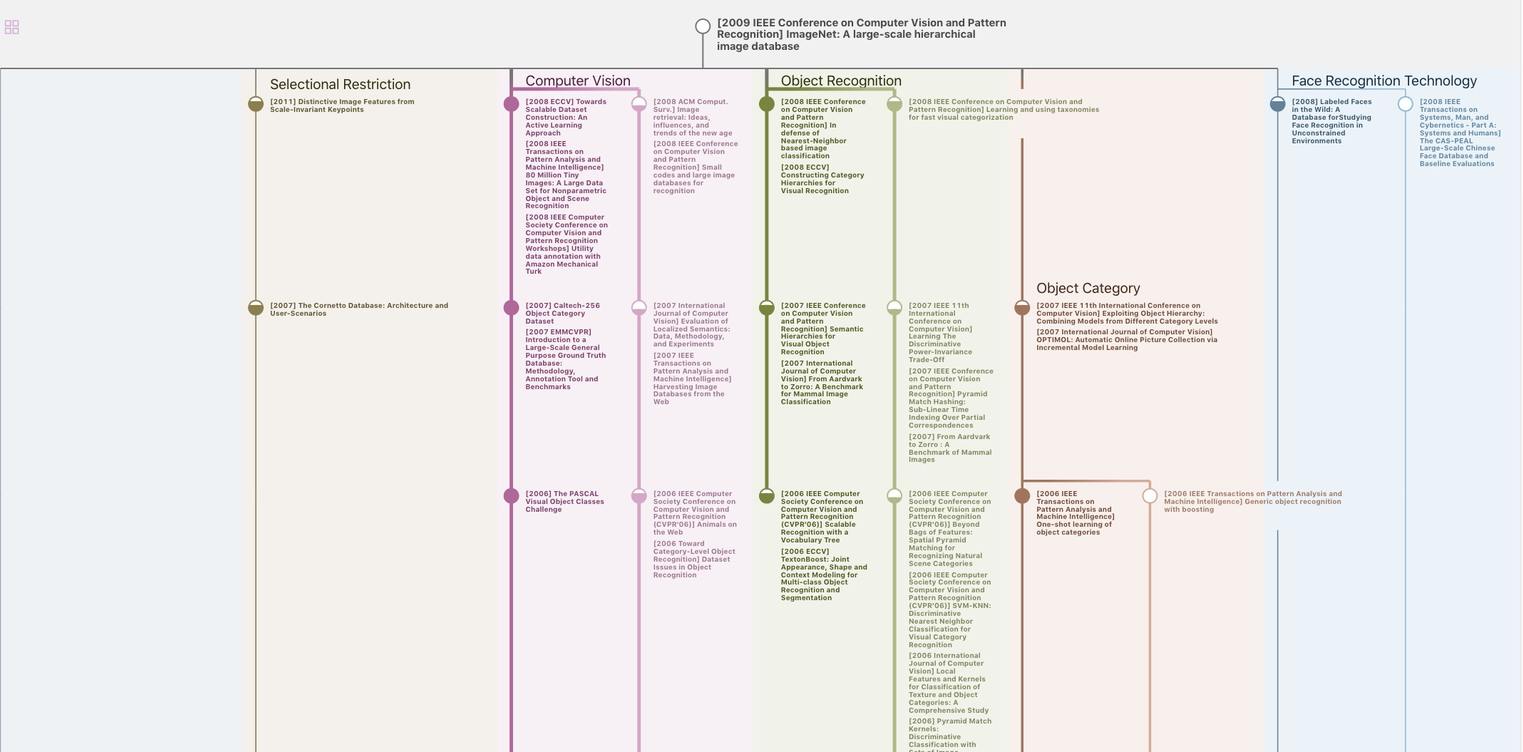
生成溯源树,研究论文发展脉络
Chat Paper
正在生成论文摘要