FDD: The Data Pipeline for Steel Defect Detectors
Research Square (Research Square)(2022)
摘要
Abstract Surface defects are a common issue that affects product quality in the industrial manufacturing process. Many companies put a lot of effort into developing automated inspection systems to handle this issue. In this work, we propose a novel deep learning-based surface defect inspection system called the forceful steel defect detector (FDD), especially for steel surface defect detection. Our model adopts the state-of-the-art cascade R-CNN as the baseline architecture, and improves it with the deformable convolution and the deformable RoI pooling to adapt to the geometric shape of defects. Besides, our model adopts the guided anchoring region proposal to generate bounding boxes with higher accuracies. Moreover, to enrich the point-of-view of input images, we propose the random scaling and the ultimate scaling techniques in the training and inference process, respectively. The experimental studies on the Severstal steel dataset, NEU steel dataset, and DAGM dataset demonstrate that our proposed model effectively improved the detection accuracy in terms of the average recall (AR) and the mean average precision (mAP) compared to state-of-the-art defect detection methods. We expect our innovation to accelerate the automation of the industrial manufacturing process by increasing productivity and by sustaining high product qualities.
更多查看译文
关键词
data pipeline,steel
AI 理解论文
溯源树
样例
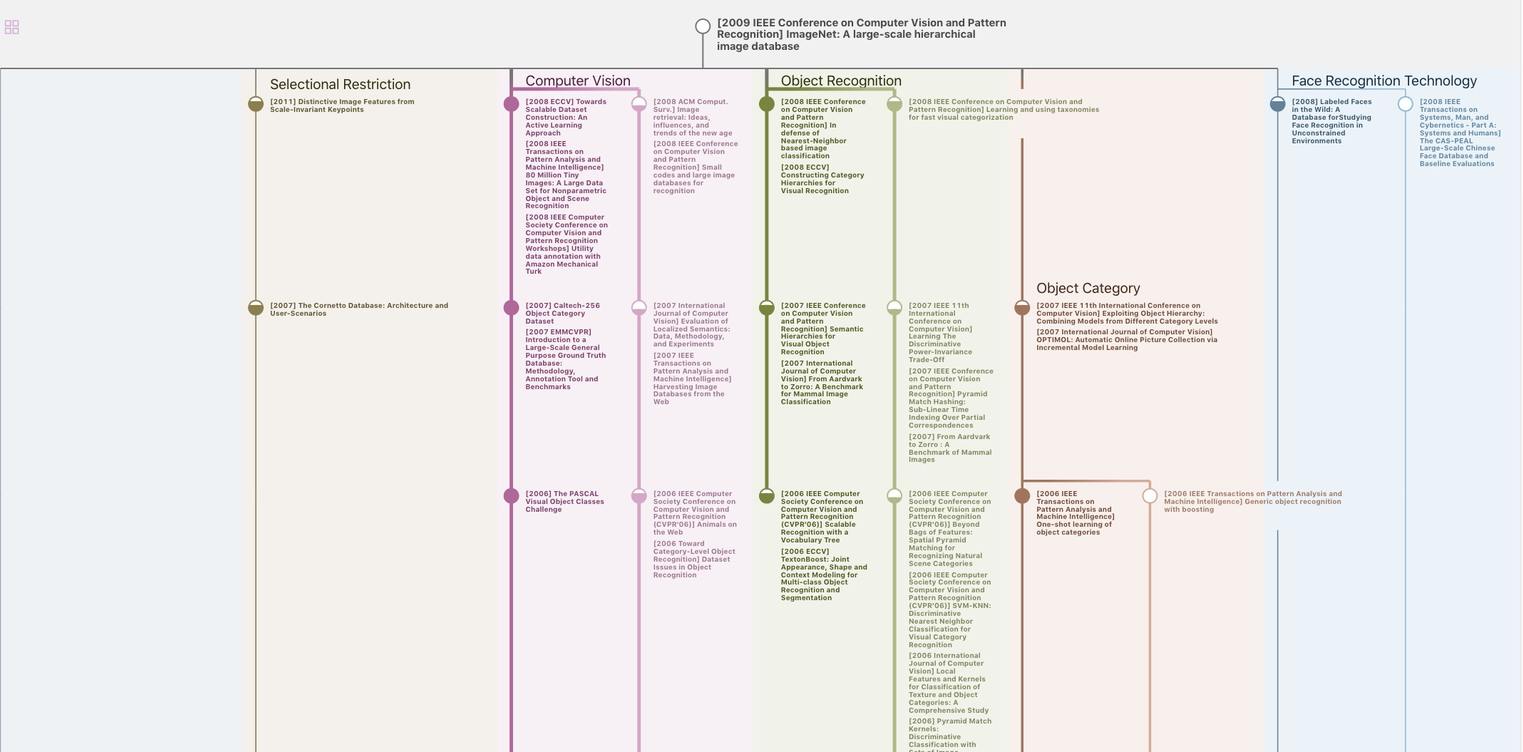
生成溯源树,研究论文发展脉络
Chat Paper
正在生成论文摘要