GANSim-surrogate: An integrated framework for conditional geomodelling and uncertainty analysis
EarthArXiv (California Digital Library)(2022)
摘要
We propose a deep-learning framework (GANSim-surrogate) for conditioning subsurface geomodel realizations to static data and dynamic flow data. The static data includes well facies data, interpreted facies probability maps, and non-spatial global features, while dynamic data can include well data such as pressures and flow rates. The framework consists of a Convolutional Neural Network (CNN) generator trained from GANSim (a Generative Adversarial Network-based geomodelling simulation approach), a CNN-based surrogate, and options for searching appropriate input latent vectors for the generator. The four search methods investigated are Markov Chain Monte Carlo, Iterative Ensemble Smoother, gradient descent, and gradual deformation. The framework is validated by applying it for geomodelling of channelized reservoirs. First, a generator is trained using GANSim to generate geologic facies models; in addition, a flow simulation surrogate is trained using a physics-informed approach. Then, given well facies data, facies probability maps, global facies proportions, and dynamic bottomhole pressure data (BHP), the trained generator takes the first three conditioning data and a latent vector as inputs and produces a random realistic facies model conditioned to the static data. To condition to the dynamic data, the produced facies model is converted to permeabilities and mapped to BHP data by the trained physics-informed surrogate. Finally, the mismatch between the surrogate-produced and the observed BHP data is minimized to obtain appropriate input latent vectors for the generator. The framework is computationally efficient, and the posterior facies models prove to be realistic and consistent with all of the conditioning data.
更多查看译文
关键词
conditional geomodelling,uncertainty,gansim-surrogate
AI 理解论文
溯源树
样例
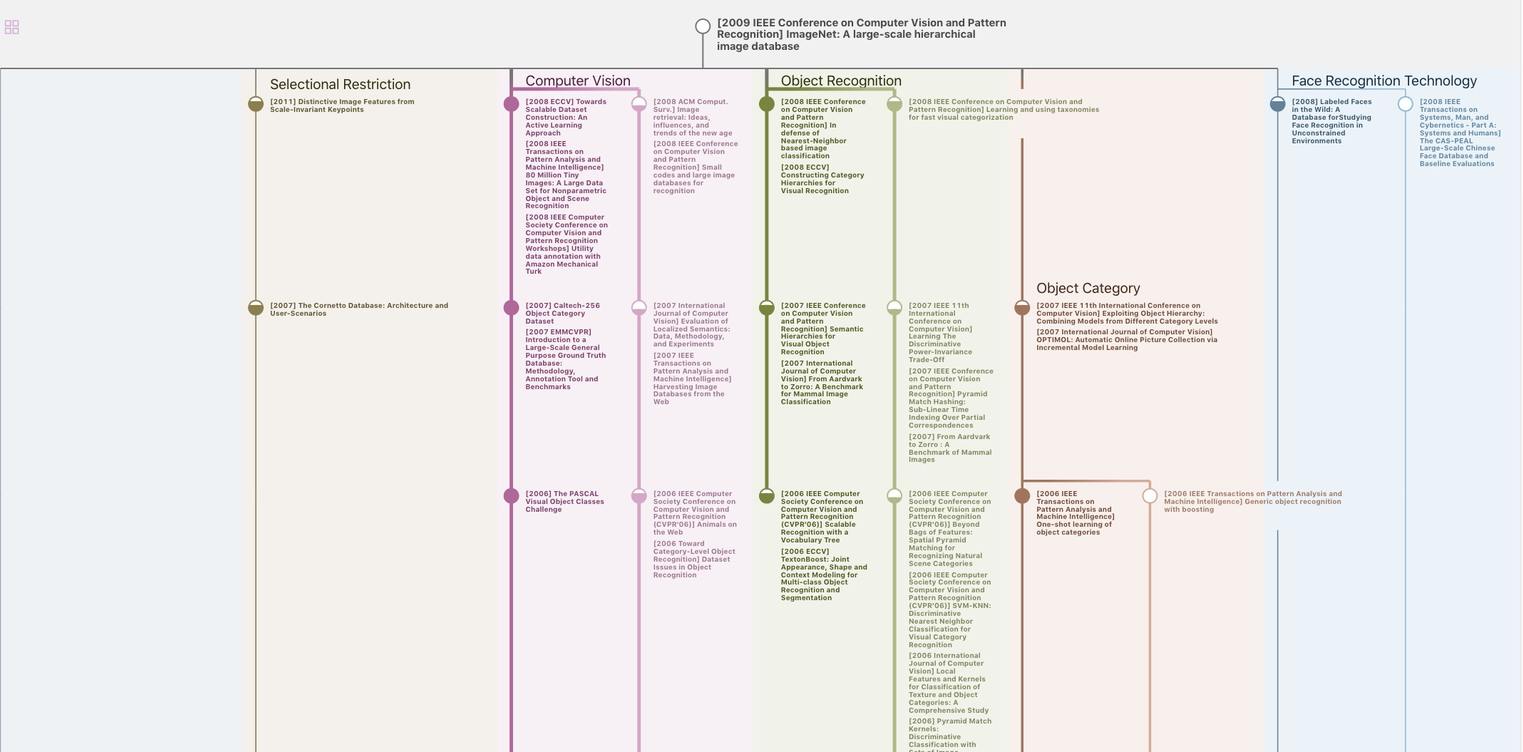
生成溯源树,研究论文发展脉络
Chat Paper
正在生成论文摘要