Hierarchical Model Compression via Shape-Edge Representation of Feature Maps-an Enlightenment From the Primate Visual System
IEEE TRANSACTIONS ON MULTIMEDIA(2023)
摘要
The cumbersome computation of deep neural networks (DNNs) limits their practical deployment on resource-constrained mobile multimedia devices. To deploy DNNs on devices with limited computing resources, model compression techniques are leveraged to accelerate the networks, where network pruning can improve the inference efficiency of DNNs by removing redundant weights and structures. As one of the important components of DNNs, the feature maps (FMs) can be leveraged to evaluate the importance of network structures for DNN pruning. However, previous methods neglect to fully explore the characteristics of FMs in network pruning. In this paper, we investigate the high capacity and resource efficient analogy-ventral dual-pathway primates visual system (PVS) to propose a hierarchical pruning framework (dubbed as HPSE). In an efficient PVS, the analog pathway analyzes low-frequency information to facilitate the high-frequency information inference in ventral stream. In HPSE, we extract the low-frequency shape information and high-frequency edge information from FMs to present a novel pruning pipeline that resembles the analysis mechanism of PVS. In particular, we first imitate the analogy pathway to group different FMs in each layer by calculating the shape-feature overlap. Secondly, we leverage the edge information modulated by the grouping results of the first step to prune the network. The effectiveness of HPSE is verified by pruning various DNNs on different benchmarks. For example, for ResNet-56 on CIFAR-10, HPSE reduces 52.9% of FLOPs with a slight accuracy improvement; for ResNet-50 on ImageNet, we achieve 54.3%-FLOPs drop with only 0.49% Top-1 accuracy loss.
更多查看译文
关键词
Frequency modulation,Shape,Image edge detection,Visualization,Visual systems,Streaming media,Feature extraction,Model compression,feature maps,shape and edge,primate visual system,deep neural network
AI 理解论文
溯源树
样例
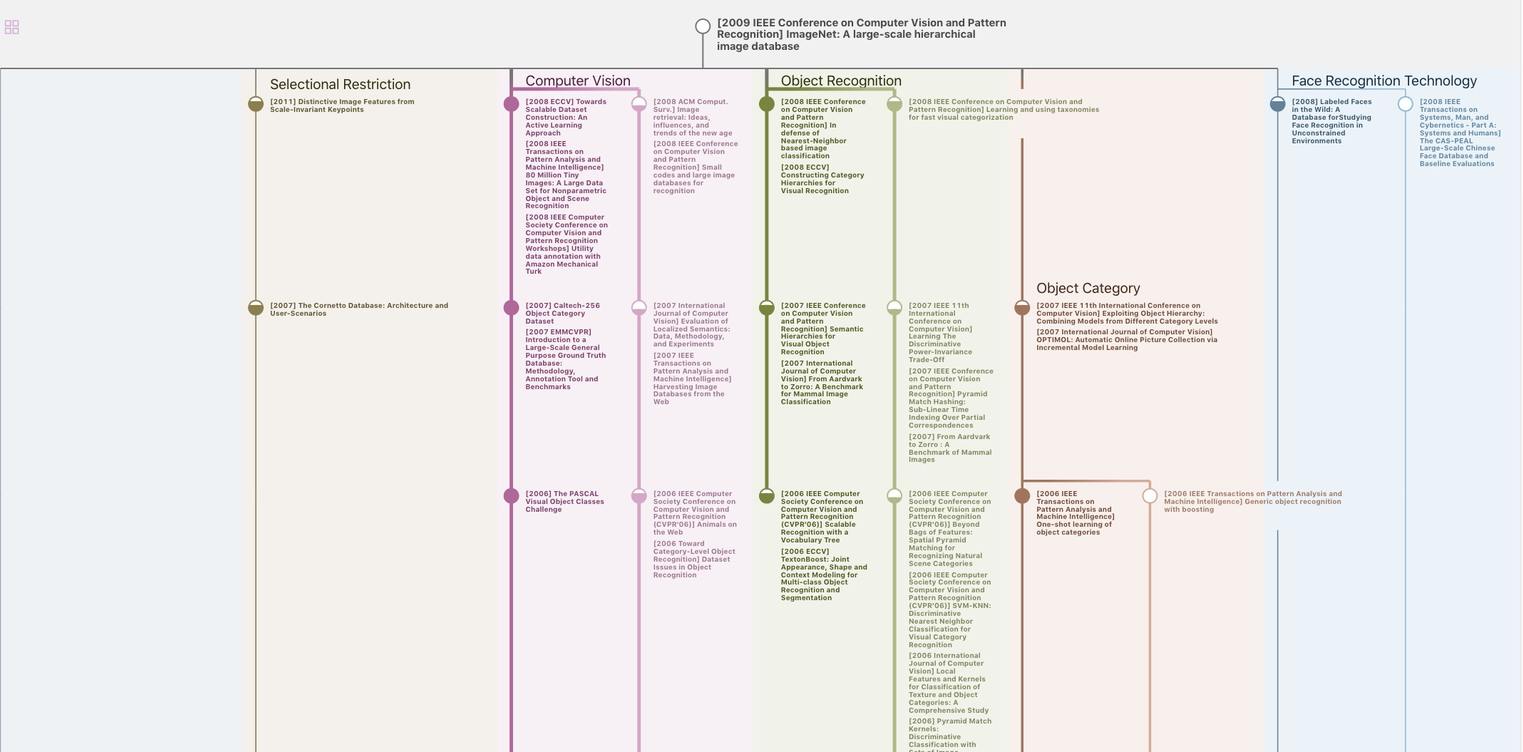
生成溯源树,研究论文发展脉络
Chat Paper
正在生成论文摘要