Non-intrusive Load Disaggregation Based on Fully Convolutional Denoising Encoder and Self-attention Mechanism
2022 4th International Conference on Power and Energy Technology (ICPET)(2022)
摘要
The non-intrusive load disaggregation (NILD) technique based on low-frequency data has a low initial investment cost, a highly promotion value, and a wide range of applications among residential customers. A non-intrusive load disaggregation model based on a fully convolution denoising encoder and self-attention mechanism (FCN-ATT) is proposed in this study. The model uses the one-dimensional aggregation power consumption as the input, the self-attention mechanism is used to improve the network's capacity to extract and apply load features, and the fully convolutional denoising encoder architecture to successfully minimize the model's number of parameters. Finally, the suggested model is tested on the publicly available UK-DALE dataset. The proposed model outperforms the three benchmark models in terms of load disaggregation and significant decreases the number of model parameters.
更多查看译文
关键词
convolutional denoising encoder,load,non-intrusive,self-attention
AI 理解论文
溯源树
样例
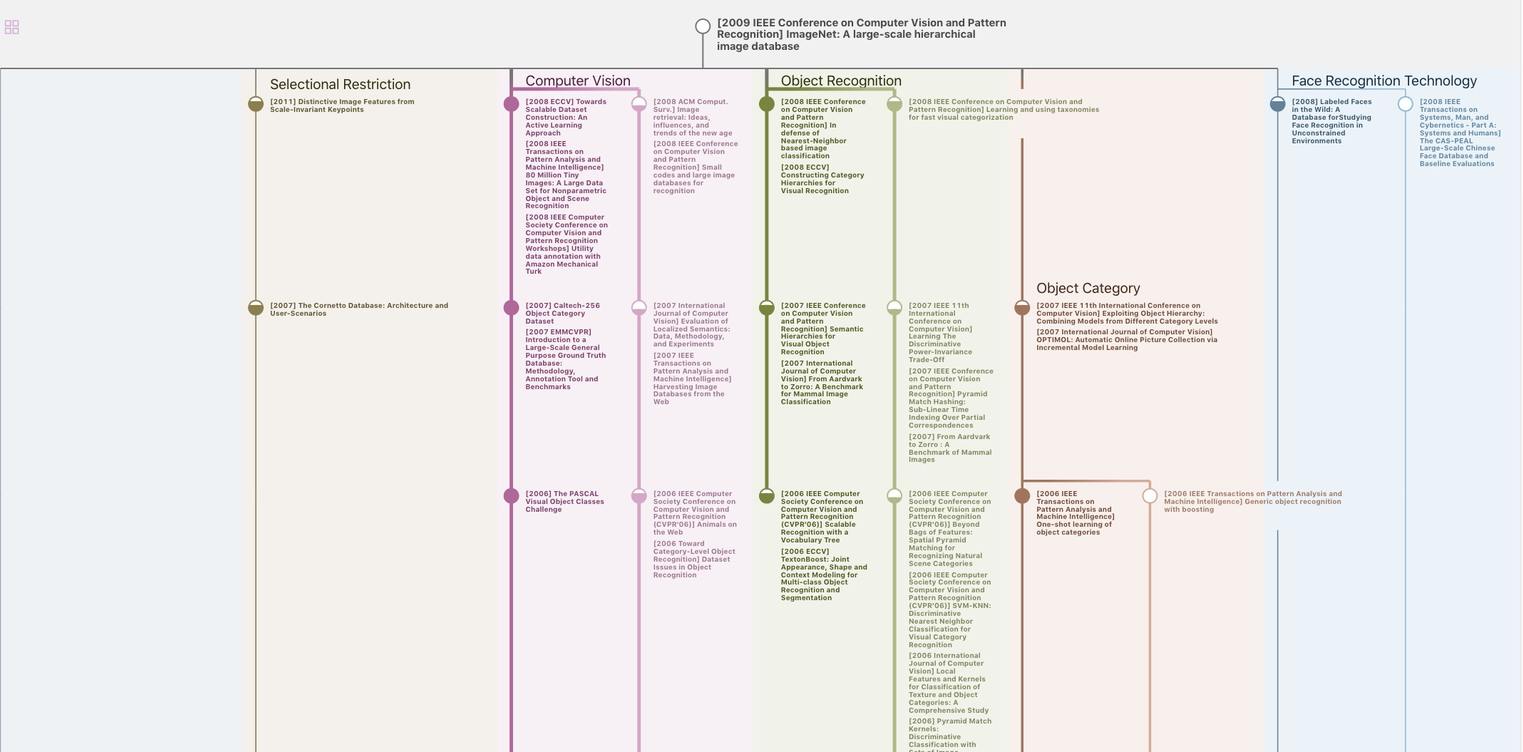
生成溯源树,研究论文发展脉络
Chat Paper
正在生成论文摘要