Time-Adaptive Expectation Maximization Learning Framework for HMM Based Data-Driven Gas Sensor Calibration
IEEE Transactions on Industrial Informatics(2023)
摘要
In this article, data-driven self-calibration algorithms for the low-cost gas sensors are designed. The sensor measurement errors happen due to the imperfect compensation for the variation of sensor component behavior that is caused by changing of environmental factors. To calibrate the sensors, the hidden Markov model (HMM) is utilized to characterize the statistical dependency between the environmental factors and the variation of sensor component behavior. Considering the time-varying property of this dependency, a time-adaptive learning framework is further designed to update the HMM so that the time-varying drift process can be better tracked over a long term. More specifically, a time-adaptive expectation maximization learning approach is proposed to efficiently update the HMM parameters. A closed form of the convergence rate of this time-adaptive learning approach is derived, which provides a theoretical guarantee on the time efficiency as well as the computational efficiency. The performance of the scheme is illustrated in numerical experiments utilizing real data, which shows that long-term stable calibration performance can be achieved.
更多查看译文
关键词
Data-driven calibration,expectation maximization (EM),hidden Markov model (HMM),nondispersive infrared (NDIR) gas sensor
AI 理解论文
溯源树
样例
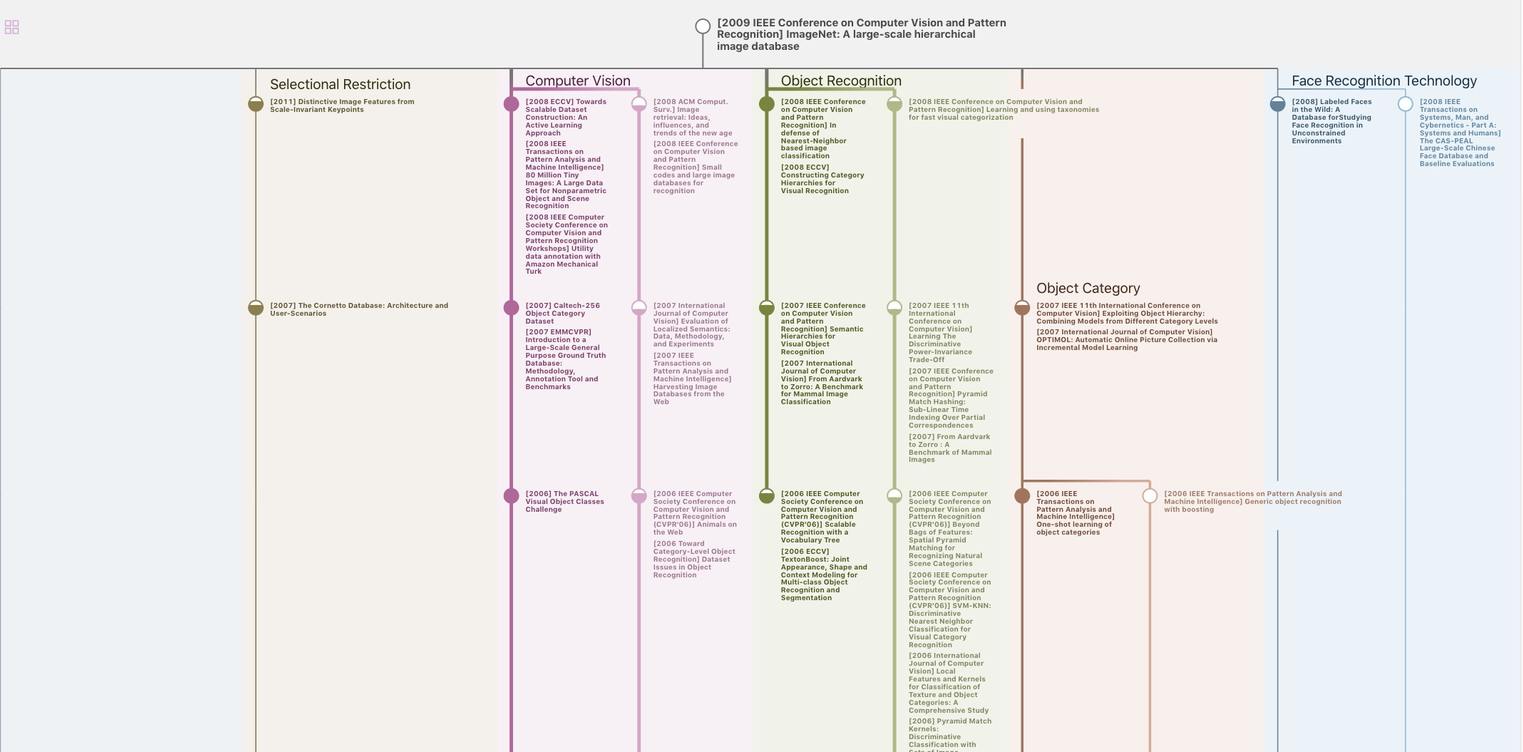
生成溯源树,研究论文发展脉络
Chat Paper
正在生成论文摘要