OERL: Enhanced Representation Learning via Open Knowledge Graphs
IEEE Transactions on Knowledge and Data Engineering(2023)
摘要
The sparseness and incompleteness of knowledge graphs (KGs) trigger considerable interest in enhancing the representation learning with external corpora. However, the difficulty of aligning entities and relations with external corpora leads to inferior performance improvement. Open knowledge graphs (OKGs) consist of entity-mentions and relation-mentions that are represented by noncanonicalized freeform phrases, which generally do not rely on the specification of ontology schema. The roughness of the nonontological construction method leads to a specific characteristic of OKGs: diversity, where multiple entity-mentions (or relation-mentions) have the same meaning but different expressions. The diversity of OKGs can provide potential textual and structural features for the representation learning of KGs. We speculate that leveraging OKGs to enhance the representation learning of KGs can be more effective than using pure text or pure structure corpora. In this paper, we propose a new
OERL
,
O
pen knowledge graph
E
nhanced
R
epresentation
L
earning of KGs. OERL automatically extracts textual and structural connections between KGs and OKGs, models and transfers refined profitable features to enhance the representation learning of KGs. The strong performance improvement and exhaustive experimental analysis prove the superiority of OERL over state-of-the-art baselines.
更多查看译文
关键词
enhanced representation learning,oerl,knowledge
AI 理解论文
溯源树
样例
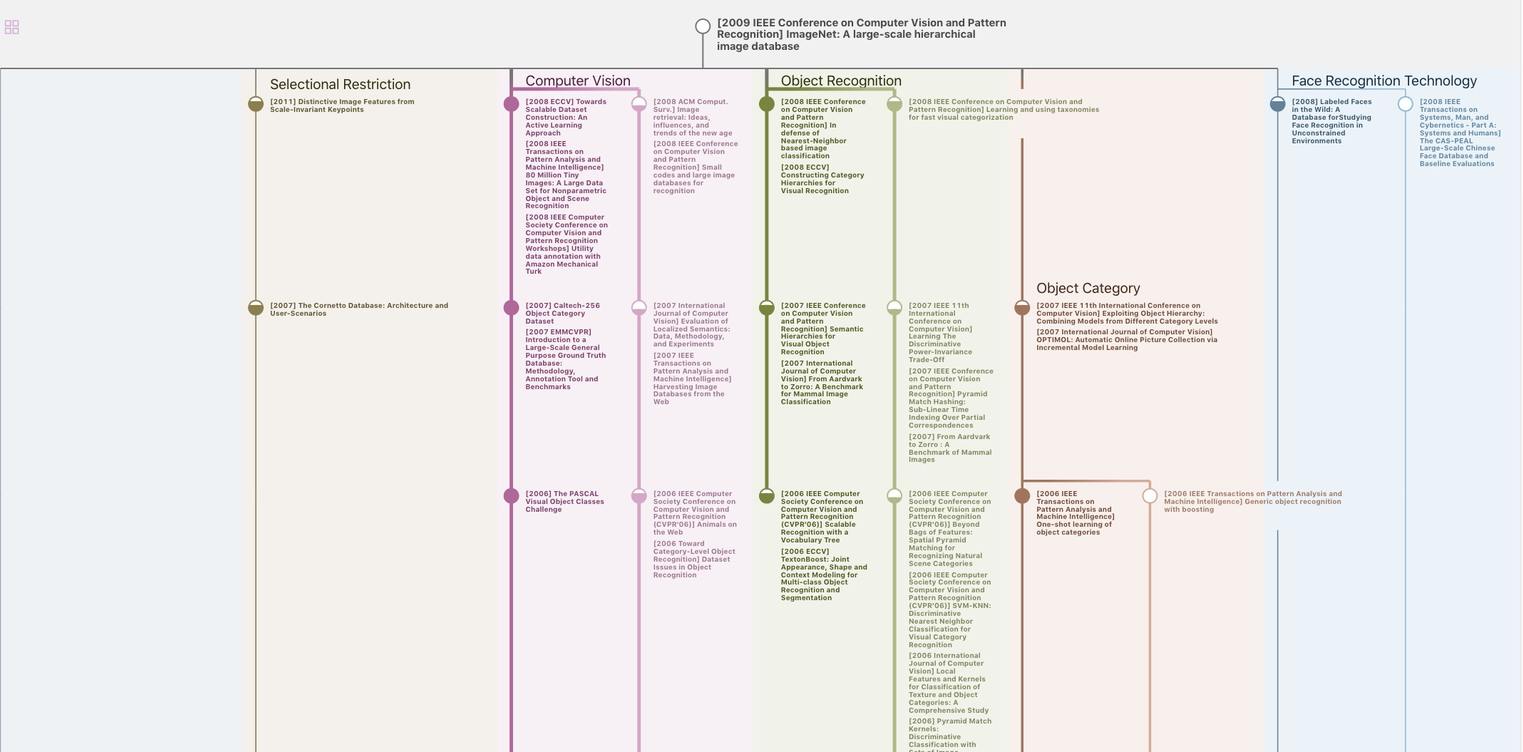
生成溯源树,研究论文发展脉络
Chat Paper
正在生成论文摘要