Learning from coarsely-labeled images for semantic segmentation
2022 37th Youth Academic Annual Conference of Chinese Association of Automation (YAC)(2022)
摘要
Image segmentation aims to assign a semantic label to each pixel of an image and thus requires accurate pixel-wise annotations of massive images to train high-performance models. This paper aims to alleviate the arduous annotations by learning from coarser polygonal annotations that can be acquired at a lower cost. A novel self-training framework is proposed to build a robust semantic segmentation model in the presence of noisy labels. A superpixel-based relabeling strategy is developed to refine the original annotations according to the local context. Then multiple segmentation models are trained on the revised annotations and produce multiple pseudo labels for model re-training. Finally, a new segmentation model is re-trained by fusing multiple pseudo-labels in terms of their confidences. Experimental results on the Cityscapes dataset demonstrate the effectiveness of the proposed method.
更多查看译文
关键词
Image segmentation,coarse annotations,self-training,superpixel-based relabeling strategy
AI 理解论文
溯源树
样例
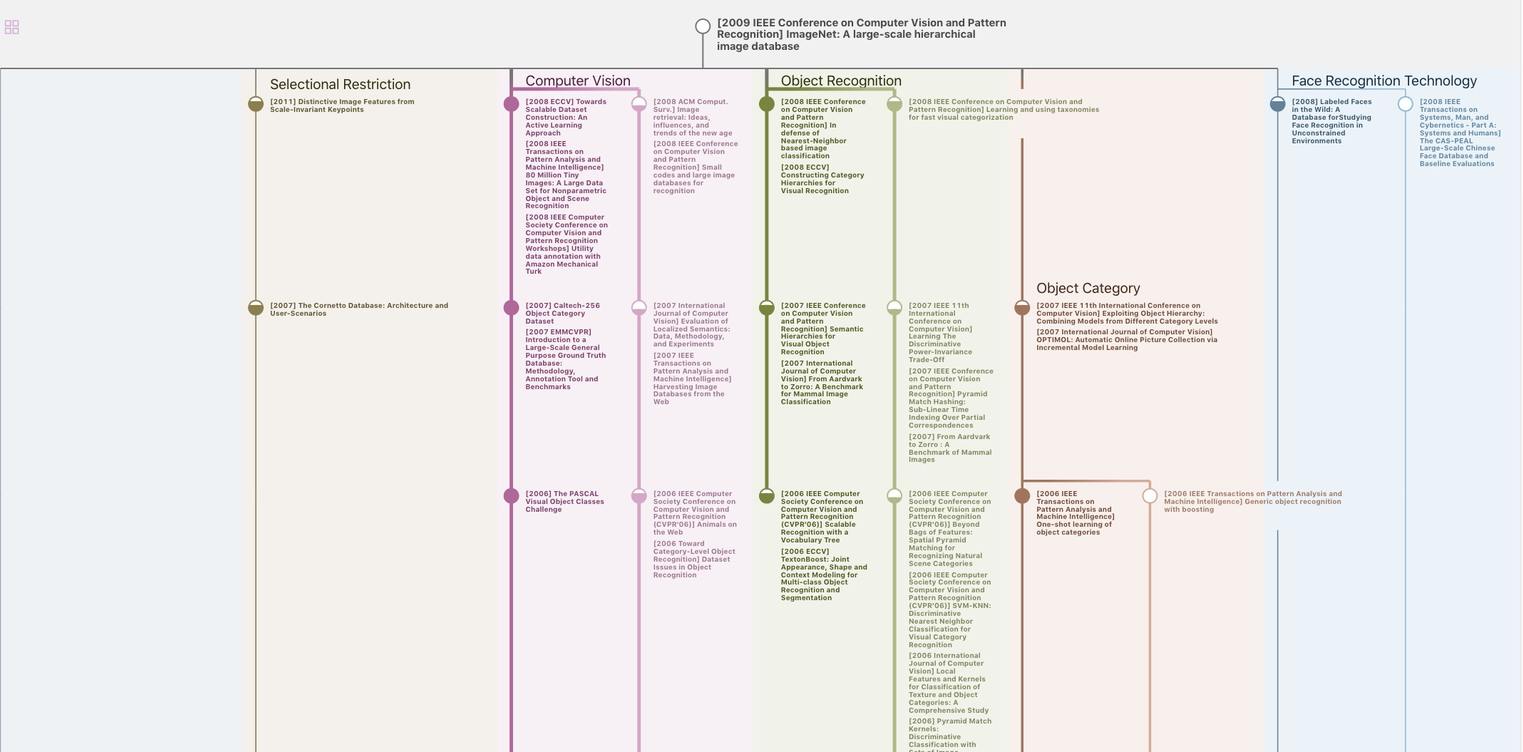
生成溯源树,研究论文发展脉络
Chat Paper
正在生成论文摘要