The Evolution of Image Denoising From Model-Driven to Machine Learning
Advances in Computational Intelligence and RoboticsHandbook of Research on Computer Vision and Image Processing in the Deep Learning Era(2022)
摘要
Image denoising is a class of image processing algorithms that aim to enhance the visual quality of the acquired images by removing noise inherent in them and is an active area of research under image enhancement and reconstruction techniques. Traditional model-driven methods are motivated by statistical assumptions on data corruption and prior knowledge of the data to recover while the machine learning (ML) approaches require a massive amount of training data. However, the manual tuning of hyperparameters in model-driven approaches and susceptibility to overfitting under learning-based techniques are their major flaws. Recent years have witnessed the amalgamation of both model and ML-based approaches. Infusing model-driven Bayesian estimator in an ML-based approach, supported by robust mathematical arguments, has been shown to achieve optimal denoising solutions in real time with less effect of over-fitting. In this chapter, the evolution of image denoising techniques is covered from a mathematical perspective along with detailed experimental analysis for each class of approach.
更多查看译文
关键词
image denoising,machine learning,model-driven
AI 理解论文
溯源树
样例
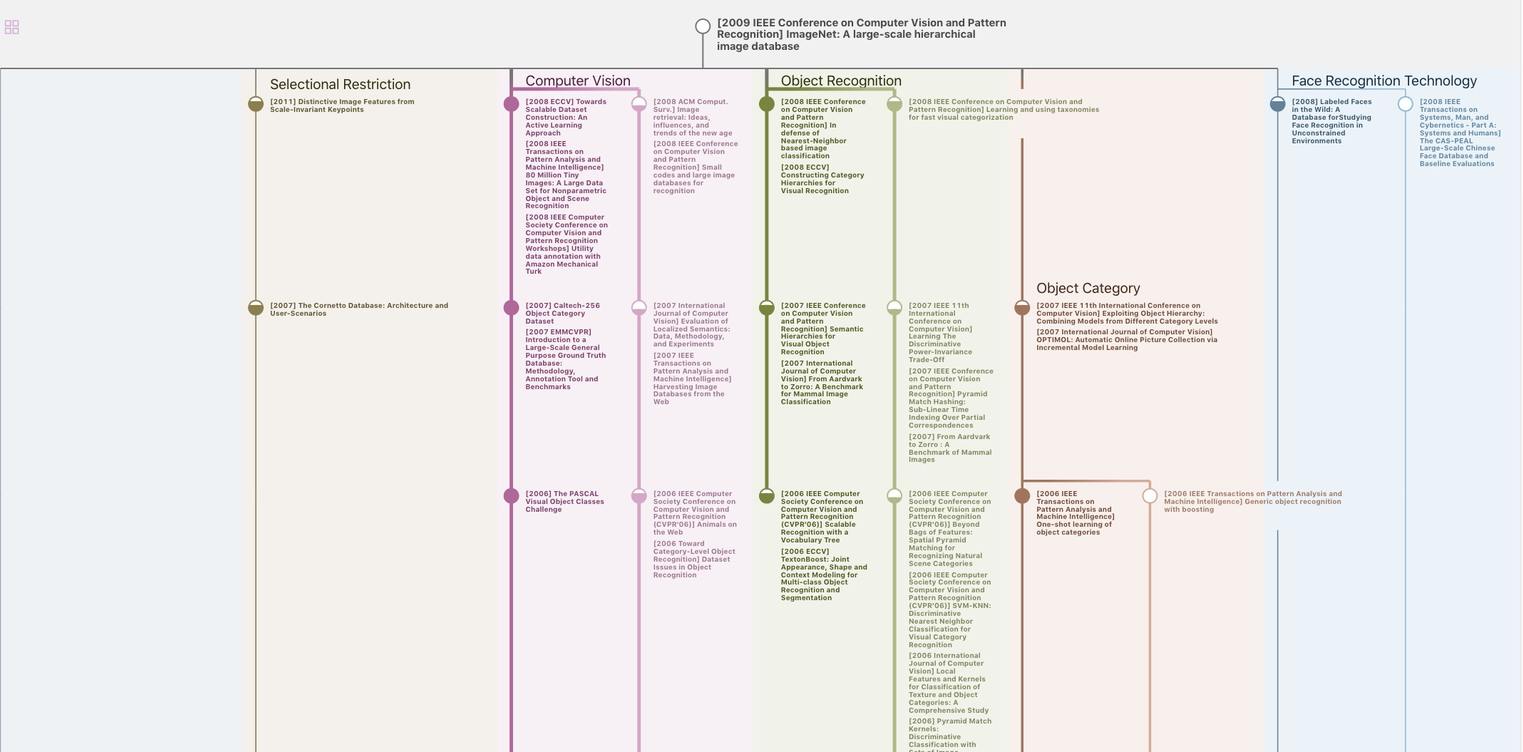
生成溯源树,研究论文发展脉络
Chat Paper
正在生成论文摘要