WRIST: Wideband, Real-Time, Spectro-Temporal RF Identification System Using Deep Learning
IEEE TRANSACTIONS ON MOBILE COMPUTING(2024)
摘要
RF emissions' detection, classification, and spectro-temporal localization are essential not only for understanding, managing, and protecting the radio frequency resources, but also for countering today's security threats such as jammers. Achieving this goal for wideband, real-time operation remains challenging. In this article, we present WRIST, a Wideband, Real-time, Spectro-Temporal RF Identification system. WRIST can detect, classify, and precisely locate RF emissions in time and frequency using RF samples of 100 MHz spectrum in real-time. The system leverages an one-stage object detection Deep Learning framework, and transfer learning to a multi-channel visual-based spectral representation. Towards developing WRIST, we devised an iterative training approach which leverages synthesized and augmented RF data to efficiently build a large dataset with high-quality labels. WRIST achieves over 99% class detection accuracy, 94% emission precision and recall, with less than 0.08 bandwidth and time offset ratios in a large anechoic chamber over-the-air environment. In the extremely congested in-the-wild environment, WRIST still achieves over 80% precision and recall. WRIST currently supports five 2.4 GHz technologies (Bluetooth, Lightbridge, Wi-Fi, XPD, and ZigBee) and is easily extendable to others. We are making our curated dataset available to the whole community. It comprises over 10 million labelled RF emissions from off-the-shelf wireless radios spanning the five classes of technologies.
更多查看译文
关键词
Radio frequency,Real-time systems,Wideband,Wireless communication,Deep learning,Time-frequency analysis,Wireless fidelity,Dataset,deep learning,spectro-temporal RF identification,wideband and real-time system
AI 理解论文
溯源树
样例
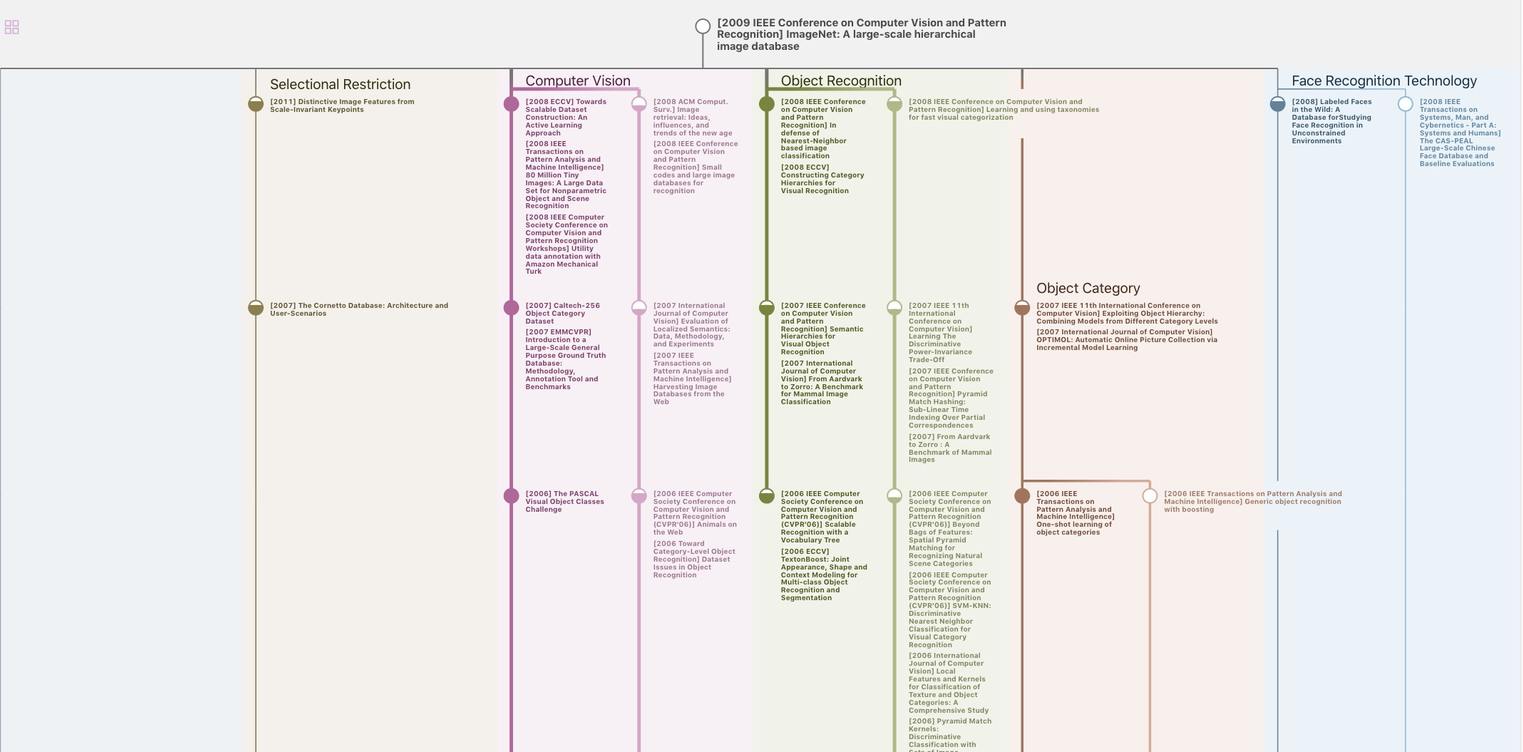
生成溯源树,研究论文发展脉络
Chat Paper
正在生成论文摘要