Fusing Higher and Lower-Order Biological Information for Drug Repositioning via Graph Representation Learning.
IEEE Trans. Emerg. Top. Comput.(2024)
摘要
Drug repositioning is a promising drug development technique to identify new indications for existing drugs. However, existing computational models only make use of lower-order biological information at the level of individual drugs, diseases and their associations, but few of them can take into account higher-order connectivity patterns presented in biological heterogeneous information networks (HINs). In this work, we propose a novel graph representation learning model, namely FuHLDR, for drug repositioning by fusing higher and lower-order biological information. Specifically, given a HIN, FuHLDR first learns the representations of drugs and diseases at a lower-order level by considering their biological attributes and drug-disease associations (DDAs) through a graph convolutional network model. Then, a meta-path-based strategy is designed to obtain their higher-order representations involving the associations among drugs, proteins and diseases. Their integrated representations are thus determined by fusing higher and lower-order representations, and finally a Random Vector Functional Link Network is employed by FuHLDR to identify novel DDAs. Experimental results on two benchmark datasets demonstrate that FuHLDR performs better than several state-of-the-art drug repositioning models. Furthermore, our case studies on Alzheimer's disease and Breast neoplasms indicate that the rich higher-order biological information gains new insight into drug repositioning with improved accuracy.
更多查看译文
关键词
drug repositioning,drug-disease association,higher and lower-order information,information fusion,graph representation learning
AI 理解论文
溯源树
样例
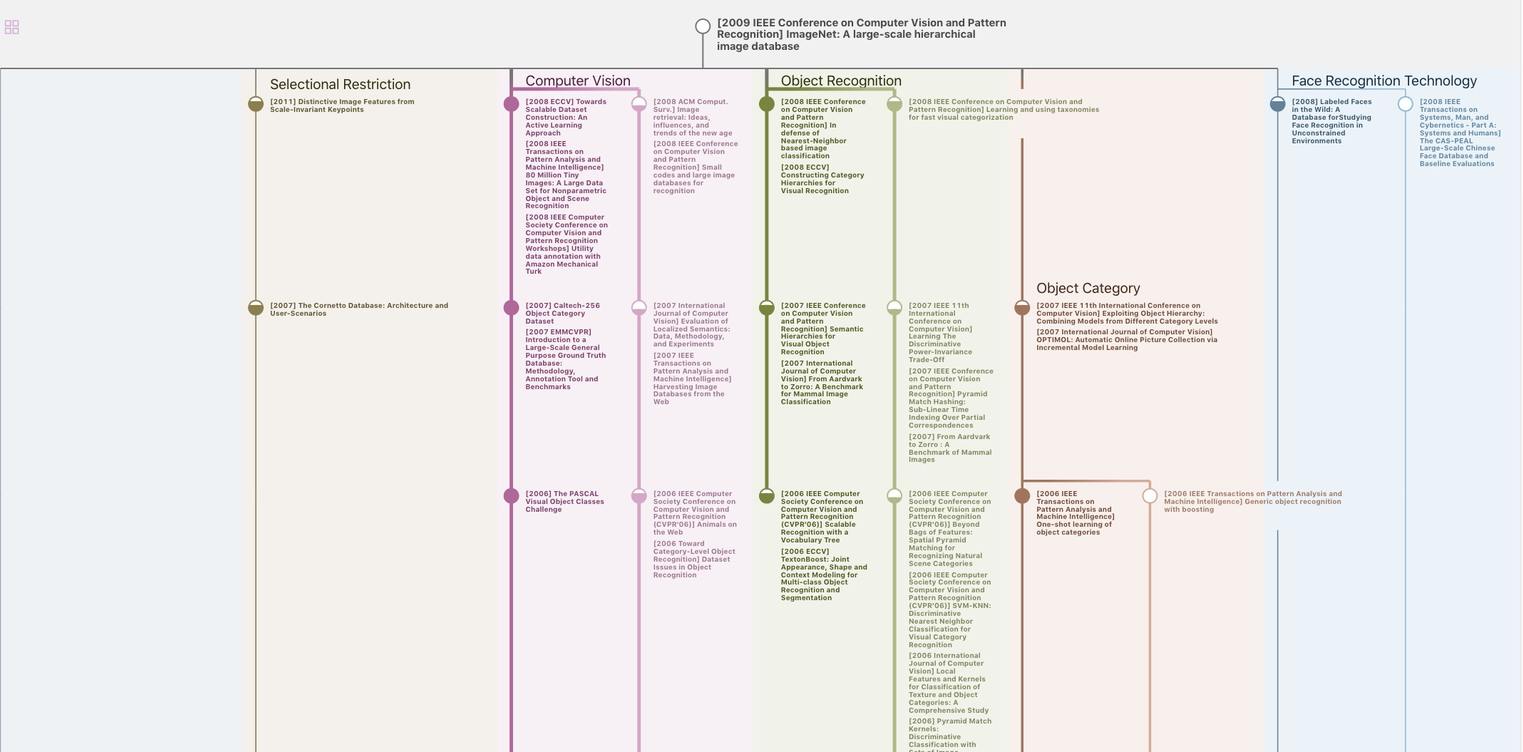
生成溯源树,研究论文发展脉络
Chat Paper
正在生成论文摘要