Attention Spiking Neural Networks.
IEEE Transactions on Pattern Analysis and Machine Intelligence(2023)
摘要
Brain-inspired spiking neural networks (SNNs) are becoming a promising energy-efficient alternative to traditional artificial neural networks (ANNs). However, the performance gap between SNNs and ANNs has been a significant hindrance to deploying SNNs ubiquitously. To leverage the full potential of SNNs, in this paper we study the attention mechanisms, which can help human focus on important information. We present our idea of attention in SNNs with a multi-dimensional attention module, which infers attention weights along the temporal, channel, as well as spatial dimension separately or simultaneously. Based on the existing neuroscience theories, we exploit the attention weights to optimize membrane potentials, which in turn regulate the spiking response. Extensive experimental results on event-based action recognition and image classification datasets demonstrate that attention facilitates vanilla SNNs to achieve sparser spiking firing, better performance, and energy efficiency concurrently. In particular, we achieve top-1 accuracy of 75.92% and 77.08% on ImageNet-1 K with single/4-step Res-SNN-104, which are state-of-the-art results in SNNs. Comparedwith counterpart Res-ANN-104, the performance gap becomes -0.95/+0.21 percent and the energy efficiency is 31.8x/7.4x. To analyze the effectiveness of attention SNNs, we theoretically prove that the spiking degradation or the gradient vanishing, which usually holds in general SNNs, can be resolved by introducing the block dynamical isometry theory. We also analyze the efficiency of attention SNNs based on our proposed spiking response visualization method. Our work lights up SNN's potential as a general backbone to support various applications in the field of SNNresearch, with a great balance between effectiveness and energy efficiency.
更多查看译文
关键词
neural networks,attention
AI 理解论文
溯源树
样例
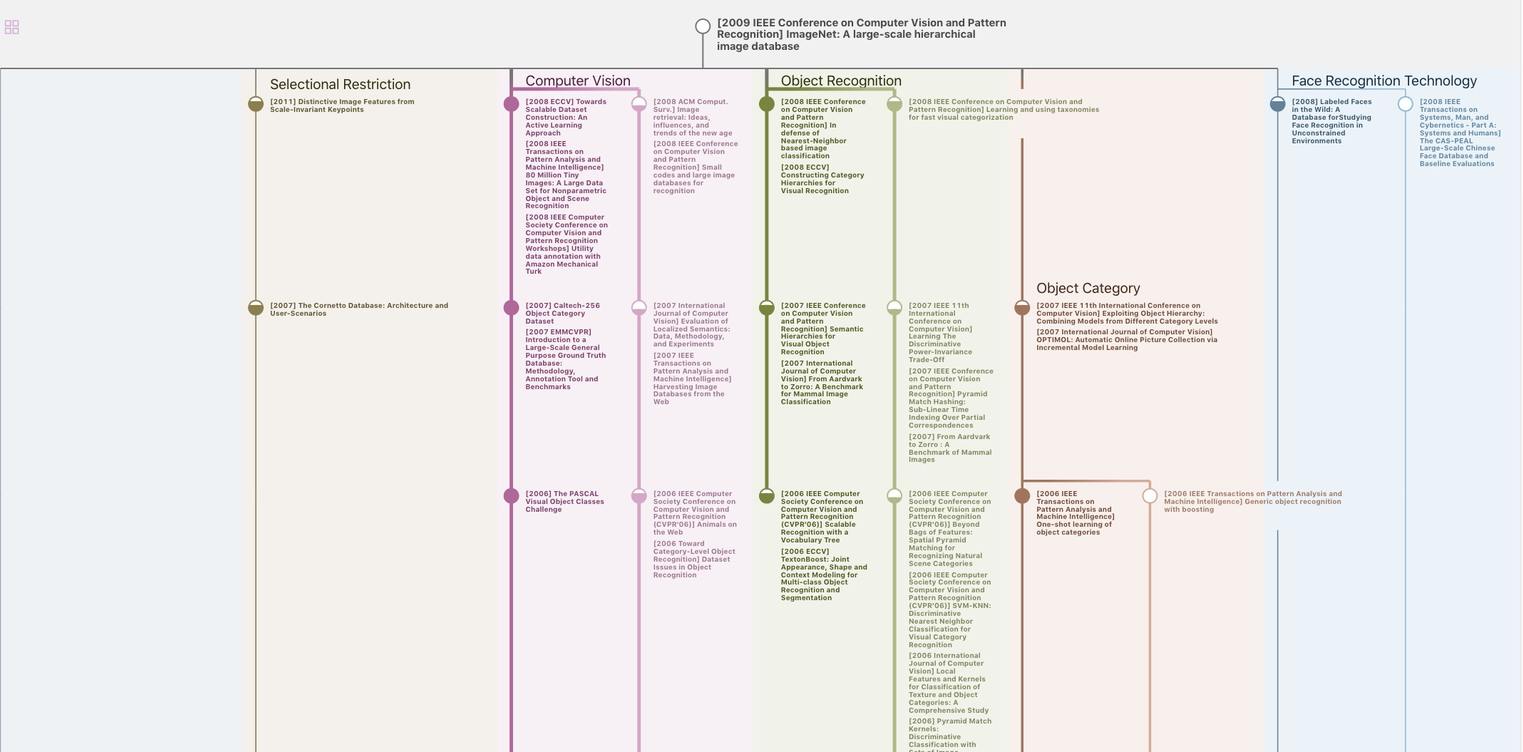
生成溯源树,研究论文发展脉络
Chat Paper
正在生成论文摘要