KC-GEE: knowledge-based conditioning for generative event extraction
WORLD WIDE WEB-INTERNET AND WEB INFORMATION SYSTEMS(2023)
摘要
Event extraction is an important, but challenging task. Many existing techniques decompose it into event and argument detection/classification subtasks, which are complex structured prediction problems. Generation-based extraction techniques lessen the complexity of the problem formulation and are able to leverage the reasoning capabilities of large pretrained language models. However, they still suffer from poor zero-shot generalizability and are ineffective in handling long contexts such as documents. We propose a generative event extraction model, KC-GEE, that addresses these limitations. A key contribution of KC-GEE is a novel knowledge-based conditioning technique that injects the schema of candidate event types as the prefix into each layer of an encoder-decoder language model. This enables effective zero-shot learning and improves supervised learning. Our experiments on two benchmark datasets demonstrate the strong performance of our KC-GEE model. It achieves particularly strong results in the challenging document-level extraction task and in the zero-shot learning setting, outperforming state-of-the-art models by up to 5.4 absolute F1 points.
更多查看译文
关键词
Event extraction,Information extraction,Zero-shot learning,Document-level event extraction
AI 理解论文
溯源树
样例
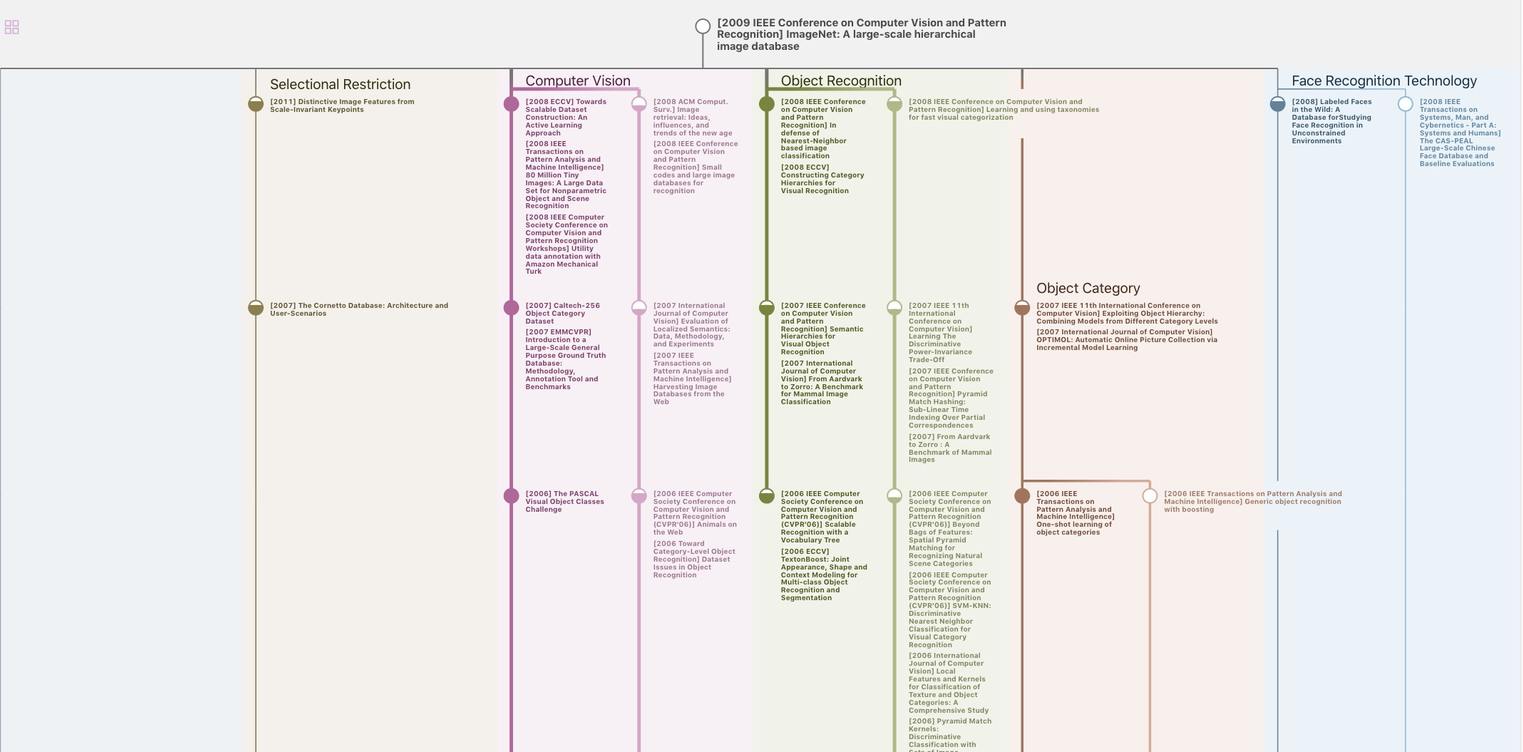
生成溯源树,研究论文发展脉络
Chat Paper
正在生成论文摘要