The Devil is in Details: Delving Into Lite FFN Design for Vision Transformers
ICASSP 2024 - 2024 IEEE International Conference on Acoustics, Speech and Signal Processing (ICASSP)(2024)
摘要
Transformer has demonstrated exceptional performance on a variety of vision tasks. However, its high computational complexity can become problematic. In this paper, we conduct a systematic analysis of the complexity of each component in vision transformers, and identify an easily overlooked detail: that the Feed-Forward Network (FFN) is the primary computational bottleneck, even more so than the Multi-Head Self-Attention (MHSA) mechanism. Inspired by this, we further propose a lightweight FFN module, named SparseFFN, that can reduce dense computations in both channel and spatial dimension. Specifically, SparseFFN consists of two components: Channel-Sparse FFN (CS-FFN) and Spatial-Sparse FFN (SS-FFN), which can be seamlessly incorporated into various vision transformers and even pure MLP models with significantly fewer FLOPs. Extensive experiments demonstrate the effectiveness and efficiency of the proposed method. For example, our approach can reduce model complexity by 23%-39% for most of vision transformers and MLP models while keeping comparable accuracy.
更多查看译文
关键词
Vision Transformer,Light-Weight Structure,Feed-Forward Networks
AI 理解论文
溯源树
样例
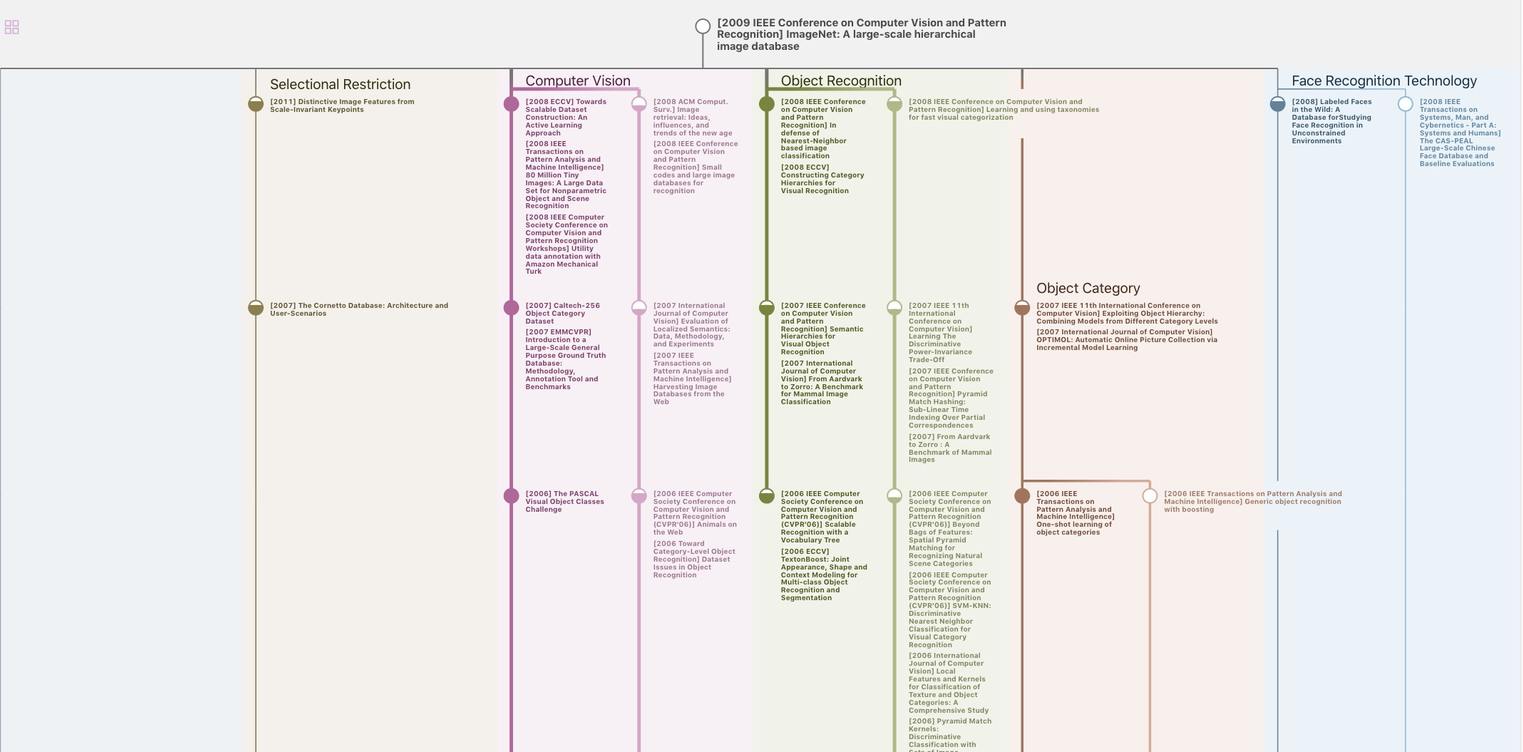
生成溯源树,研究论文发展脉络
Chat Paper
正在生成论文摘要